Parameterized L0 Image Smoothing With Unsupervised Learning
IEEE TRANSACTIONS ON EMERGING TOPICS IN COMPUTATIONAL INTELLIGENCE(2024)
摘要
The traditional L-0 filter shows exquisite smoothing quality, but it suffers from high computational cost. In this paper, we propose an efficient solution to the L-0 -regularized optimization problem based on deep unsupervised learning. The L-0-norm involves a discrete counting scheme, which can not be directly optimized with gradient descent. Therefore, in this paper, we propose to decompose the problem into a series of optimization problems based on a truncated L-1-norm with varying parameters. Comparedwith the truncated L-2-norm explored in traditional L-0 filter, the truncated L-1 -norm promotes the capabilities in structure- and edge-preserving smoothing, reduces the number of iterations, and facilitates the deep learning-based optimization. Furthermore, we propose a deep learning-based parameterized approach to solve the truncated L-1 -regularized problems so that we only need to train a single fully convolutional network to support varying smoothing parameters. We are not trying to reproduce the traditional L-0 filter in this paper. Instead, we show that the proposed deep L-0 filter provides a better smoothing quality. Experimental results indicate that the proposed filter outperforms the state-of-the-art on various applications, including edge-preserving smoothing, non-photorealistic rendering, texture removal, edge extraction, image composition, and compression artifact removal. Moreover, our filter is efficient, it is able to process 720P color images at interactive rates on a modern GPU.
更多查看译文
关键词
Computational photography,image smoothing,L-0 filter,unsupervised learning
AI 理解论文
溯源树
样例
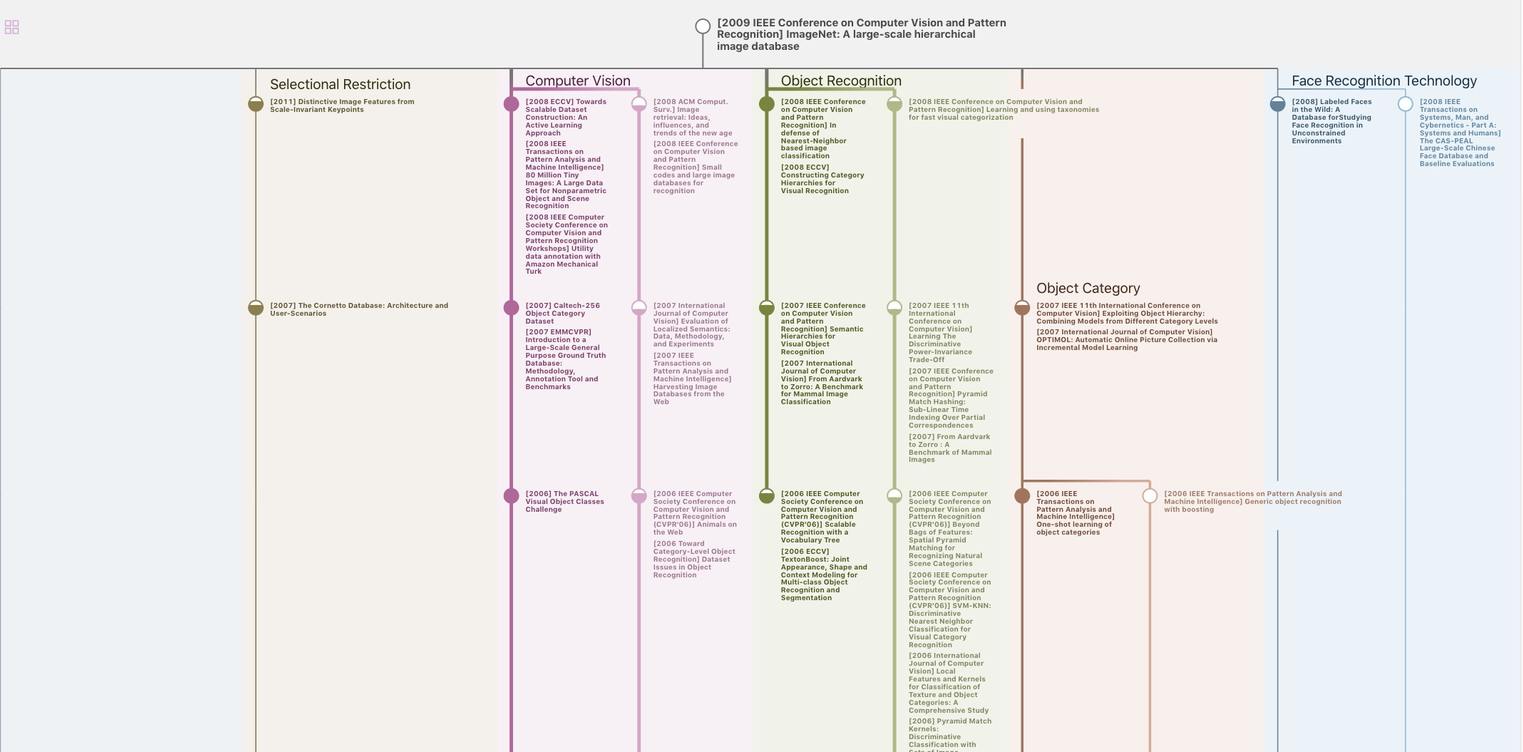
生成溯源树,研究论文发展脉络
Chat Paper
正在生成论文摘要