On variational inference and maximum likelihood estimation with the -exponential family
FOUNDATIONS OF DATA SCIENCE(2024)
摘要
The lambda-exponential family has recently been proposed to generalize the exponential family. While the exponential family is well-understood and widely used, this is not the case yet for the A-exponential family. However, many applications require models that are more general than the exponential family, and the lambda-exponential family is often a good alternative. In this work, we propose a theoretical and algorithmic framework to solve variational inference and maximum likelihood estimation problems over the lambda-exponential family. We give new sufficient optimality conditions for variational inference problems. Our conditions take the form of generalized moment-matching conditions and generalize existing similar results for the exponential family. We exhibit novel characterizations of the solutions of maximum likelihood estimation problems, that recover optimality conditions in the case of the exponential family. For the resolution of both problems, we propose novel proximal-like algorithms that exploit the geometry underlying the A-exponential family. These new theoretical and methodological insights are tested on numerical examples, showcasing their usefulness and interest, especially on heavy-tailed target distributions.
更多查看译文
关键词
Variational inference,maximum likelihood estimation,Renyi diver- gence,A-exponential family,generalized subdifferential,heavy-tailed distribution
AI 理解论文
溯源树
样例
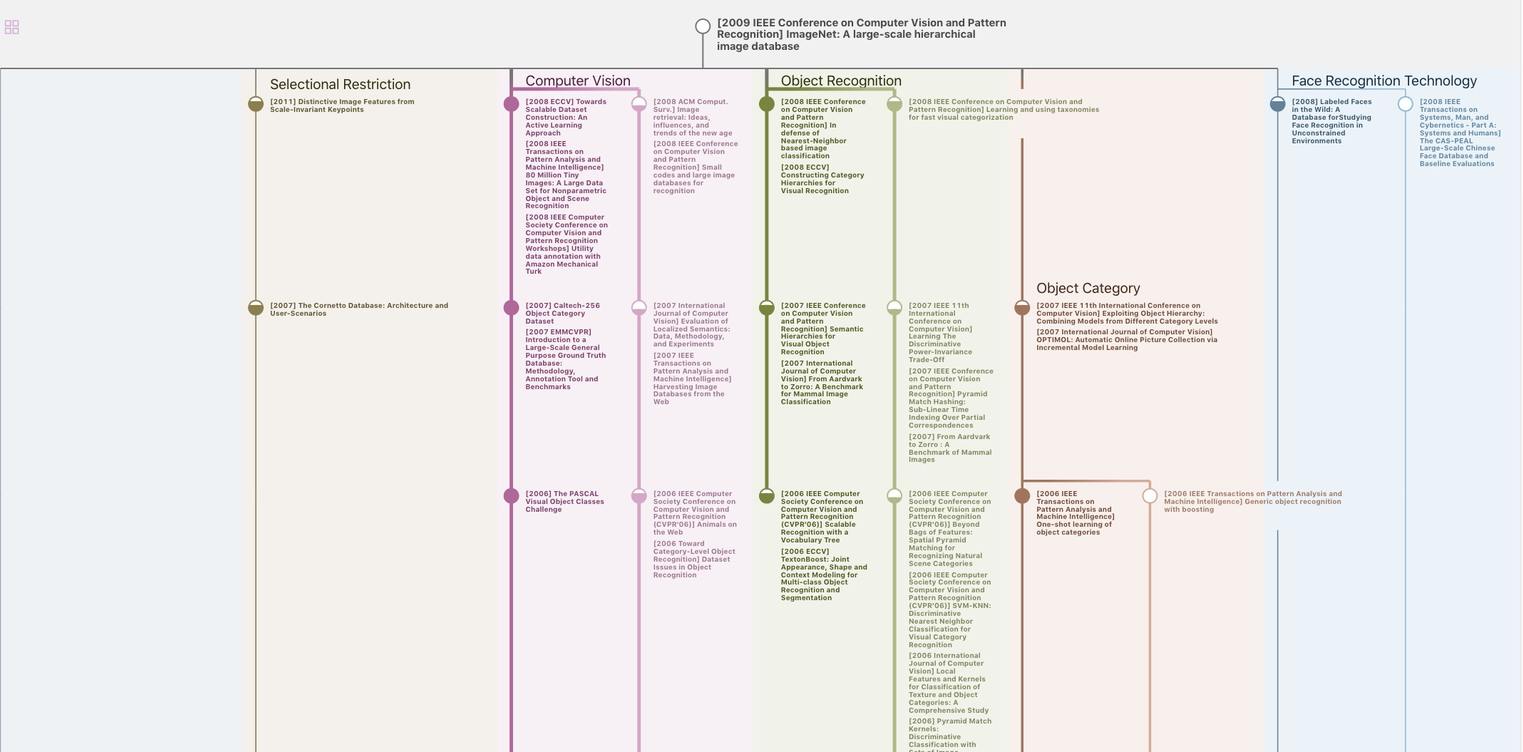
生成溯源树,研究论文发展脉络
Chat Paper
正在生成论文摘要