Assessing the Applicability of Deep-Learning Method for Predicting Cyanobacteria in a Regulated River
JOURNAL OF ENVIRONMENTAL ENGINEERING(2024)
摘要
Cyanobacterial harmful algal blooms (cyanoHABs) caused by cyanobacteria negatively affect humans via river water and aquatic life. Thus, reliable cyanobacteria predictions are essential for managing cyanoHABs. With recent advancements in computer technology and big data usage, artificial intelligence (AI) technologies have gained attention in various fields, such as water resources, weather and climate, and water quality. This study evaluated the applicability of deep-learning-based AI technology for predicting cyanobacteria. A convolutional neural network (CNN)-long short-term memory (LSTM) model, a deep-learning-based AI technology advantageous for predicting time-series data and cyanobacteria features, was built. Its results were analyzed and compared with those of the existing physical Environmental Fluid Dynamics Code (EFDC)-National Institute of Environment Research (NIER) model for cyanobacteria prediction. The CNN-LSTM model performed better, with an accuracy of 69%, which is an improvement over the previous EFDC-NIER model's accuracy of 45%. In particular, there was a dramatic improvement in the prediction accuracy for low cyanobacteria cell counts in Level 1, which increased from 39% to 87%. There also was an improvement in the prediction accuracy for Levels 2 and 3. The accuracy for Level 2 increased from increased from 56% to 69%, and the accuracy for Level 3 increased from 38% to 48%. However, there was a significant decrease in prediction accuracy for high cyanobacteria cell counts in Level 4, for which the measured data were very scarce; accuracy decreased from 49% to 16.7%. The CNN-LSTM model yielded better overall prediction performance than the EFDC-NIER model, demonstrating its applicability in cyanobacteria prediction. However, it has limitations of overfitting areas with inadequate data and not accurately predicting patterns that have not occurred in the past. To address this issue, we propose an approach the combines the advantages of physics-based models and AI-based deep learning models, creating a hybrid concept.
更多查看译文
关键词
Cyanobacteria,Cyanobacterial harmful algal blooms (CyanoHABs),Deep-learning-based artificial intelligence (AI) technology,Convolutional neural network (CNN)-long short-term memory (LSTM),Hybrid model
AI 理解论文
溯源树
样例
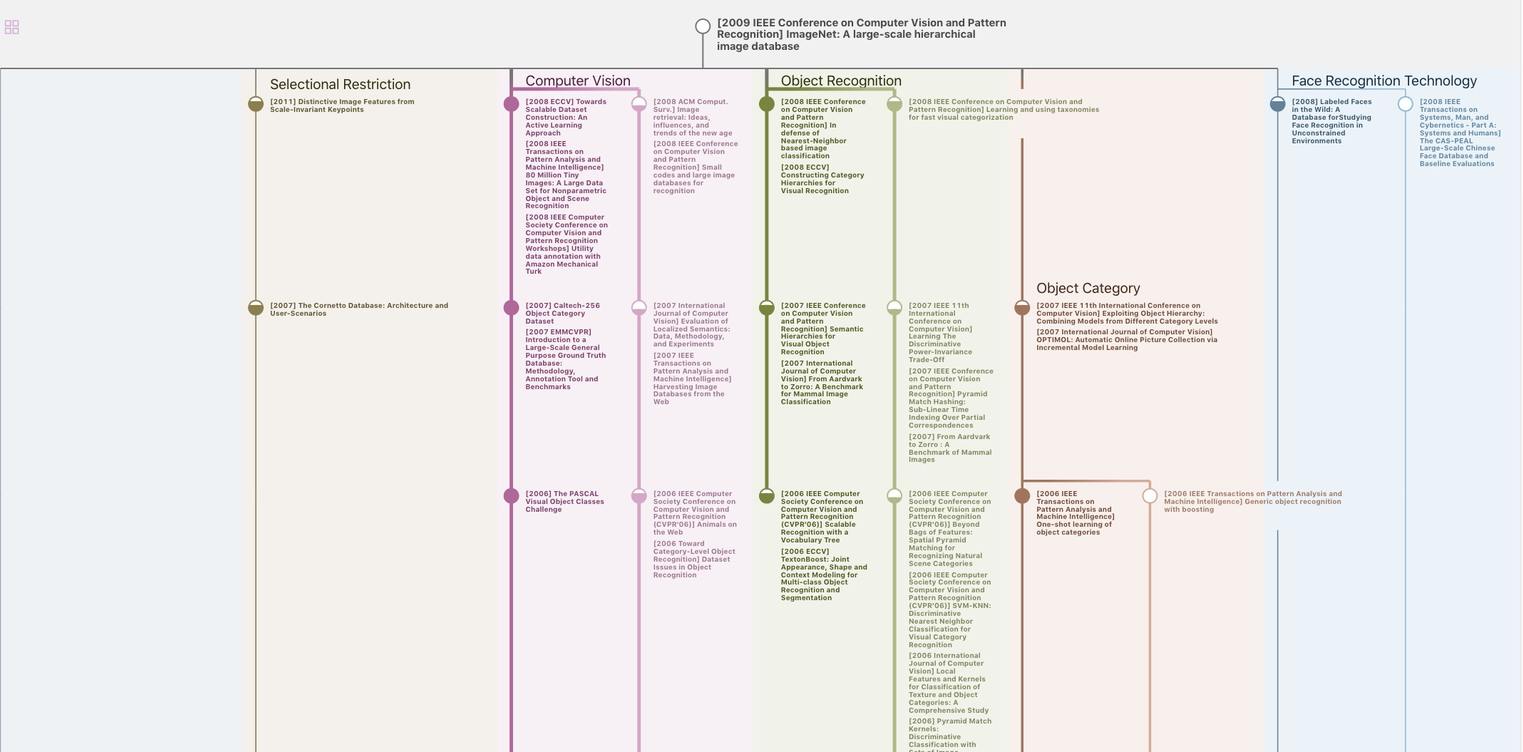
生成溯源树,研究论文发展脉络
Chat Paper
正在生成论文摘要