AnatSwin: An anatomical structure-aware transformer network for cardiac MRI segmentation utilizing label images
NEUROCOMPUTING(2024)
摘要
Despite the extensive utilization of deep learning in medical image segmentation, the achieved accuracy remains inadequate for clinical requirements due to the scarcity of annotated data, which constrains the acquisition of anatomical knowledge. Leveraging anatomical information is particularly advantageous in medical image segmentation, especially for multi -modal and cross -domain tasks. To better capture and represent anatomical structures, we propose a Swin Transformer -based anatomical structure -aware network, AnatSwin, which adopts a unique approach by utilizing label images as inputs. Compared with gray -scale images, label images, devoid of intensity information, explicitly enhance the representation of anatomical shape and spatial tissue relationships, offering valuable resources for learning anatomical structures effectively and allowing the model to concentrate on understanding morphological and spatial relationship cues. AnatSwin follows an encoder-decoder architecture, where the encoder incorporates two branches that share weights. The Swin-Transformer block serves as the basic unit of the encoder, accepting both the template label (representing the correct anatomical structure) and the pseudo label (generated by a registration model) as inputs. In order to facilitate efficient interaction among features at the same hierarchy, an attention -based feature interaction (FI) block is introduced. FI block enhances the model's ability to capture anatomical structure by promoting feature interactions within the two branches. Furthermore, the decoder employs FI blocks to learn relationships between features at the same hierarchy, ultimately improving the segmentation performance. Experimental evaluations demonstrate that the proposed AnatSwin outperforms state-of-theart models, highlighting its significant potential in improving the learning and representation of anatomical structures, as well as optimizing tasks related to medical image segmentation. This work signifies a promising step forward in addressing the challenges of medical image segmentation and paves the way for further advancements in the field.
更多查看译文
关键词
Anatomical structure,Deep learning,Medical image segmentation,Transformer
AI 理解论文
溯源树
样例
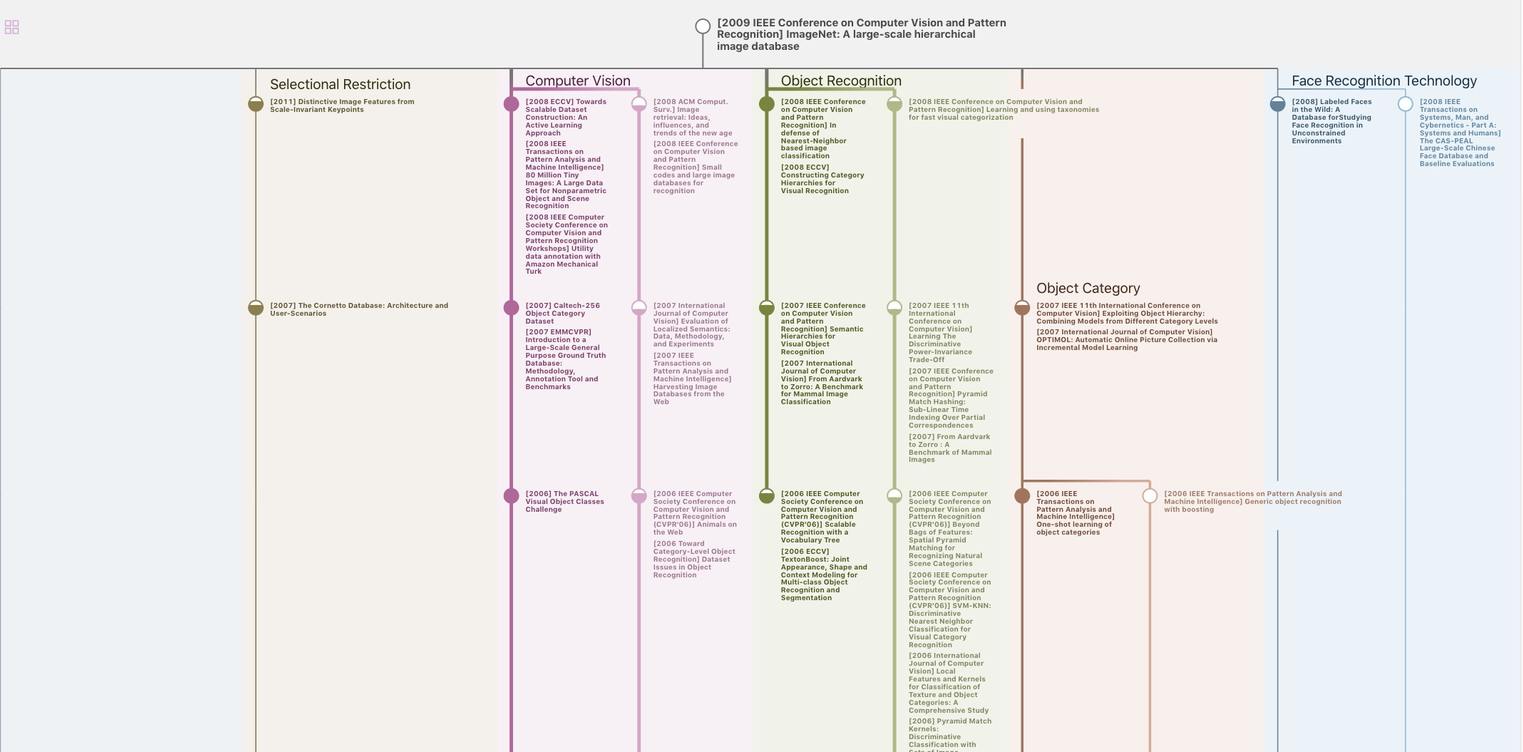
生成溯源树,研究论文发展脉络
Chat Paper
正在生成论文摘要