Weak-formulated physics-informed modeling and optimization for heterogeneous digital materials
PNAS Nexus(2024)
摘要
Abstract Numerical solutions to partial differential equations (PDEs) are instrumental for material structural design where extensive data screening is needed. However, traditional numerical methods demand significant computational resources, highlighting the need for innovative optimization algorithms to streamline design exploration. Direct gradient-based optimization algorithms, while effective, rely on design initialization and require complex, problem-specific sensitivity derivations. The advent of machine learning offers a promising alternative to handling large parameter spaces. To further mitigate data dependency, researchers have developed physics-informed neural networks (PINNs) to learn directly from PDEs. However, the intrinsic continuity requirement of PINNs restricts their application in structural mechanics problems, especially for composite materials. Our work addresses this discontinuity issue by substituting the PDE residual with a weak formulation in the physics-informed training process. The proposed approach is exemplified in modeling digital materials, which are mathematical representations of complex composites that possess extreme structural discontinuity. This paper also introduces an interactive process that integrates physics-informed loss with design objectives, eliminating the need for pre-trained surrogate models or analytical sensitivity derivations. The results demonstrate that our approach can preserve the physical accuracy in data-free material surrogate modeling but also accelerates the direct optimization process without model pre-training.
更多查看译文
AI 理解论文
溯源树
样例
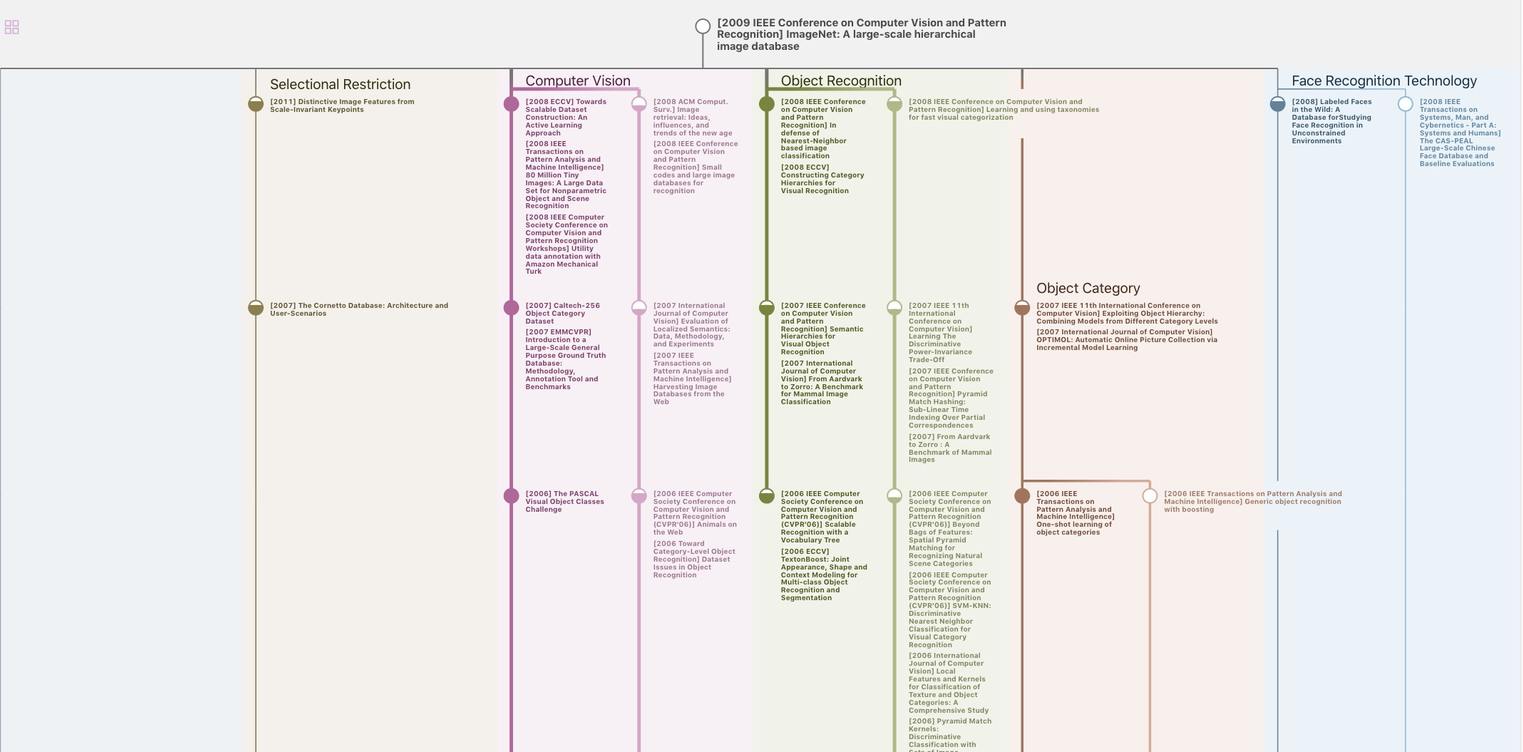
生成溯源树,研究论文发展脉络
Chat Paper
正在生成论文摘要