Attribute Enhanced Graph Attention Networks for Session-based Recommendation.
International Conference on Advanced Cloud and Big Data(2023)
摘要
Session-based recommendation (SBR) aims to predict the next item that the anonymous users will interact with based on their short behavior sequence. However, most of the current approaches towards SBR only focus on modeling users’ interest preferences based on a series of items, ignoring extra item attributes, i.e., the brand attribute. This paper proposes a novel approach, called the Attribute Enhanced Graph Attention Networks for Session-based Recommendation (AGSR), using the brand attribute to better model user preferences. Specifically, (i) AGSR constructs the session graph of the current session to capture the user’s session-level item embedding; (ii) AGSR constructs the brand global graph to capture the brand-level item embedding and brand embedding; (iii) Based on the learned node embeddings, AGSR apply attention layers to extract users’ interest and brand preferences. Finally, AGSR makes the recommendation based on item features and user interest and brand preferences, so as to provide better recommendations. Extensive experiments on three real-world datasets demonstrate the effectiveness of our approach.
更多查看译文
关键词
Session-based recommendation,Graph attention networks,Brand preferences,Interest preferences
AI 理解论文
溯源树
样例
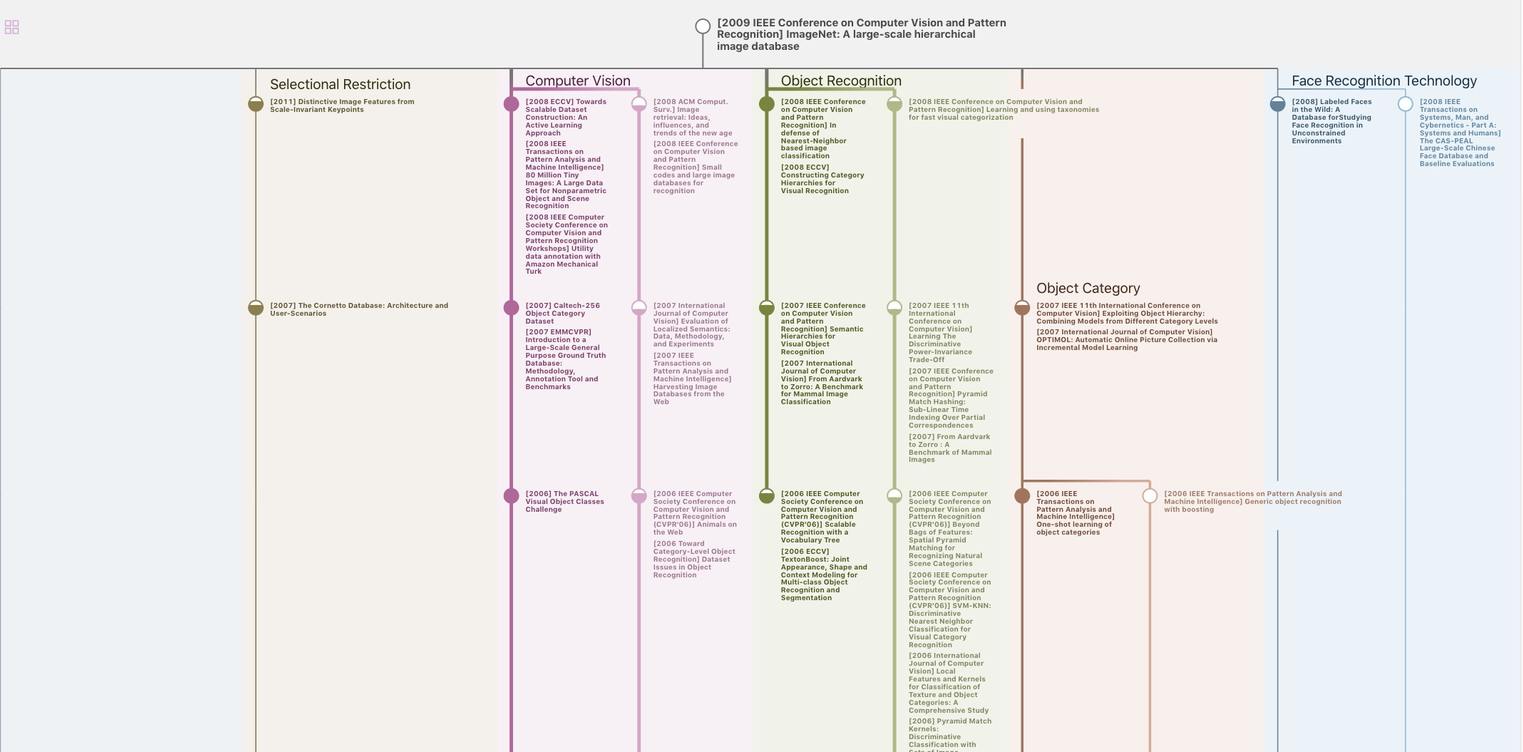
生成溯源树,研究论文发展脉络
Chat Paper
正在生成论文摘要