Correlation concept-cognitive learning model for multi-label classification
KNOWLEDGE-BASED SYSTEMS(2024)
摘要
As a cognitive process, concept -cognitive learning (CCL) emphasizes the structured expression of data through systematic cognition and understanding, to obtain valuable information in the data. Although concept -cognitive learning has achieved good results in single -label classification tasks, it has not yet been applied to multi -label learning. The difficulty is that the existing concept -cognitive learning fails to effectively associate and utilize the structural relationships in the feature concepts and the multi -label concepts. In addition, in the face of complex multi -label datasets, it is difficult to obtain effective concepts for classification tasks. To solve these problems, this paper proposes a correlation concept -cognitive learning method and applies it to the multi -label classification (MLC) task. Moreover, the relationship between the feature concepts and the multi -label concepts is established by extent. On this basis, we comprehensively consider the extent relevancy and intent relevancy, and learn correlation concepts. In order to improve the classification precision, we construct correlation concept spaces to obtain the representation of effective concepts. Finally, we conducted experimental evaluations on ten datasets to illustrate the effectivity and advantages of the proposed approach.
更多查看译文
关键词
Concept-cognitive learning,Granular computing,Formal concept analysis,Multi-label learning,Label correlations
AI 理解论文
溯源树
样例
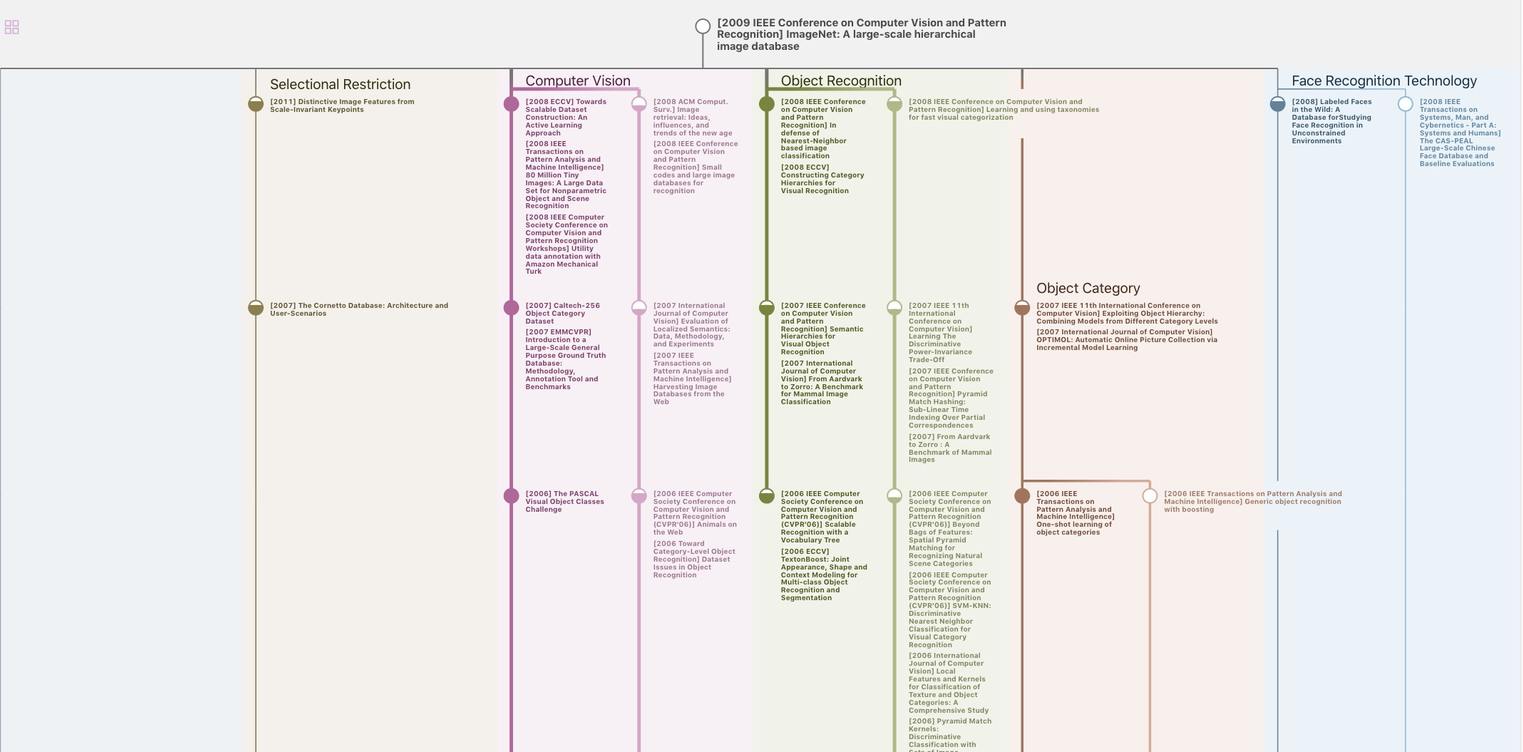
生成溯源树,研究论文发展脉络
Chat Paper
正在生成论文摘要