Potential factors-embedding group recommendation for online education
Discover Computing(2024)
摘要
Online education platform urgently needs recommendation methods to service learning groups. The existing group recommendation methods rely on member preference aggregation. However, potential factors such as teachers and schools have an important impact on the decision-making of learning groups. Due to the different ways in which potential factors operate, it is very challenging to model the impact these potential factors have on groups. In this paper, we propose a group recommendation method for online education (GEMst) that innovatively designs two modules to capture different types of potential factor embedding. In the subjective guidance module, we construct a heterogeneous information network based on the relationship between guiders (teacher, leader, etc.) and groups, analyze the influence of the guider on the group, and obtain the group representation from this perspective. In the objective environment hypergraph module, we consider the influence mode of the environment (school, platform, etc.) on the group, and propose the objective relationship hypergraph convolution. Compared with hypergraph convolution, it directly learns the influence between groups in the same environment. We provide information fusion strategies that GEMst can collaboratively consider group members’ opinions and the impact of potential factors. In addition, to enhance the learning effect of the model, we design a pre-training strategy based on the user grouping relationship that obtains more accurate user embedding representations. We test GEMst on two real-world datasets, and the results show that GEMst outperforms the current baseline group recommendation method.
更多查看译文
关键词
Group recommendation,Online education services,Pre-trained model,Potential factors
AI 理解论文
溯源树
样例
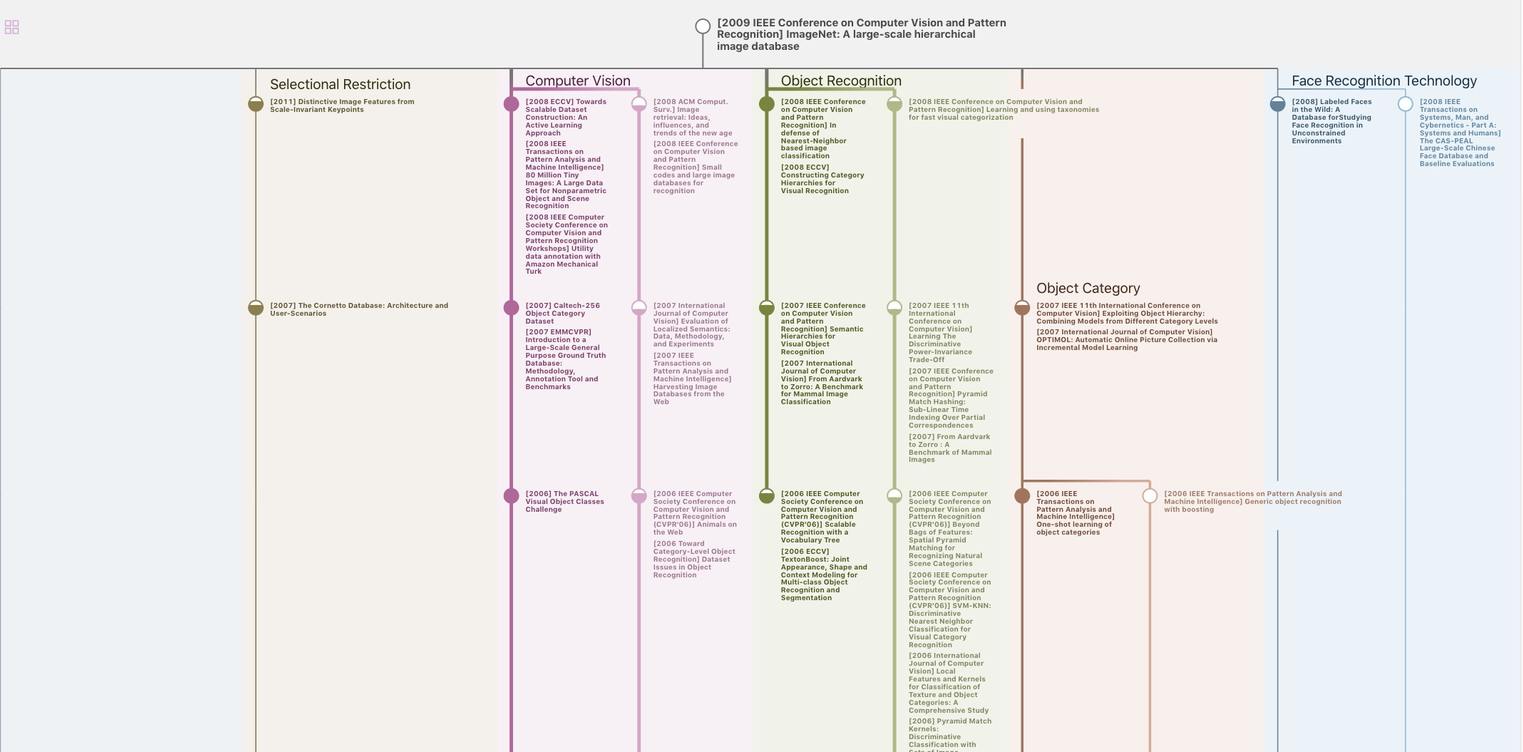
生成溯源树,研究论文发展脉络
Chat Paper
正在生成论文摘要