A learning numerical spiking neural P system for classification problems
Knowledge-Based Systems(2024)
摘要
In recent years, classification problems have been widely studied as one of the critical research directions in artificial intelligence. Efficiently simulating the human brain’s ability to solve deterministic classification problems has become a prominent research branch in the field of classification problems. Numerical spiking neural P systems (NSN P systems) with the characteristics of the highly flexible spiking neural P (SNP)systems architecture and addressing numerical variants have special advantages for solving classification problems in the real world. This paper proposes, a novel NSN P system, namely, a learning numerical spiking neural P system (LNSN P system), is proposed to solve classification problems. In LNSN P systems, each neuron contains not only discrete spikes and rules that must be described by regular expressions but also numerical variables and production functions that can be in any form, overcoming the discontinuity of traditional SN P systems. In addition, an ascending structure without an outer encoder has been designed in this study to encode information from low-dimensional indivisible space to high-dimensional divisible space. At the same time, an adaptive learning mechanism has been introduced to improve algorithm convergence and precision. Finally, the experimental results obtained using eight benchmark datasets from the machine learning repository demonstrate the feasibility and effectiveness of the proposed LNSN P system. Moreover, the proposed LNSN P system exhibits lower space complexity and better generalisation ability than the existing SN P systems with the learning ability to solve different classification problems.
更多查看译文
关键词
Classification problems,Spiking neural P systems,Numerical spiking neural P systems with weights,Learning numerical spiking neural P systems,Membrane computing
AI 理解论文
溯源树
样例
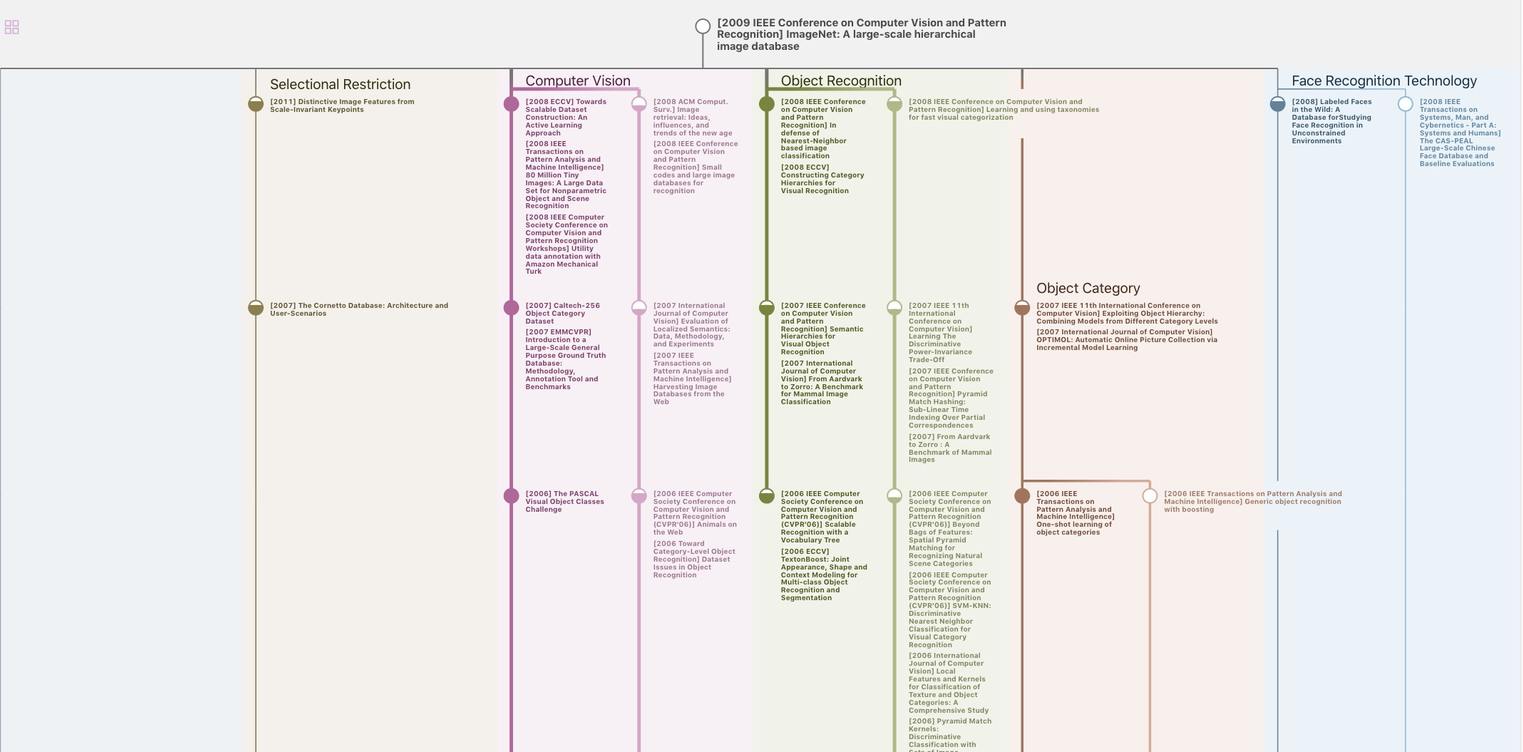
生成溯源树,研究论文发展脉络
Chat Paper
正在生成论文摘要