Adversarial Complementary Learning for Just Noticeable Difference Estimation
KSII TRANSACTIONS ON INTERNET AND INFORMATION SYSTEMS(2024)
摘要
Recently, many unsupervised learning -based models have emerged for Just Noticeable Difference (JND) estimation, demonstrating remarkable improvements in accuracy. However, these models suffer from a significant drawback is that their heavy reliance on handcrafted priors for guidance. This restricts the information for estimating JND simply extracted from regions that are highly related to handcrafted priors, while information from the rest of the regions is disregarded, thus limiting the accuracy of JND estimation. To address such issue, on the one hand, we extract the information for estimating JND in an Adversarial Complementary Learning (ACoL) way and propose an ACoL-JND network to estimate the JND by comprehensively considering the handcrafted priors -related regions and non -related regions. On the other hand, to make the handcrafted priors richer, we take two additional priors that are highly related to JND modeling into account, i.e., Patterned Masking (PM) and Contrast Masking (CM). Experimental results demonstrate that our proposed model outperforms the existing JND models and achieves state-of-the-art performance in both subjective viewing tests and objective metrics assessments.
更多查看译文
关键词
Just Noticeable Difference (JND),convolutional neural networks,Human Visual System (HVS)
AI 理解论文
溯源树
样例
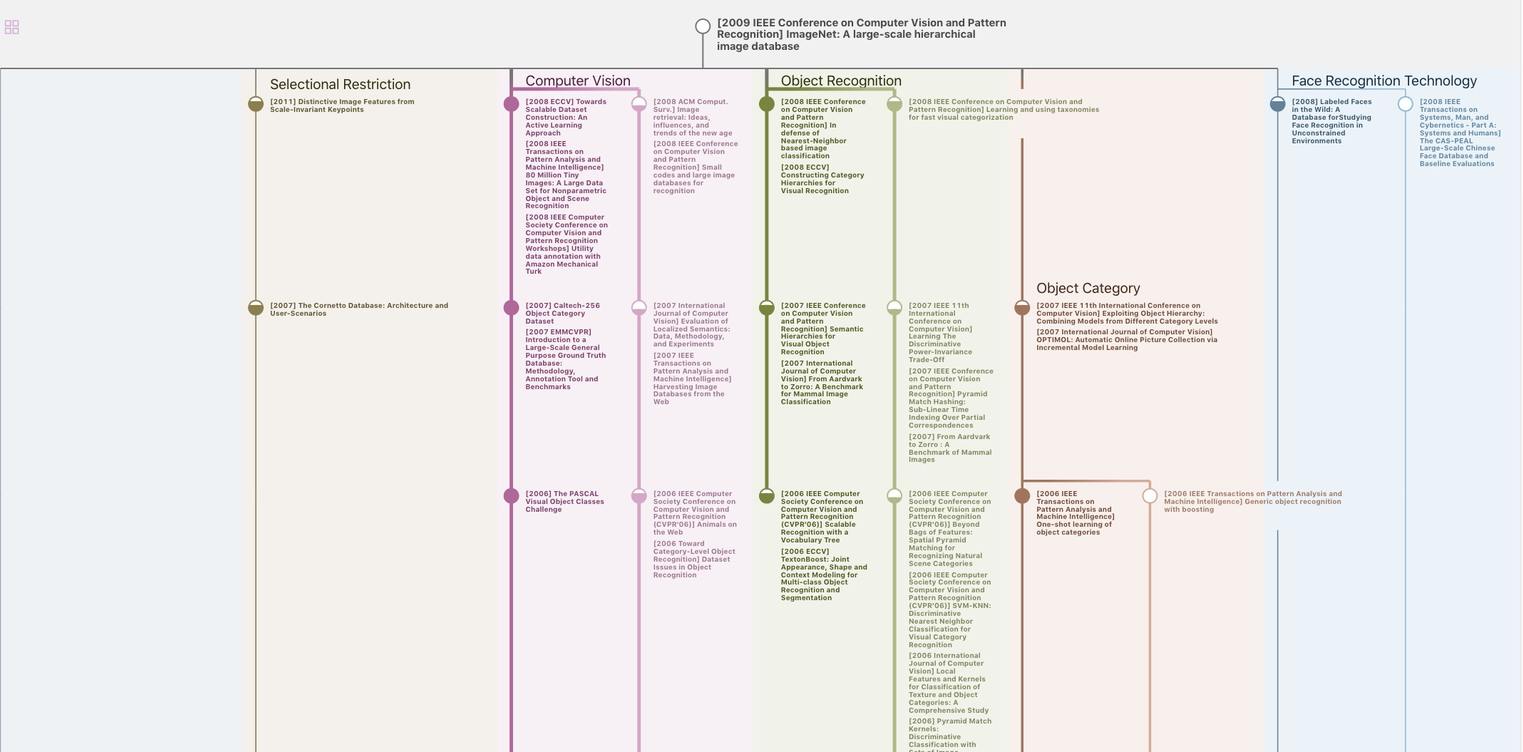
生成溯源树,研究论文发展脉络
Chat Paper
正在生成论文摘要