Empowering Women's Health: Machine Learning for PCOS Detection and Prediction
2024 International Conference on Distributed Computing and Optimization Techniques (ICDCOT)(2024)
摘要
This research delves into the prompt identification and prediction of Polycystic Ovary Syndrome utilizing machine learning, specifically focusing on the XGBoost algorithm. Through an examination of data gathered from 541 women, we pinpointed seven crucial clinical and metabolic markers from a pool of 44 features. Notably, the XGBoost algorithm showcased remarkable efficacy, achieving a testing accuracy rate of 96%, surpassing alternative methodologies. This underscores the potential of machine learning in enhancing PCOS diagnosis, facilitating prompt interventions to mitigate associated complications. By bolstering early detection capabilities, our methodology significantly contributes to enhancing patient outcomes and underscores the significance of data-driven strategies in managing PCOS. However, further validation and refinement are imperative to optimize its clinical applicability and broader influence on healthcare practices.
更多查看译文
关键词
PCOS,Machine Learning,Classification,SVM,KNN,XGBoost,Logistic Regression,Random Forest
AI 理解论文
溯源树
样例
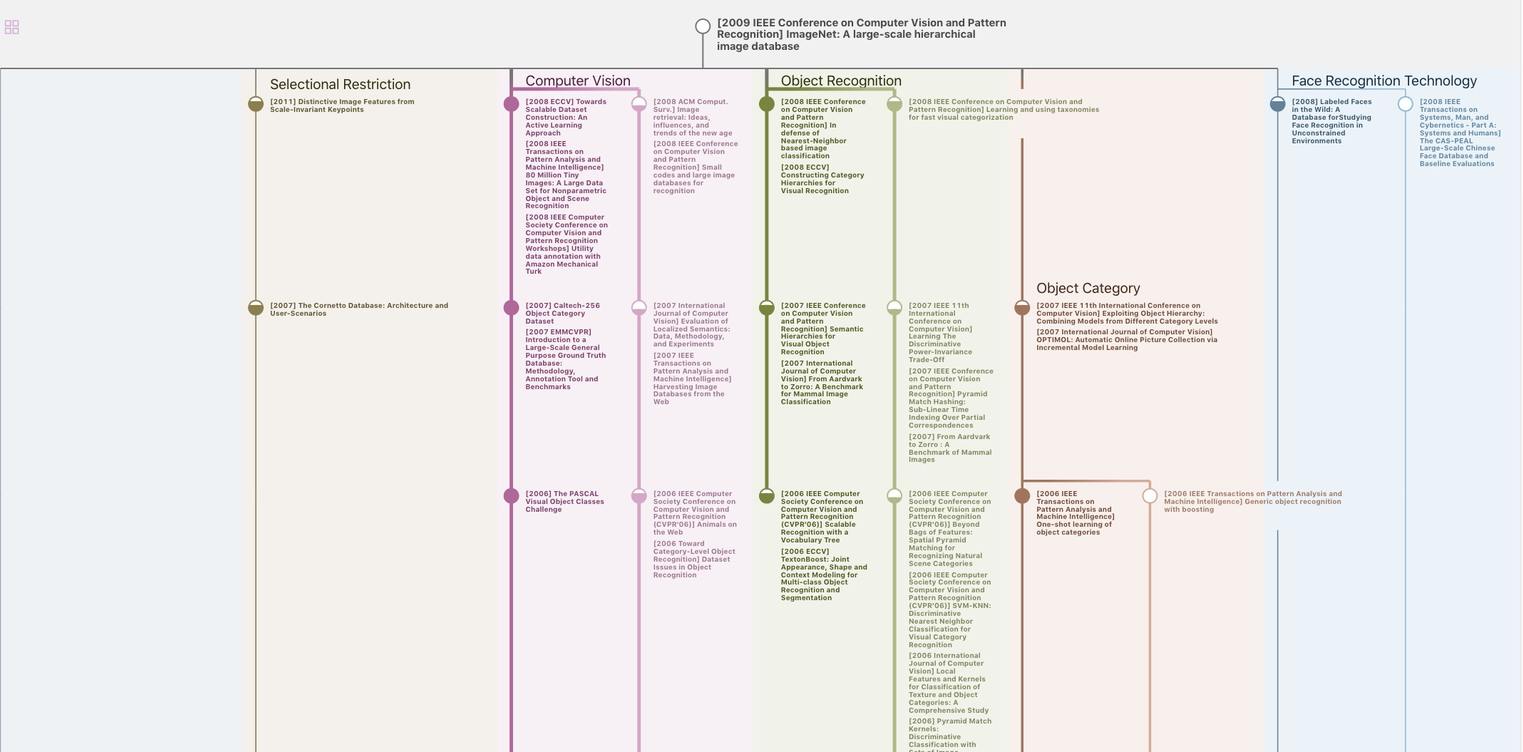
生成溯源树,研究论文发展脉络
Chat Paper
正在生成论文摘要