Poor Man's Quality Estimation: Predicting Reference-Based MT MetricsWithout the Reference
17TH CONFERENCE OF THE EUROPEAN CHAPTER OF THE ASSOCIATION FOR COMPUTATIONAL LINGUISTICS, EACL 2023(2023)
摘要
Machine translation quality estimation (QE) predicts human judgements of a translation hypothesis without seeing the reference. Stateof-the-art QE systems based on pretrained language models have been achieving remarkable correlations with human judgements yet they are computationally heavy and require human annotations, which are slow and expensive to create. To address these limitations, we define the problem of metric estimation (ME) where one predicts the automated metric scores also without the reference. We show that even without access to the reference, our model can estimate automated metrics (rho=60% for BLEU, rho=51% for other metrics) at the sentence-level. Because automated metrics correlate with human judgements, we can leverage the ME task for pre-training a QE model. For the QE task, we find that pre-training on TER is better (rho=23%) than training for scratch (rho=20%).
更多查看译文
AI 理解论文
溯源树
样例
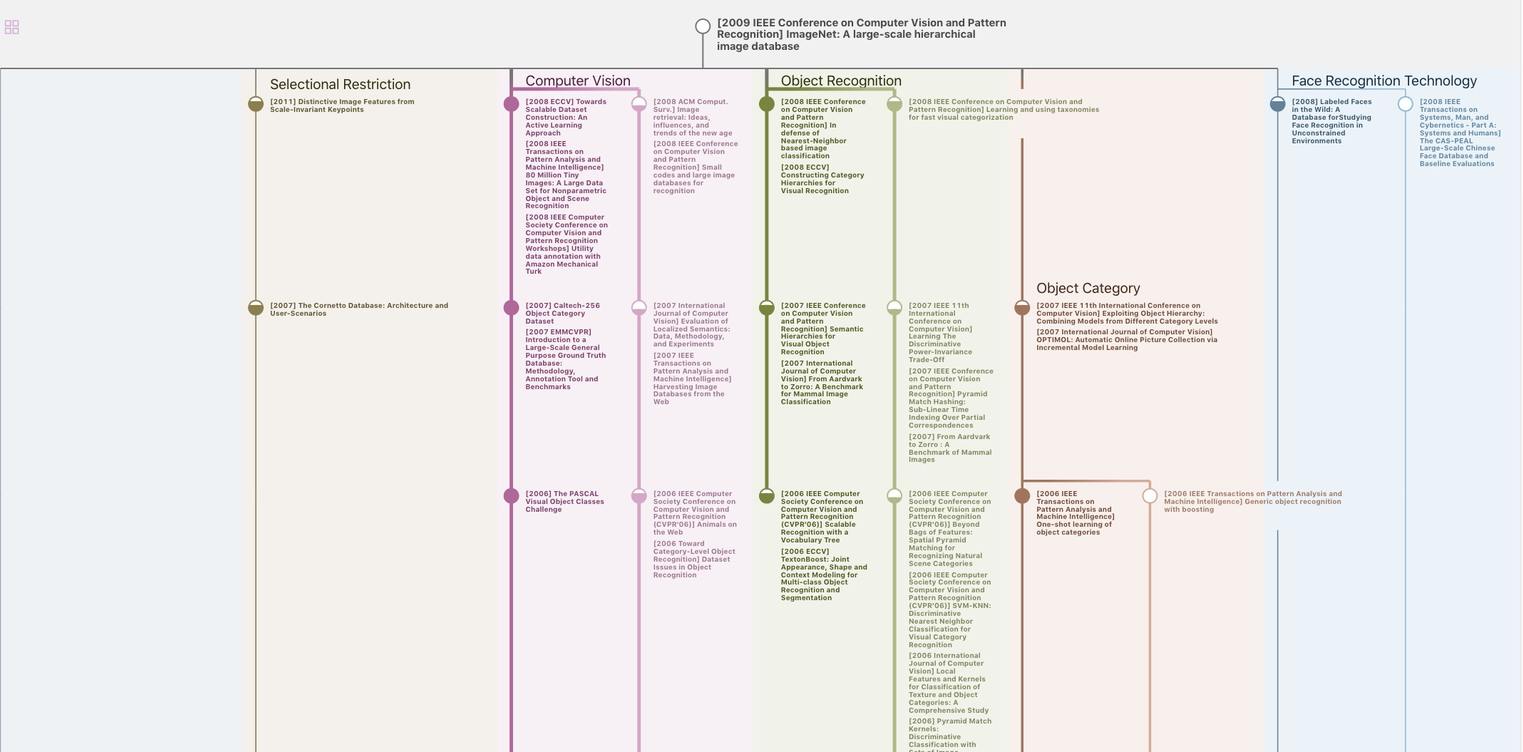
生成溯源树,研究论文发展脉络
Chat Paper
正在生成论文摘要