Explicit solutions for the asymptotically optimal bandwidth in cross-validation
BIOMETRIKA(2024)
摘要
We show that least-squares cross-validation methods share a common structure that has an explicit asymptotic solution, when the chosen kernel is asymptotically separable in bandwidth and data. For density estimation with a multivariate Student-t(nu) kernel, the cross-validation criterion becomes asymptotically equivalent to a polynomial of only three terms. Our bandwidth formulae are simple and noniterative, thus leading to very fast computations, their integrated squared-error dominates traditional cross-validation implementations, they alleviate the notorious sample variability of cross-validation and overcome its breakdown in the case of repeated observations. We illustrate our method with univariate and bivariate applications, of density estimation and nonparametric regressions, to a large dataset of Michigan State University academic wages and experience.[Received on 15 March 2023. Editorial decision on 30 January 2024]
更多查看译文
关键词
Academic wage distribution,Bandwidth choice,Cross-validation,Explicit analytical solution,Nonparametric density estimation
AI 理解论文
溯源树
样例
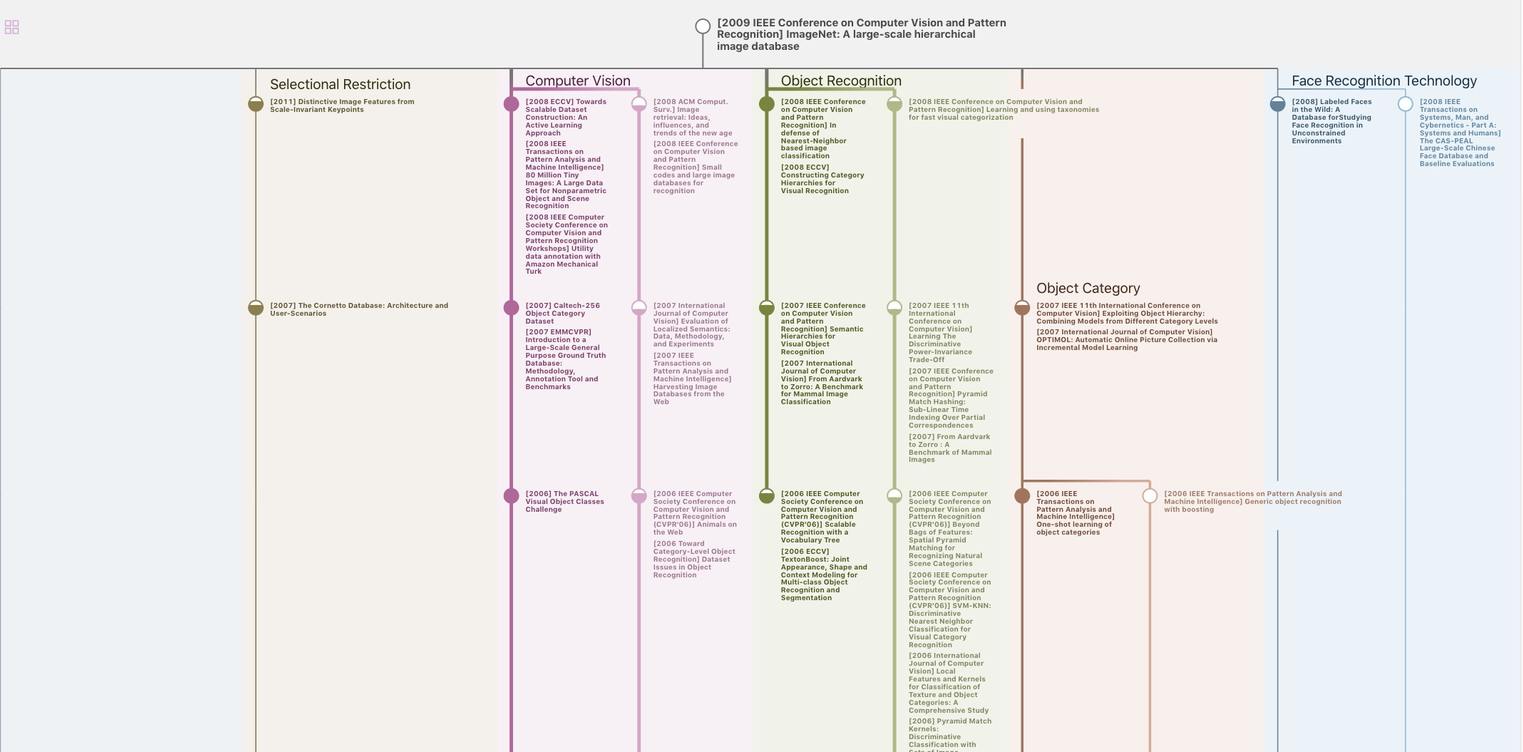
生成溯源树,研究论文发展脉络
Chat Paper
正在生成论文摘要