A data-driven hybrid sensor fault detection/diagnosis method with flight test data
MEASUREMENT SCIENCE AND TECHNOLOGY(2024)
摘要
Fault diagnosis of multi-source signal systems is critical for the safe and reliable operation of modern industrial systems, and accurate fault diagnosis of systems based on multi-source signals remains challenging. This study proposes a data-driven hybrid fault detection/diagnosis method to identify sensor faults in complex systems through interactions between multiple sensors. Dynamic mode decomposition with control is used to obtain the approximate model of the investigated system from multi-source signals, extract the underlying physical mechanisms, combined with the Kalman filter observer to generate the residual between the observed data and the predicted data. Then the residual (moving innovation covariance matrix V k ) is input into the k-nearest neighbor classification algorithm for fault detection and diagnosis. The effectiveness of the proposed method was evaluated using the flight test braking system dataset. The results showed that the accuracy of the proposed method in fault detection and diagnosis (with accuracies of 100% and 100%, respectively) was significantly improved than that of using raw signal data (76.6% and 6.38%) or raw signal data and V k (80.85% and 42.55%). The analysis of different parameters including fault severity, algorithm hyperparameter k , and sensor type showed that the proposed method has high robustness, generalization ability, and practicality.
更多查看译文
关键词
fault detection/diagnosis,dynamic mode decomposition,Kalman filter,k-nearest neighbor
AI 理解论文
溯源树
样例
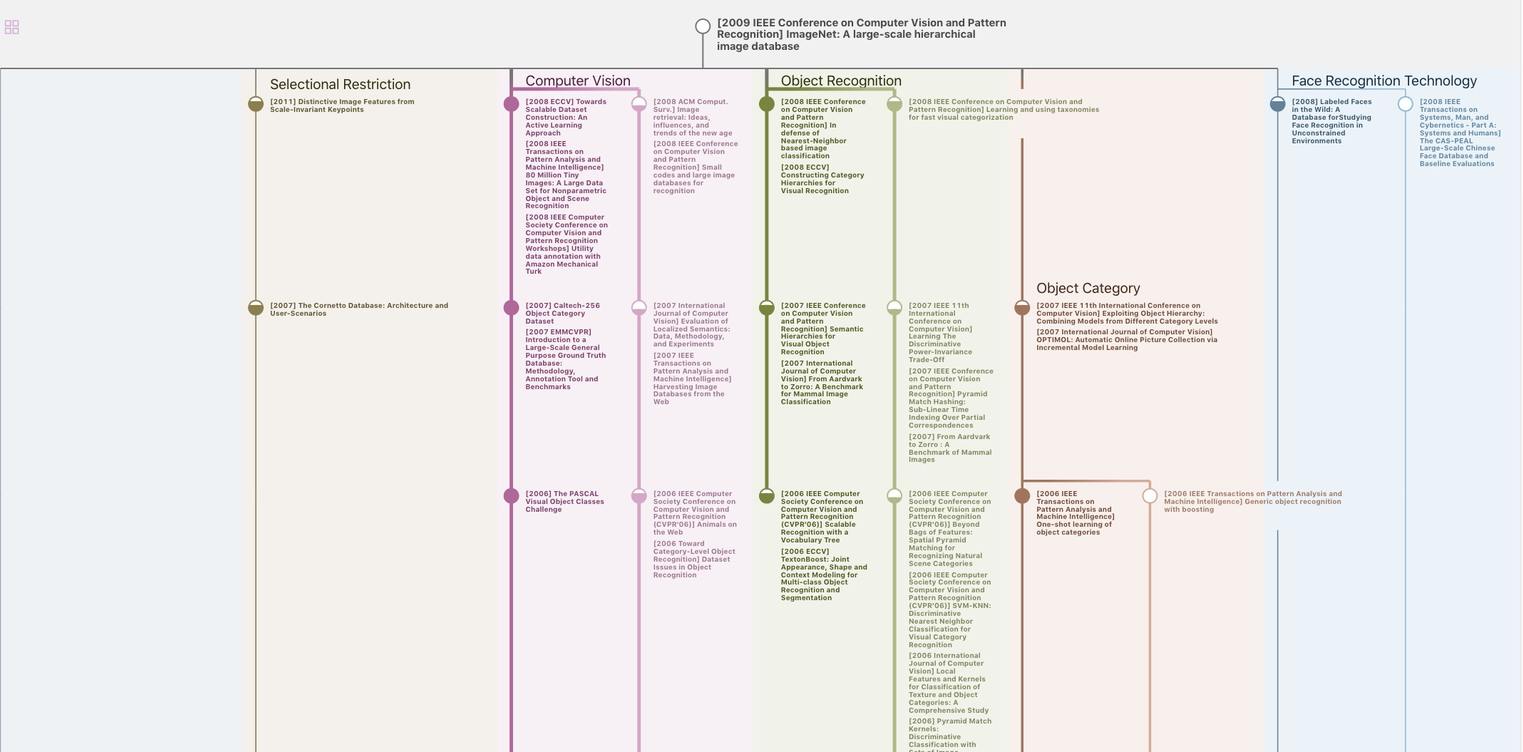
生成溯源树,研究论文发展脉络
Chat Paper
正在生成论文摘要