A machine learning proxy based multi-objective optimization method for low-carbon hydrogen production
JOURNAL OF CLEANER PRODUCTION(2024)
摘要
With industrial informatization, rich data frameworks provide the possibility of efficient design and global optimization of methane to hydrogen processes. However, the high coupling between mixed variables leads to the difficulty of first -principles modeling (FPM) to capture the optimal Pareto front in infeasible domains. In this paper, a machine learning proxy (MLP) method is proposed to minimize economic, exergy destruction, CO2 emissions, and maximize hydrogen production. First, process simulation as FPM is built using physical properties, energy balance, and reaction kinetics to analyze single variable effects and objective trade-offs. Then, datasets consisting of feed, heat transfer, and design variables are built by sampling the FPM in design and extended spaces. The eXtreme Gradient Boosting (XGBoost) with hyperparameter optimization is built as a proxy FPM, capturing the complex mapping between variables and objectives. Finally, the Pareto front is searched in design and extended spaces by combining the proxy model and genetic algorithm. The proposed method benefits 20.2%, 37.2%, 46.7%, and 2.3% in economic, exergy destruction, CO2 emissions and hydrogen production respectively compared to the heuristic design. XGBoost not only can effectively proxy FPM to capture the complex behavior of reforming techniques, but also can perform better in infeasible domains that are difficult to explore with FPM.
更多查看译文
关键词
Multi-objective optimization,Machine learning,Hydrogen,CO2 emission,Methane dual reforming
AI 理解论文
溯源树
样例
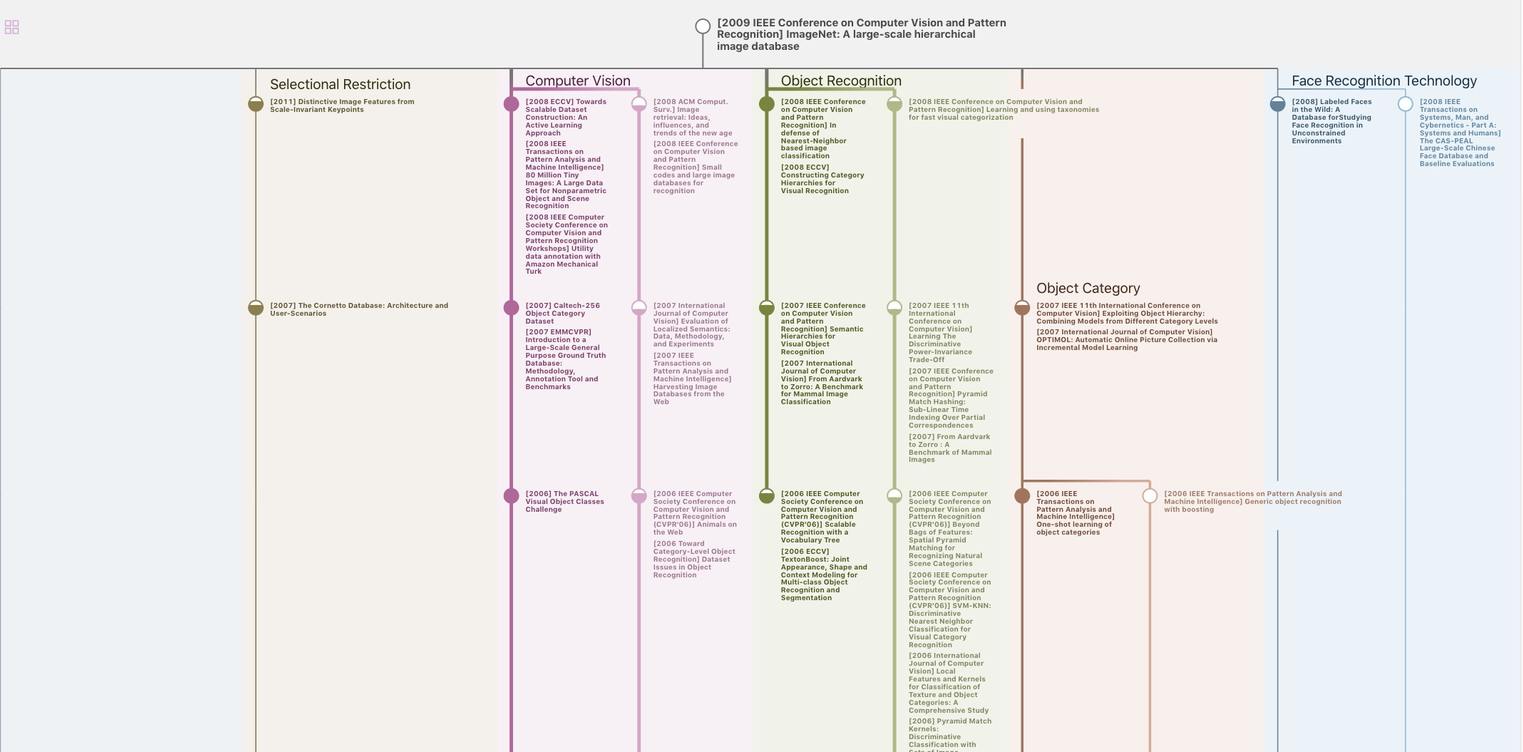
生成溯源树,研究论文发展脉络
Chat Paper
正在生成论文摘要