Degradation adaptive energy management strategy for FCHEV based on the Rule-DDPG method: tailored to the current SOH of the powertrain
IEEE Transactions on Transportation Electrification(2024)
摘要
To develop an energy management strategy (EMS) that adapts to the state-of-health (SOH) of the fuel cell stack (FCS) to keep the output of the powertrain of fuel cell hybrid electric vehicles optimal all the time, a degradation adaptive EMS is proposed. The proposed EMS is based on a hierarchical framework, where the upper layer involves a degradation rate model to estimate the SOH of the FCS online and acquire performance characteristics at different SOH levels. In the lower layer, an EMS using the Rule-DDPG algorithm is developed. The results prove that the proposed method outperforms existing mainstream EMSs and aligns closely with the dynamic programming approach regarding economic and durability indicators. Compared to non-adaptive EMSs across various driving cycles, the proposed degradation adaptive EMS can consistently achieve the lowest energy consumption and optimal durability performance, tailored to the current SOH of the powertrain. In addition, a comparison between offline simulation and hard-in-the-loop testing validates the computational efficiency and real-time applicability of the proposed strategy in practical environments. Specifically, the calculation time for each sample during the WLTC cycle is shown to be less than 213.214ms, with an average calculation time of 168.637ms and a minimum calculation time of 150.618ms.
更多查看译文
关键词
Fuel cell hybrid electric vehicle,Energy management strategy,Deep reinforcement learning,Fuel cell durability,Degradation adaption
AI 理解论文
溯源树
样例
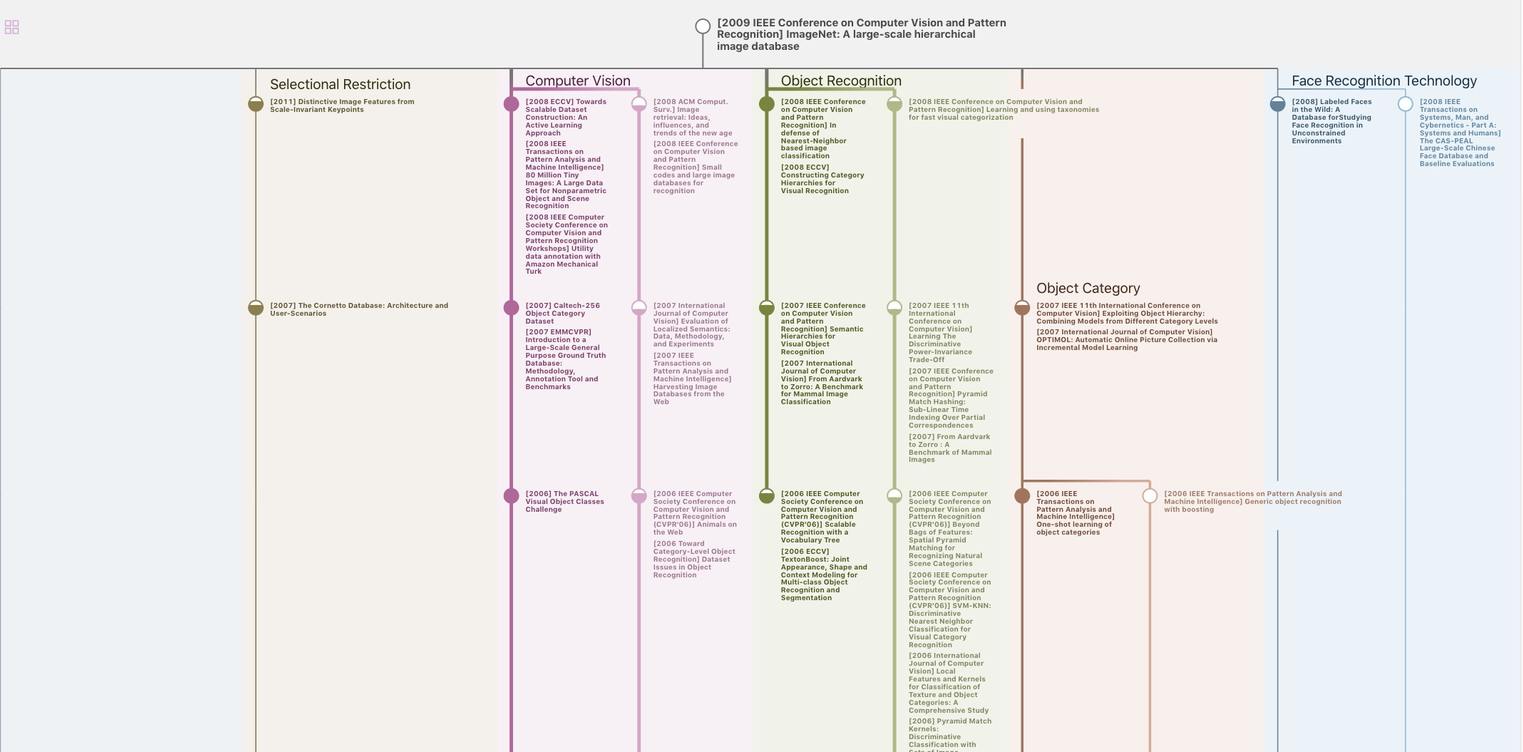
生成溯源树,研究论文发展脉络
Chat Paper
正在生成论文摘要