Applications of Bayesian networks in assessing the effects of family resilience on caregiver behavioral problems, depressive symptoms, and burdens
Journal of Computational Social Science(2024)
摘要
Family caregiving often leads to increased stress and anxiety due to intensive support in daily living and medical tasks. Traditionally, determining appropriate interventions has been a challenging task, given the need to conduct extended cohort studies with a restricted range of interventions, which proves to be time-consuming and costly. Moreover, the relationships between the results and the interventions often remain ambiguous, as the experiments lack complete control. In this study, we propose the use of Bayesian networks as a novel methodology for evaluating the impacts of hypothetical interventions aimed at reducing the burdens borne by family caregivers. This innovative approach demands only a single comprehensive survey measuring various aspects of family caregiver dynamics; specifically, family resilience, and caregiver behavioral problems, depressive symptoms, and burdens. Bayesian networks enable us to discern the relationships between these aspects, assess the strength of the causality, and evaluate the effects of interventions. Because all variables are modeled contemporaneously within the network, this approach allows us to control variables freely by applying hypothetical interventions, compared with traditional methods. Our results suggest fostering social interactions and enhancing family resilience as effective strategies by offering a number of social events, fitness and creative classes, and workshops. Other strategies that showed a positive impact included providing counseling to enhance family resilience, along with offering the caregivers medical assistance pertaining to their mental health, especially for depression and anxiety. Providing religious advice services also appeared to contribute to alleviating those symptoms and associated stress.
更多查看译文
关键词
Causality,Depression,Directed acyclic graph,Graphcial model,Hypothetical interventions
AI 理解论文
溯源树
样例
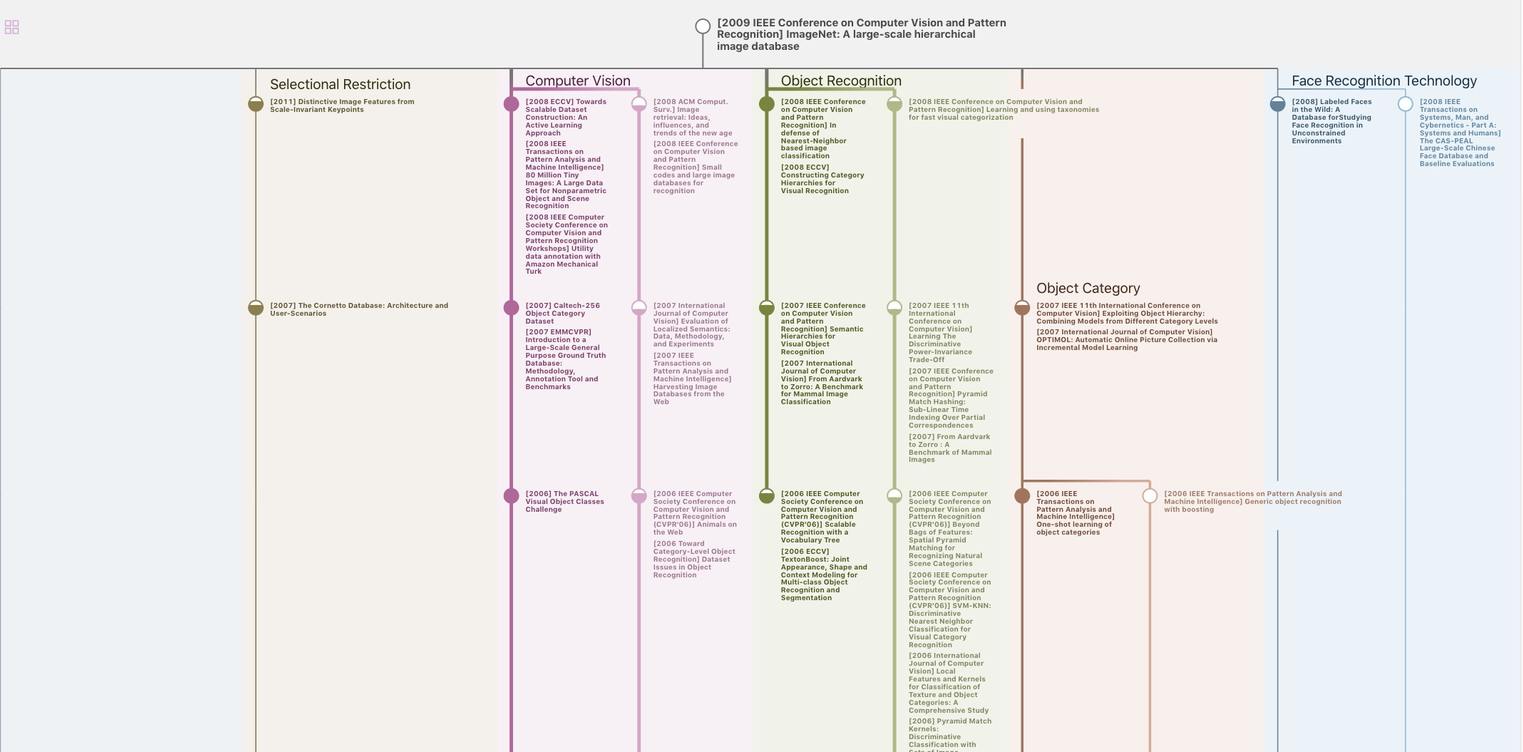
生成溯源树,研究论文发展脉络
Chat Paper
正在生成论文摘要