MeshPointNet: 3D Surface Classification Using Graph Neural Networks and Conformal Predictions on Mesh-Based Representations
JOURNAL OF MECHANICAL DESIGN(2024)
摘要
In many design automation applications, accurate segmentation and classification of 3D surfaces and extraction of geometric insight from 3D models can be pivotal. This paper primarily introduces a machine learning-based scheme that leverages graph neural networks for handling 3D geometries, specifically for surface classification. Our model demonstrates superior performance against two state-of-the-art models, PointNet + + and PointMLP, in terms of surface classification accuracy, beating both models. Central to our contribution is the novel incorporation of conformal predictions, a method that offers robust uncertainty quantification and handling with marginal statistical guarantees. Unlike traditional approaches, conformal predictions enable our model to ensure precision, especially in challenging scenarios where mistakes can be highly costly. This robustness proves invaluable in design applications, and as a case in point, we showcase its utility in automating the computational fluid dynamics meshing process for aircraft models based on expert guidance. Our results reveal that our automatically generated mesh, guided by the proposed rules by experts enabled through the segmentation model, is not only efficient but matches the quality of expert-generated meshes, leading to accurate simulations.
更多查看译文
关键词
mesh,deep learning,graph neural networks,conformal predictions,GNN,mesh segmentation,3D model segmentation,CFD,data-driven design,design automation,design evaluation,simulation-based design
AI 理解论文
溯源树
样例
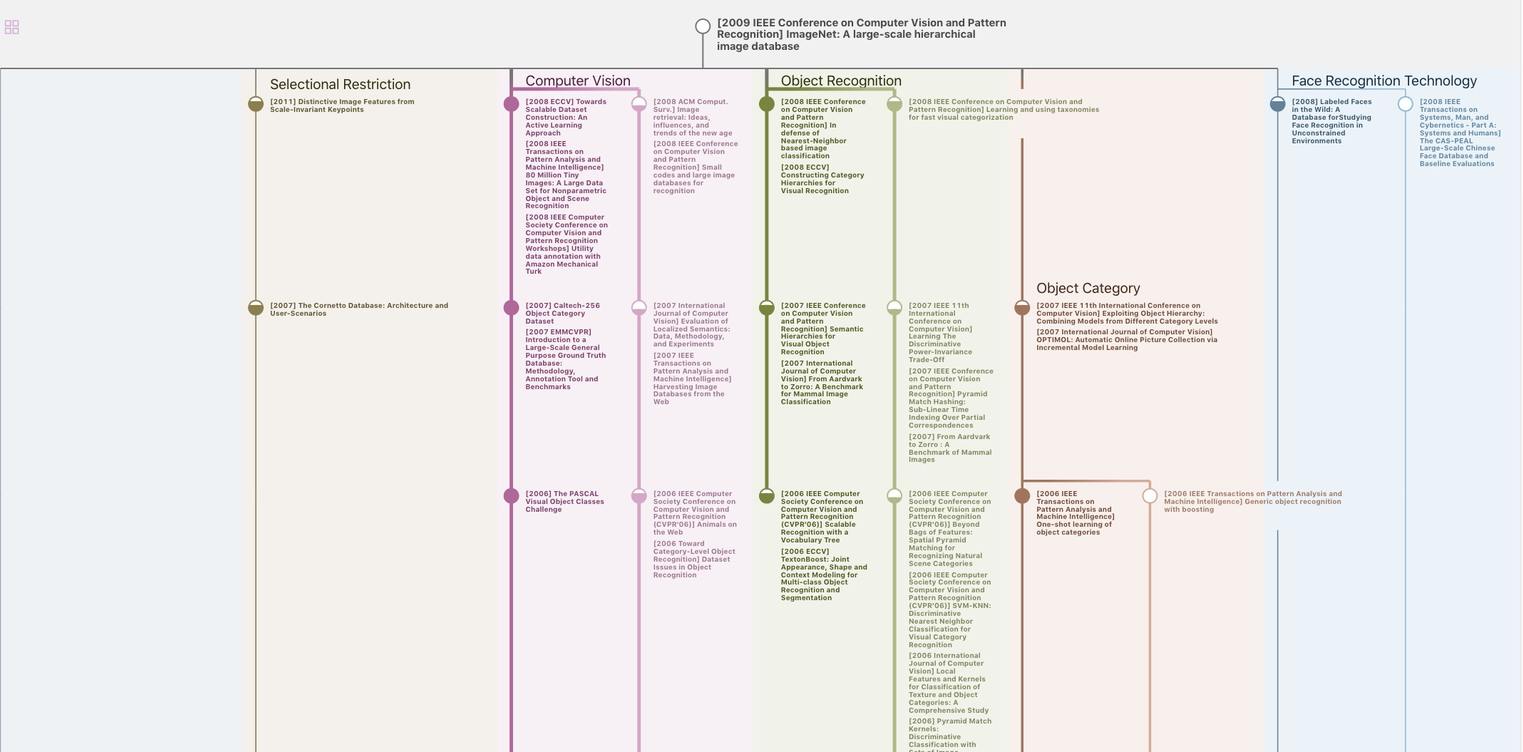
生成溯源树,研究论文发展脉络
Chat Paper
正在生成论文摘要