Artificial Intelligence in Stroke Imaging: A Comprehensive Review
EURASIAN JOURNAL OF MEDICINE(2023)
摘要
The aging population challenges the health-care system with chronic diseases. Cerebrovascular diseases are important components of these chronic conditions. Stroke is the acute cessation of blood in the brain, which can lead to rapid tissue loss. Therefore, fast, accurate, and reliable automatic methods are required to facilitate stroke management. The performance of artificial intelligence (AI) methods is increasing in all domains. Vision tasks, including natural images and medical images, are particularly benefiting from the skills of AI models. The AI methods that can be applied to stroke imaging have a broad range, including classical machine learning tools such as support vector machines, random forests, logistic regression, and linear discriminant analysis, as well as deep learning models, such as convolutional neural networks, recurrent neural networks, autoencoders, and U -Net. Both tools can be applied to various aspects of stroke management, including time-to-event onset determination, stroke confirmation, large vessel occlusion detection, diffusion restriction, perfusion deficit, core and penumbra identification, affected region segmentation, and functional outcome prediction. While building these AI models, maximum care should be exercised in order to reduce bias and build generalizable models. One of the most important prerequisites for building unbiased models is collecting large, diverse, and quality data that reflects the underlying population well and splitting the training and testing parts in a way that both represent a similar distribution. Explainability and trustworthiness are other important properties of machine learning models that could be widely adopted in clinical practices.
更多查看译文
关键词
Artificial intelligence,convolutional neural network,deep learning,infarct region,radiomics,stroke
AI 理解论文
溯源树
样例
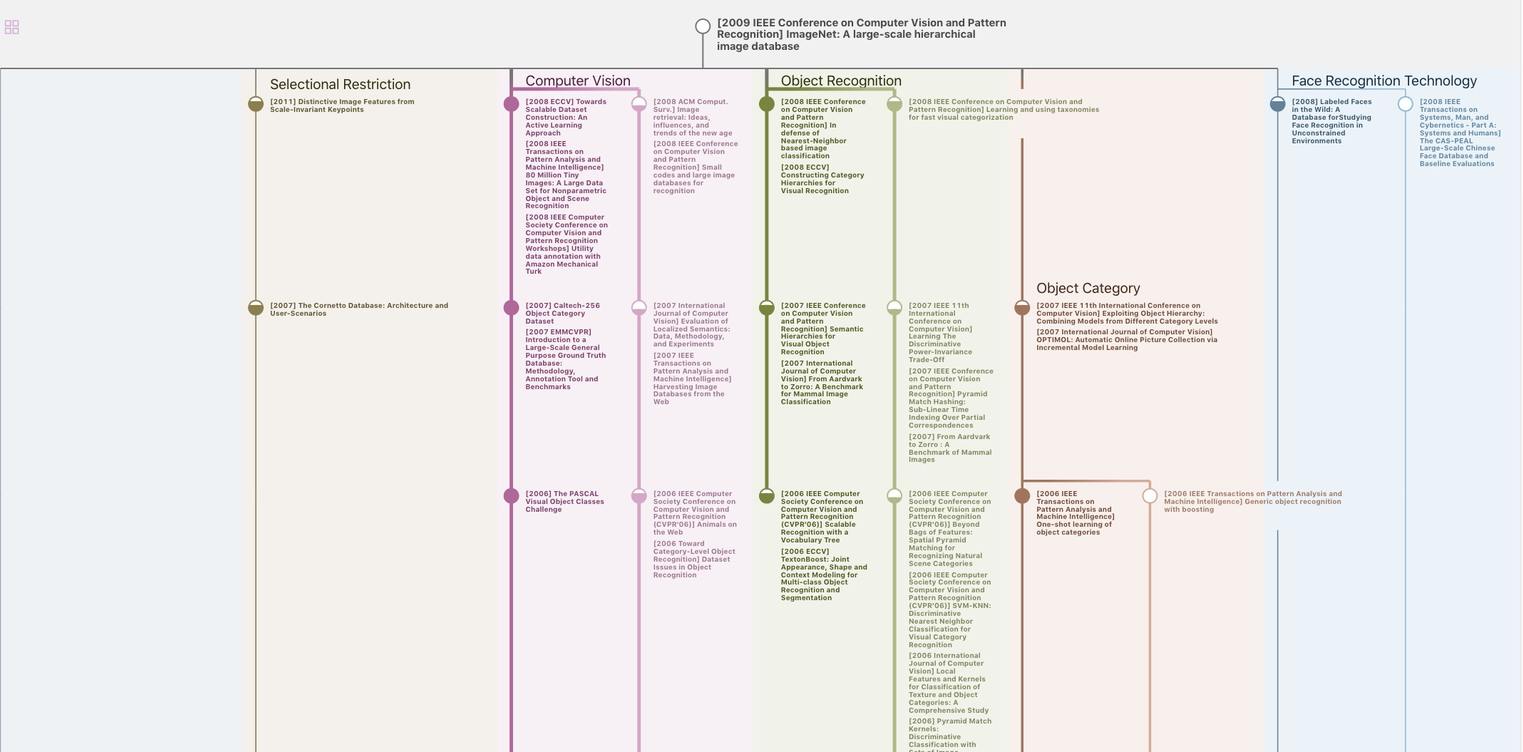
生成溯源树,研究论文发展脉络
Chat Paper
正在生成论文摘要