A CNN-LSTM-based Dual-task Neural Network for Power Operator Activity Detection
2023 International Conference on Advances in Electrical Engineering and Computer Applications (AEECA)(2023)
摘要
Human Activity Recognition (HAR) technology has been created to support the intelligent development of the operator activity detection system under electric power scenarios. However, there are still some challenges: 1) the existing algorithms primarily target simple activities, while having insufficient researches on complex activities; 2) the existing complex activity recognition algorithms do not fully utilize the temporal features of the data over a long time and the recognition accuracy is not high. Therefore, in order to better identify the complex human activities in electric power scenarios, which are composed of simple activities according to the temporal order rule, a CNN-LSTM-based dual-task neural network model is proposed in this paper to divide the recognition task into a CNN-based simple activity recognition task and a LSTM-based complex activity recognition task. The model completely integrates channel features with temporal features and uses different scale window segmentation techniques to account for the periodic differences between simple and complex activities. Additionally, the experiments of the CNN-LSTM-based dual-task neural network is conducted on seven types of simple activities and five types of complex activities in electric power scenarios. The experimental results show that the suggested model has an average accuracy of 93% on the test sets, which is 8% and 13% higher than the average accuracy of the CNN and LSTM models, respectively.
更多查看译文
关键词
human activity recognition,deep learning,wearable sensors,electric power system
AI 理解论文
溯源树
样例
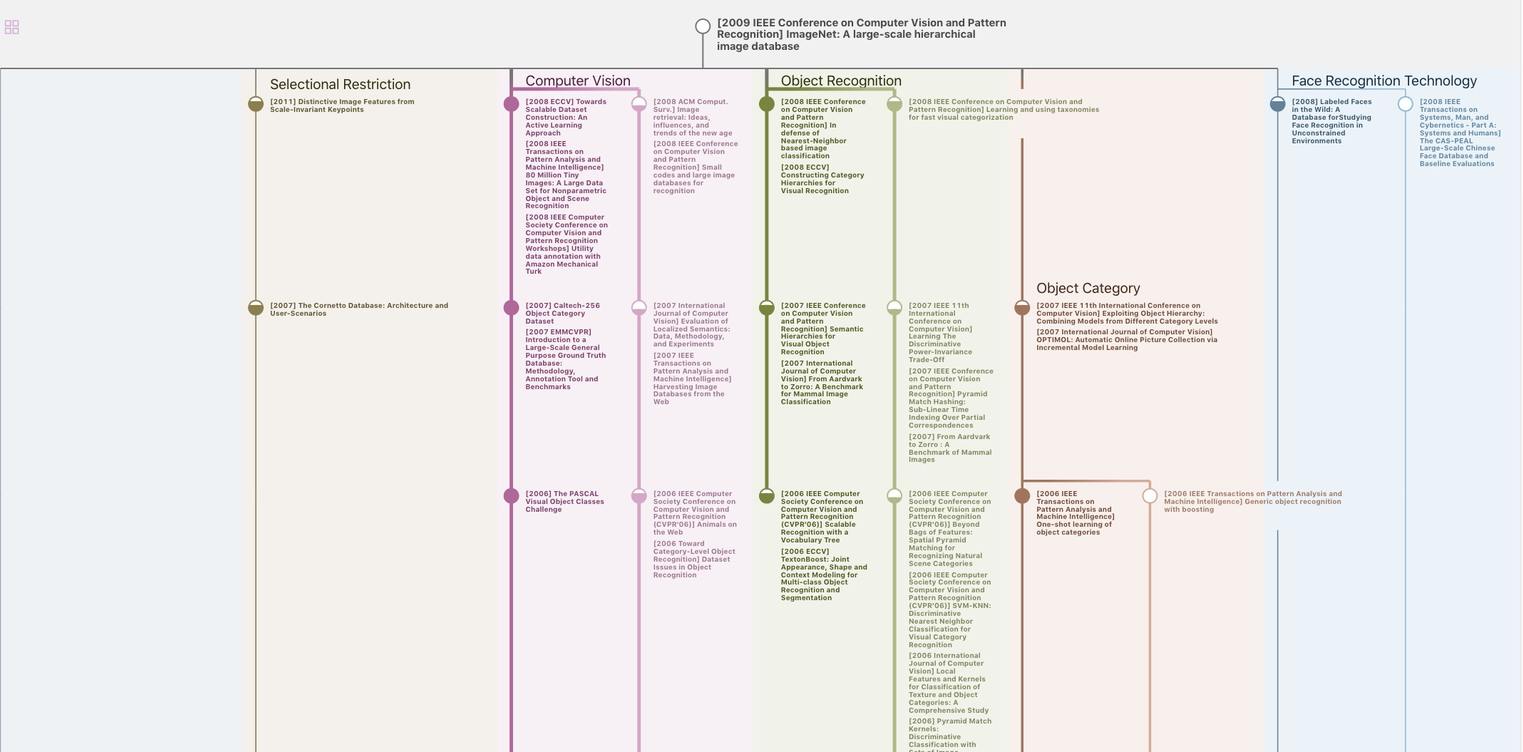
生成溯源树,研究论文发展脉络
Chat Paper
正在生成论文摘要