Generating and encouraging: An effective framework for solving class imbalance in multimodal emotion recognition conversation
Engineering Applications of Artificial Intelligence(2024)
摘要
In recent years, Intelligent Personal Assistants (IPAs) have emerged as important tools in human–computer interaction, with a wide range of applications such as voice assistant, virtual customer service, and navigation. Capturing and understanding the prominent emotional needs of users is important for improving the quality of service of IPAs. Multimodal emotion recognition in conversation (MMERC) aimed at automatically identifying and tracking the emotional states of speakers during the dialogue process has become a crucial component for building emotional IPAs and attracted increasing attention. Current research in this field is based on graph simulation for cross-modal and single-modal interactions. However, these methods ignore the highly imbalanced class problem inherent in MMERC, leading to a decrease in the generalization ability of the model and an inability to effectively recognize minority emotion classes. Data mining methods use oversampling to solve the imbalanced classification, but they are unsuitable for MMERC as they disrupt the conversational coherence and modality alignment characteristics of multimodal emotion recognition datasets. To overcome these problems, this paper proposes an IMBA-MMERC, which is an effective framework to address the pervasive issue of class imbalance in MMERC. Within this framework, sample generation for multimodal conversation tackles the application challenges that exist in multimodal conversational emotion recognition datasets, and well-classified encouraging loss mitigates the performance degradation of the model on certain majority classes due to decision boundary deviations. On two English benchmark datasets and one Chinese public dataset, we used two performance indicators to demonstrate the effectiveness and superiority of the proposed IMBA-MMERC. Ablation experiment, case study, and histograms visualization further verify the well performance of the proposed framework.
更多查看译文
关键词
Multimodal emotion recognition in conversation,Graph convolutional network,Sample generation,Well-classified encouraging loss
AI 理解论文
溯源树
样例
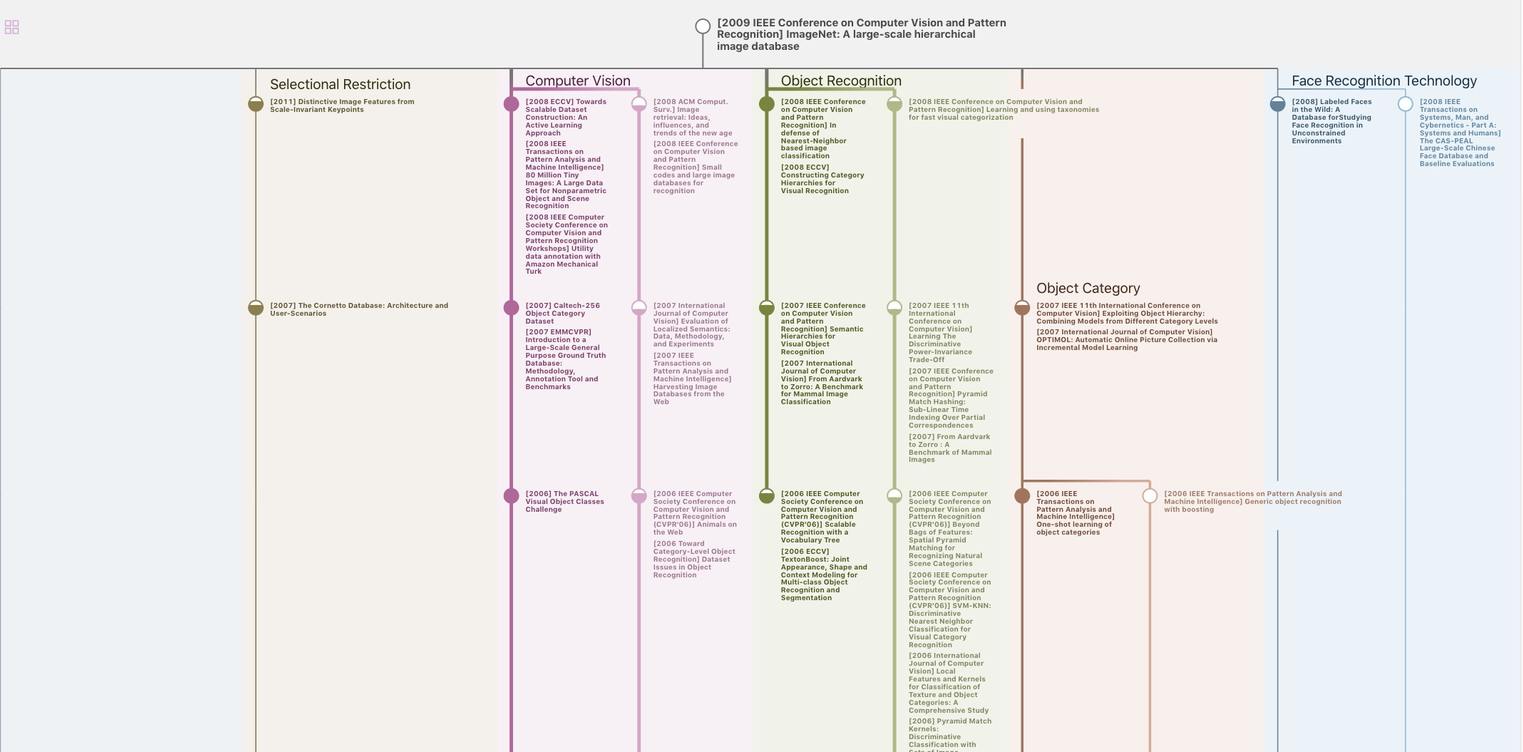
生成溯源树,研究论文发展脉络
Chat Paper
正在生成论文摘要