Better Data Distillation by Condensing the Interpolated Graphs.
International Conference on Advanced Cloud and Big Data(2023)
摘要
Tasks like continual learning and neural architecture search in deep learning often require neural model retraining. Retraining large neural models iteratively consumes a huge amount of time and resources. Recent research efforts on dataset distillation propose to condense the implicit information from large training datasets into small synthetic graphs, resulting in speeding up the neural model training. However, existing methods lose critique knowledge from datasets during the condensation process, which leads to poor performance in terms of generalization and robustness of the synthetic datasets. In this paper, we focus on graph data to address the above issues. Our proposed framework employs graph augmentation methods to generate interpolated graph data based on the training dataset, which enriches the implicit knowledge during the distillation process. The generated interpolated graph data, together with the original training data, help gradient-based data distillation methods to obtain better synthetic datasets. Experimental results show that data interpolation augmentation methods can improve the quality of the synthetic datasets, demonstrating that the proposed framework performs better than other state-of-the-art distillation methods. The source codes are available at https://github.com/t-kanade/GDDA.
更多查看译文
关键词
Graph Neural Networks,Dataset Distillation,Graph Dataset Augmentation,Data Interpolation
AI 理解论文
溯源树
样例
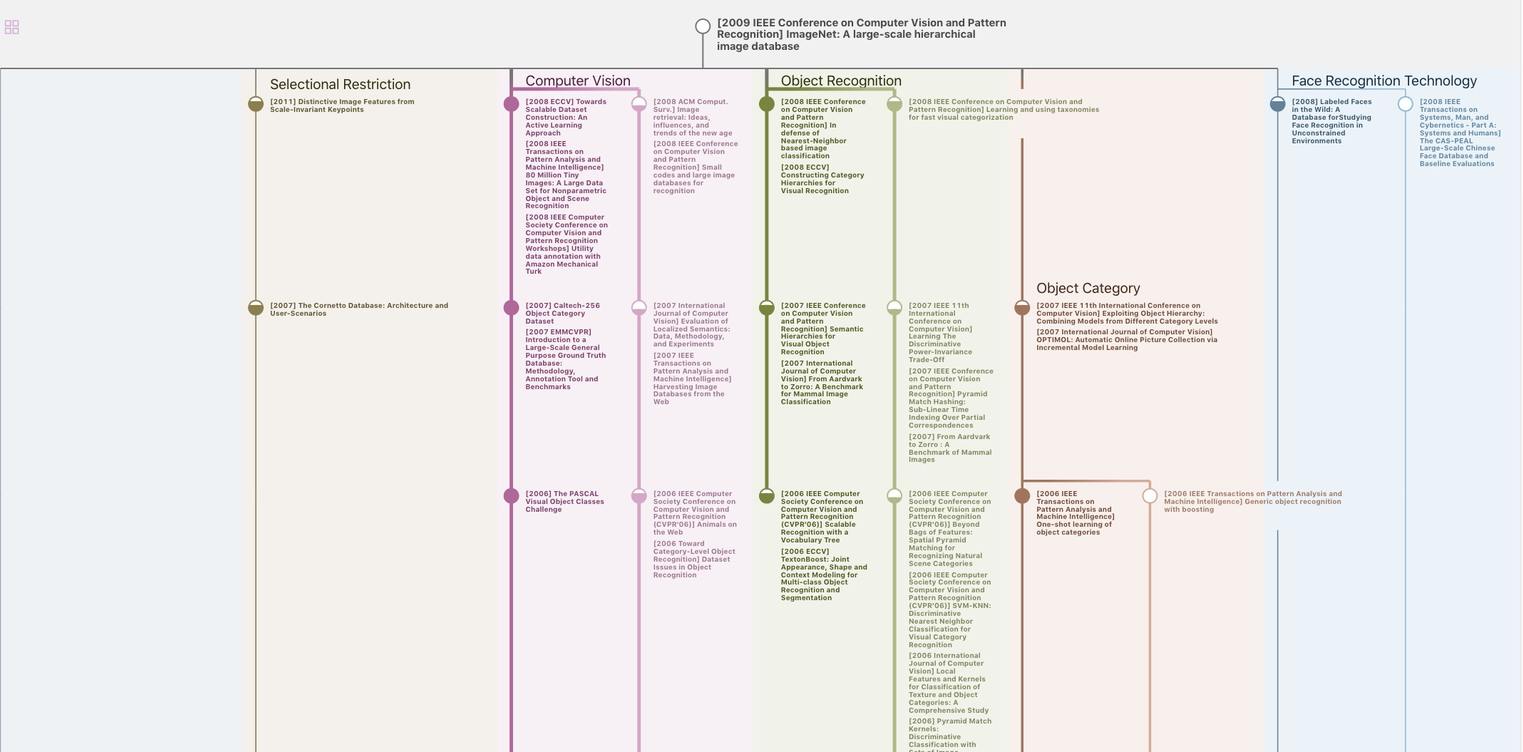
生成溯源树,研究论文发展脉络
Chat Paper
正在生成论文摘要