Enhancing 2D-3D Facial Recognition Accuracy of Truncated-Hiden Faces Using Fused Multi-Model Biometric Deep Features
Multimedia Tools and Applications(2024)
摘要
Facial recognition based on truncated and obscured data is a challenging topic for various issues in computer vision and biometrics. It allows to determine features that truncate or obscure a face based on synchronous or asynchronous facial changes. The issue in facial recognition is how to develop robust algorithms capable of solving various problems related to information flow in images. In this paper, we propose a novel distinct model for 2D and 3D facial recognition based on deep learning, called HResAlex-Net. The proposed model is a hybrid Convolutional Neural Network (CNN) architecture designed for face identification by leveraging multimodal biometric feature fusion. The aim is to enhance the recognition system’s performance. The proposed approach applies feature-level fusion, combining elements from both the ResNet and AlexNet CNN structures. Our novel approach synergistically combines the strengths of AlexNet and ResNet-50 architectures, thereby amplifying their individual advantages while concurrently minimizing the overall computational complexity. The proposed method was assessed on 2D and 3D data. A brand-new 2D YaleFace dataset that includes hidden truncated images, asynchronous face changes, brightness level changes, and lighting condition changes (center light, left light, right light), was generated. Other complex and challenging 2D/3D databases in terms of variations in facial expressions, position, and asynchronous variations, were also used. The experiments conducted on blurred, transcribed, and masked image types, have shown that the proposed method allows achieving high recognition rates of up to 98.31
更多查看译文
关键词
2D-3D facial recognition,Deep learning,Convolutional neural network,Feature level fusion
AI 理解论文
溯源树
样例
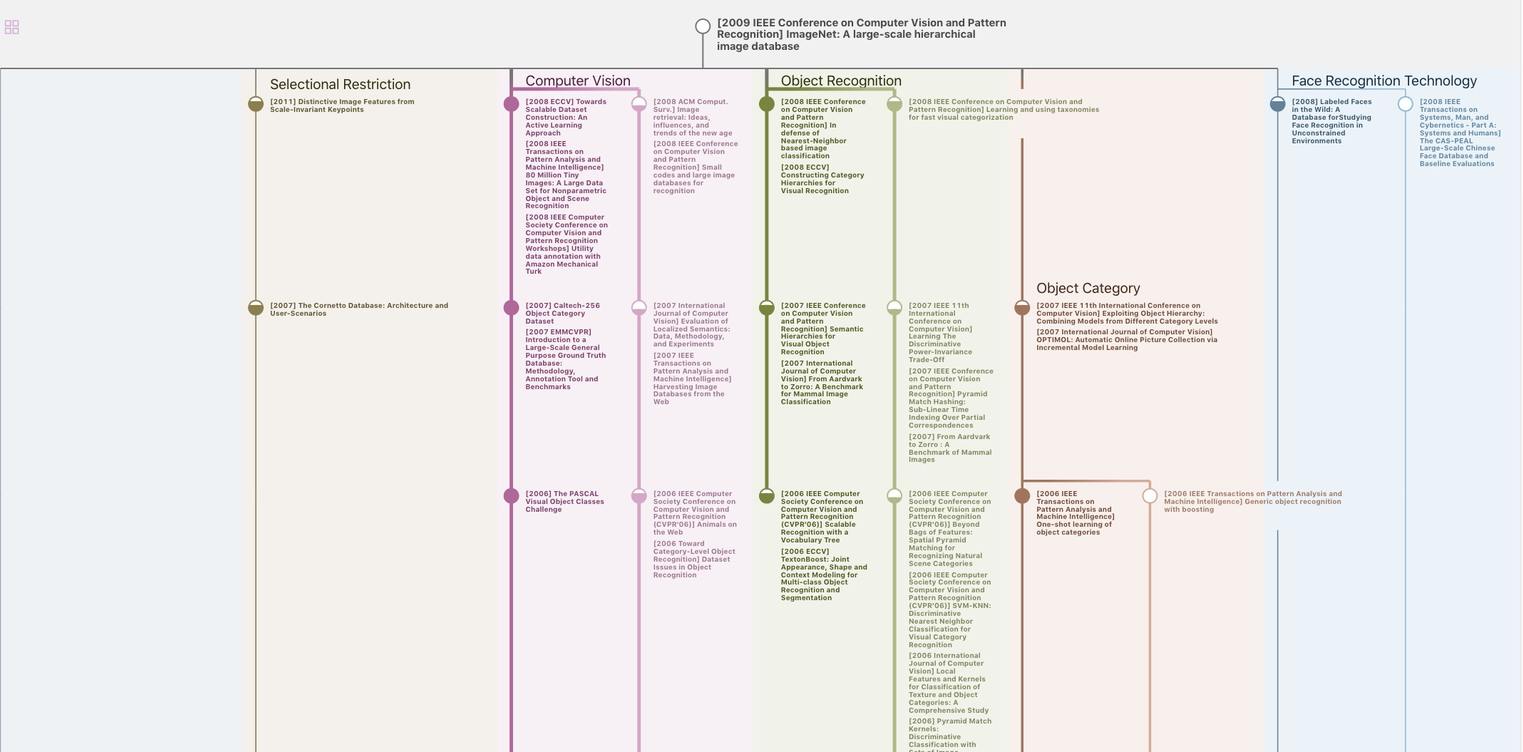
生成溯源树,研究论文发展脉络
Chat Paper
正在生成论文摘要