CamoNet : On-Device Neural Network Adaptation With Zero Interaction and Unlabeled Data for Diverse Edge Environments
IEEE Transactions on Mobile Computing(2024)
摘要
Deploying deep learning models to edge devices for low-latency and privacy-preserving applications has become a trend. To adapt to heterogeneous devices and data, it is significant to generate customized models. However, existing model adaptation approaches require edge devices to make interactions (collecting hardware information or local data) with the cloud, which raises privacy concerns, increases communication costs, and burdens the cloud. By contrast, we propose
CamoNet
, a universal on-device model adaptation framework with zero interaction between devices and the cloud. In
CamoNet
, a lightweight on-device neural architecture search module is utilized to quickly generate a customized model for subsequent on-device training, followed by an on-device contrastive transfer learning module to effectively leverage unlabeled data for fine-tuning the customized model. Extensive experimental results show that
CamoNet
can effectively run on various edge devices. Compared with the SOTA model adaptation approaches,
CamoNet
achieves significant accuracy improvement by 25.2% on average for image classification, 10.1% on average for object detection, and reduces the training memory by 4.8-11.4×. We will open-source our models and tools for edge AI developers.
更多查看译文
关键词
Contrastive learning,mobile-edge computing,neural architecture search,on-device training
AI 理解论文
溯源树
样例
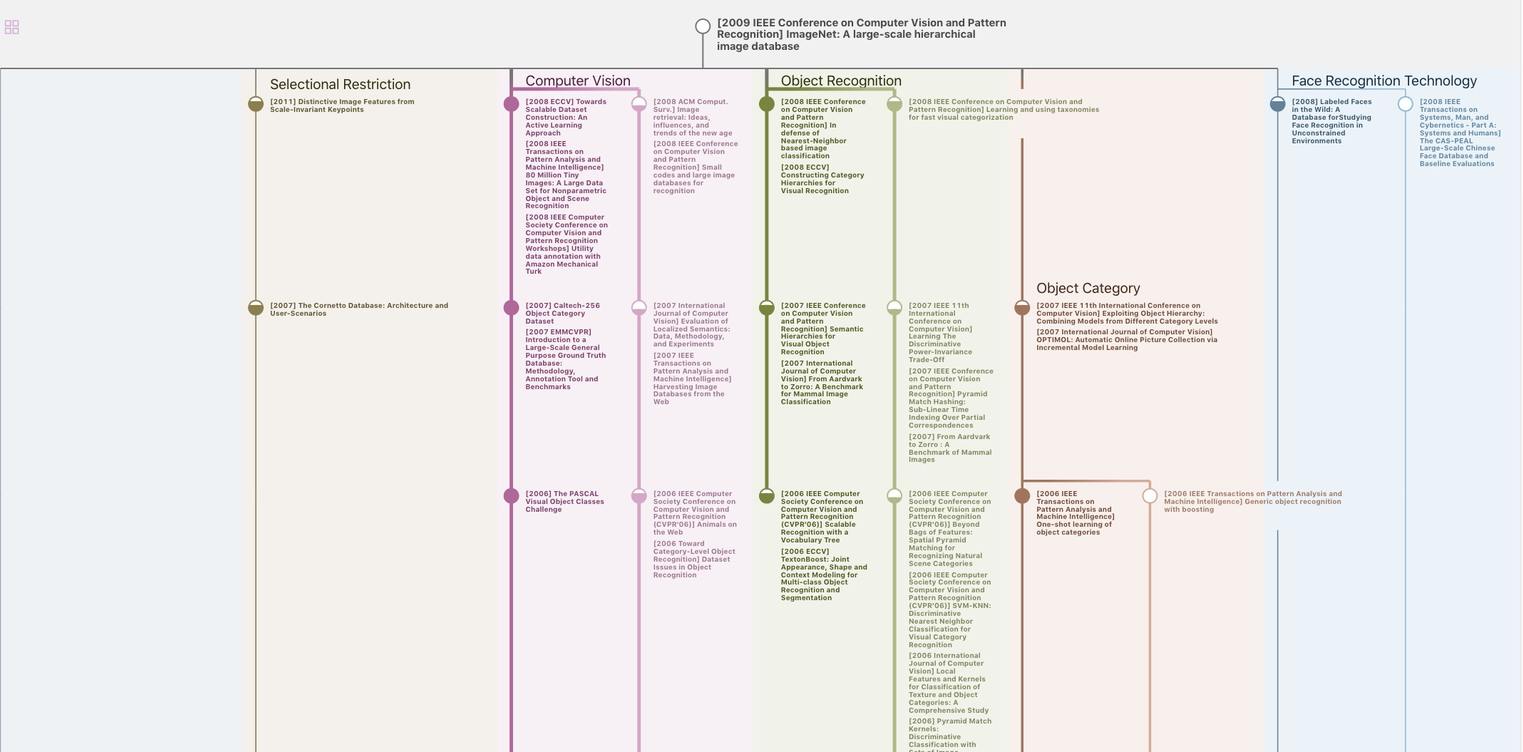
生成溯源树,研究论文发展脉络
Chat Paper
正在生成论文摘要