Efficient Multi-view Stereo by Dynamic Cost Volume and Cross-scale Propagation
IEEE Transactions on Circuits and Systems for Video Technology(2024)
摘要
Currently, learning-based multi-view stereo (MVS) has been dominated by the pipeline of 3D cost volume and regularization network over the
static cost volume
for depth regression. However, this methodology is plagued by heavy time and memory consumption, which greatly hinders the applications of these methods for real-world high-resolution images. To address these challenges, we present Effi-MVS+, an efficient multi-scale
dynamic cost volume
based MVS method. Firstly, instead of constructing a static cost volume and predicting a probability distribution map for depth regression, we update the depth map by iteratively predicting depth residuals. In each iteration, we construct a lightweight dynamic cost volume by encoding local matching and regularization information. The dynamic cost volume is subsequently processed using a 2D convolution-based GRU, which owns significant advantages in computational complexity and efficiency. Secondly, we propose a cross-scale propagation mechanism to enhance the multi-scale dynamic cost volume. This mechanism facilitates the progressive aggregation of multi-scale information, thereby providing enhanced matching and regularization information. Thirdly, to further improve the efficiency, we provide a reliable initial depth map to launch the framework and guarantee fast convergence. Extensive experiments on the DTU and Tanks & Temples benchmarks demonstrate the superiority of our method, which outperforms other state-of-the-art methods by a large margin in terms of
reconstruction quality, speed, and memory usage
. Code will be released at https://github.com/npucvr/Effi-MVS-plus.
更多查看译文
关键词
Multi-view stereo,Dynamic Cost volume,Deep Neural Networks,Cross-scale Propagation
AI 理解论文
溯源树
样例
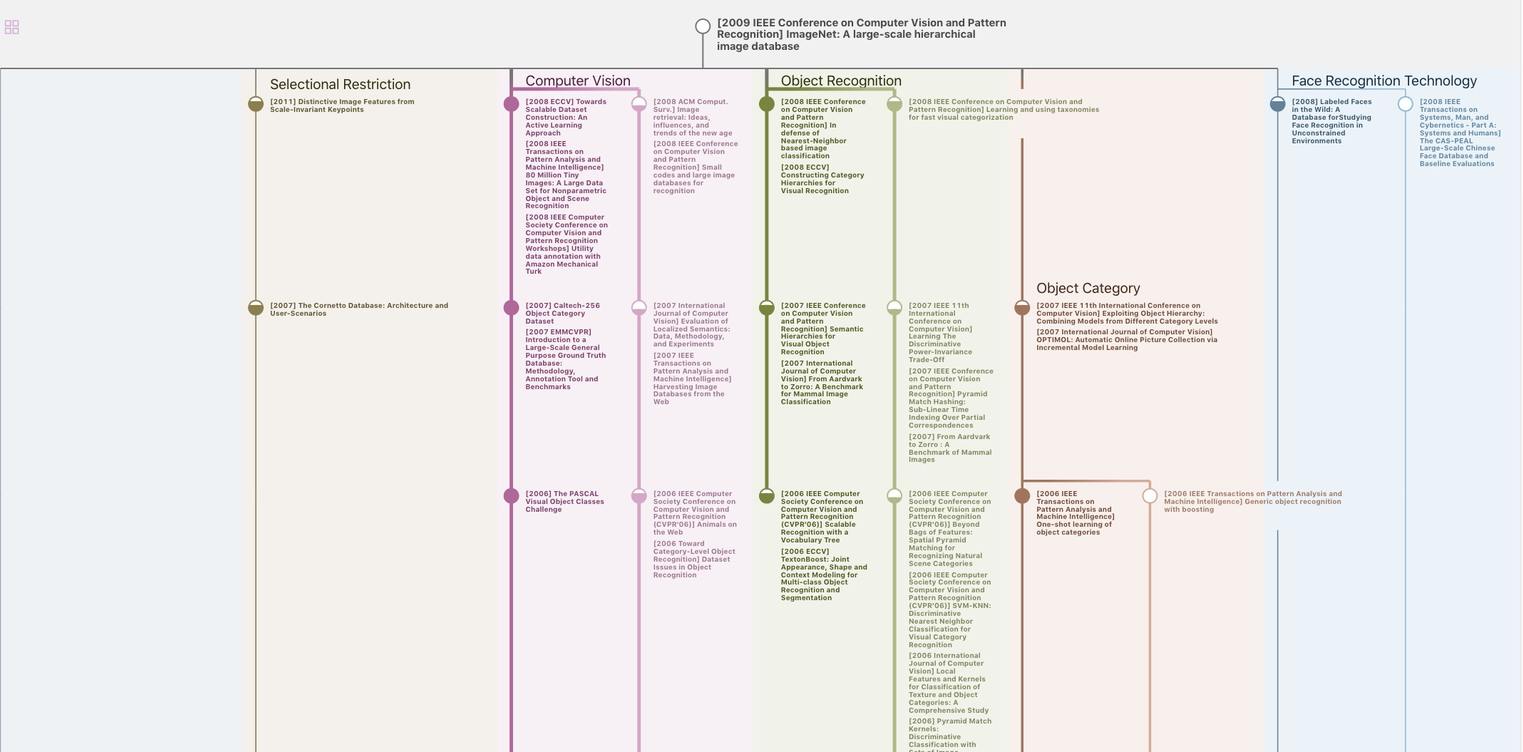
生成溯源树,研究论文发展脉络
Chat Paper
正在生成论文摘要