Online energy management strategy for ammonia-hydrogen hybrid electric vehicles harnessing deep reinforcement learning
Energy(2024)
摘要
Powertrain electrification and fuel decarbonization play pivotal roles in the pursuit of carbon peak and carbon neutrality within the realm of transportation. Ammonia and hydrogen are essential carbon-neutral fuels suited for deployment in heavy-duty, long-haul transportation applications. The technological complementarity of ammonia and hydrogen can be realized in the current context by combining various energy conversion devices. Energy management strategy (EMS) is one of the critical technologies in hybrid systems. In this study, a novel EMS based on deep reinforcement learning (DRL) is proposed for a heavy-duty automotive hybrid system containing an ammonia-hydrogen internal combustion engine (ICE), a fuel cell system (FCS), an ammonia electrolysis cell (AEC), and Li-ion batteries (LB). To address the protracted training times and convergence challenges intrinsic to DRL, a fuzzy logic control (FLC) is introduced to provide expert demonstrations during the DRL agent's learning process. The incorporation of FLC not only facilitates the convergence speed of the DRL algorithm, but also strikes a good balance between the operation efficiency improvement and battery SOC maintenance. The simulation results show that the proposed EMS improves average efficiency by 2% with a modest 0.75% SOC reduction across four operational scenarios compared to the FLC-based EMS. After thorough validation of the proposed EMS, a parametric study is conducted to examine three key parameters: charge mode threshold, FCS power change rate limit, and hybrid mode threshold. The study aims to offer valuable insights for formulating the EMS of the ammonia-hydrogen hybrid system.
更多查看译文
关键词
Energy management strategy,ammonia-hydrogen,hybrid powertrain,deep reinforcement learning,TD3
AI 理解论文
溯源树
样例
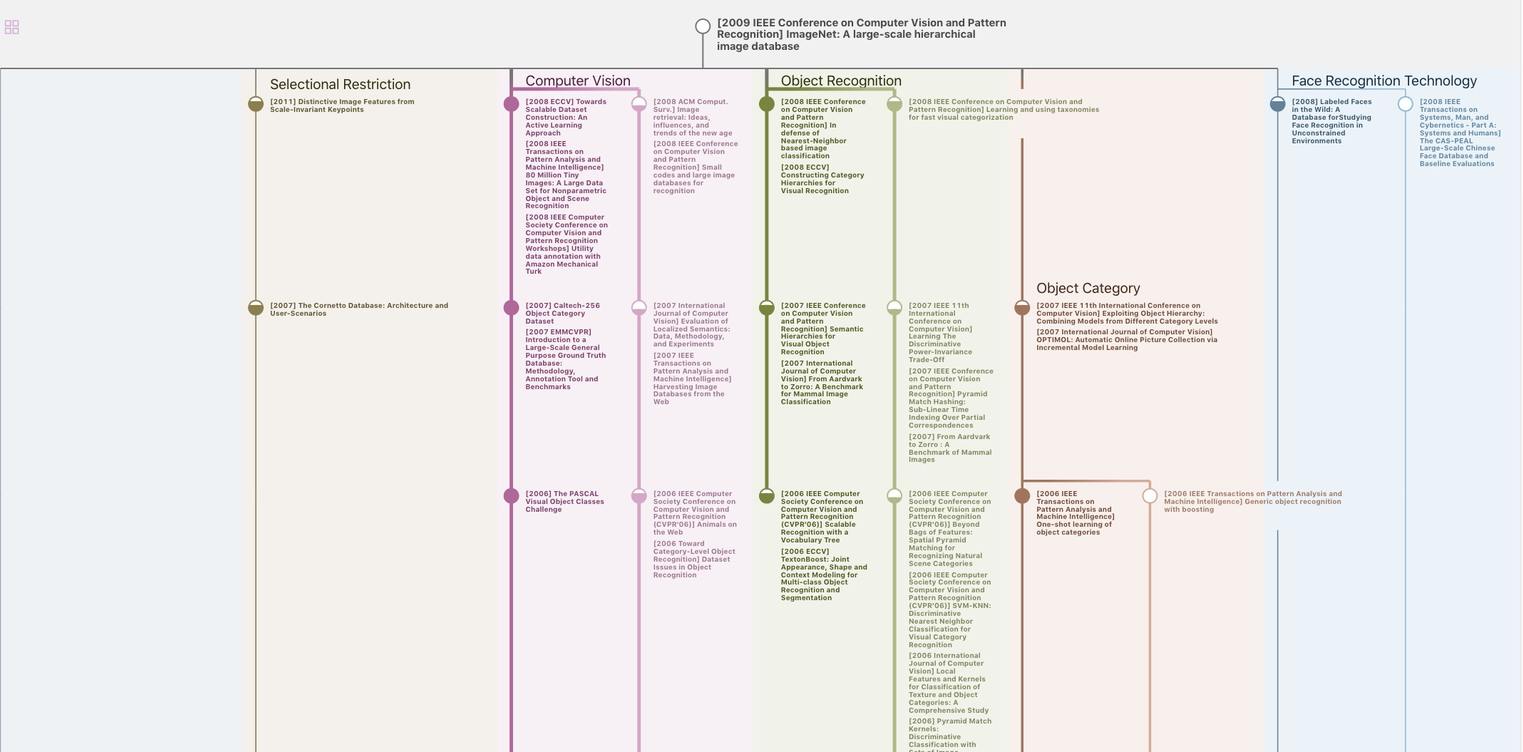
生成溯源树,研究论文发展脉络
Chat Paper
正在生成论文摘要