Ensembling Supervised and Unsupervised Machine Learning Algorithms for Detecting Distributed Denial of Service Attacks
ALGORITHMS(2024)
摘要
The distributed denial of service (DDoS) attack is one of the most pernicious threats in cyberspace. Catastrophic failures over the past two decades have resulted in catastrophic and costly disruption of services across all sectors and critical infrastructure. Machine-learning-based approaches have shown promise in developing intrusion detection systems (IDSs) for detecting cyber-attacks, such as DDoS. Herein, we present a solution to detect DDoS attacks through an ensemble-based machine learning approach that combines supervised and unsupervised machine learning ensemble frameworks. This combination produces higher performance in detecting known DDoS attacks using supervised ensemble and for zero-day DDoS attacks using an unsupervised ensemble. The unsupervised ensemble, which employs novelty and outlier detection, is effective in identifying prior unseen attacks. The ensemble framework is tested using three well-known benchmark datasets, NSL-KDD, UNSW-NB15, and CICIDS2017. The results show that ensemble classifiers significantly outperform single-classifier-based approaches. Our model with combined supervised and unsupervised ensemble models correctly detects up to 99.1% of the DDoS attacks, with a negligible rate of false alarms.
更多查看译文
关键词
network security,DDoS attack detection,machine learning,ensemble
AI 理解论文
溯源树
样例
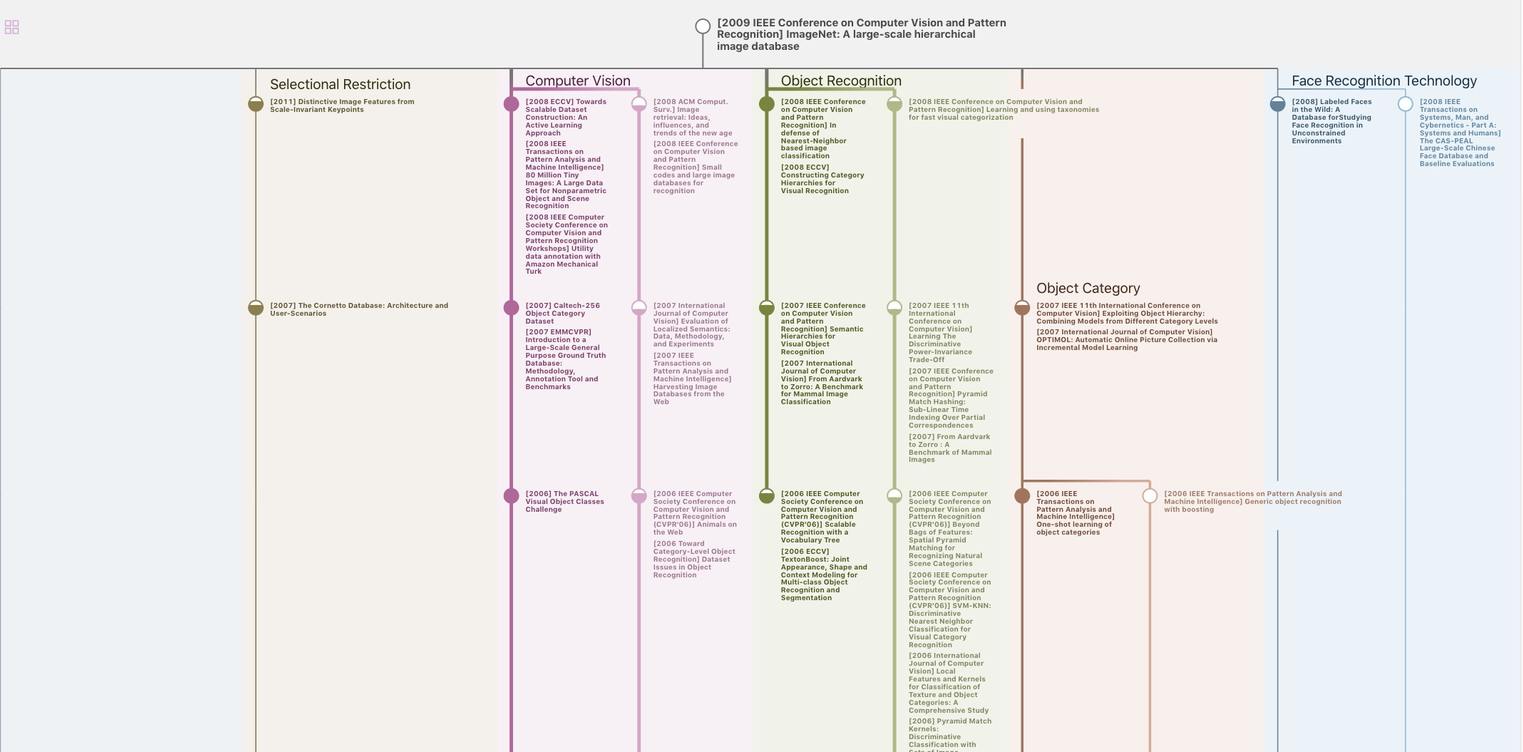
生成溯源树,研究论文发展脉络
Chat Paper
正在生成论文摘要