How Inverse Conditional Flows Can Serve as a Substitute for Distributional Regression
CoRR(2024)
Abstract
Neural network representations of simple models, such as linear regression,
are being studied increasingly to better understand the underlying principles
of deep learning algorithms. However, neural representations of distributional
regression models, such as the Cox model, have received little attention so
far. We close this gap by proposing a framework for distributional regression
using inverse flow transformations (DRIFT), which includes neural
representations of the aforementioned models. We empirically demonstrate that
the neural representations of models in DRIFT can serve as a substitute for
their classical statistical counterparts in several applications involving
continuous, ordered, time-series, and survival outcomes. We confirm that models
in DRIFT empirically match the performance of several statistical methods in
terms of estimation of partial effects, prediction, and aleatoric uncertainty
quantification. DRIFT covers both interpretable statistical models and flexible
neural networks opening up new avenues in both statistical modeling and deep
learning.
MoreTranslated text
AI Read Science
Must-Reading Tree
Example
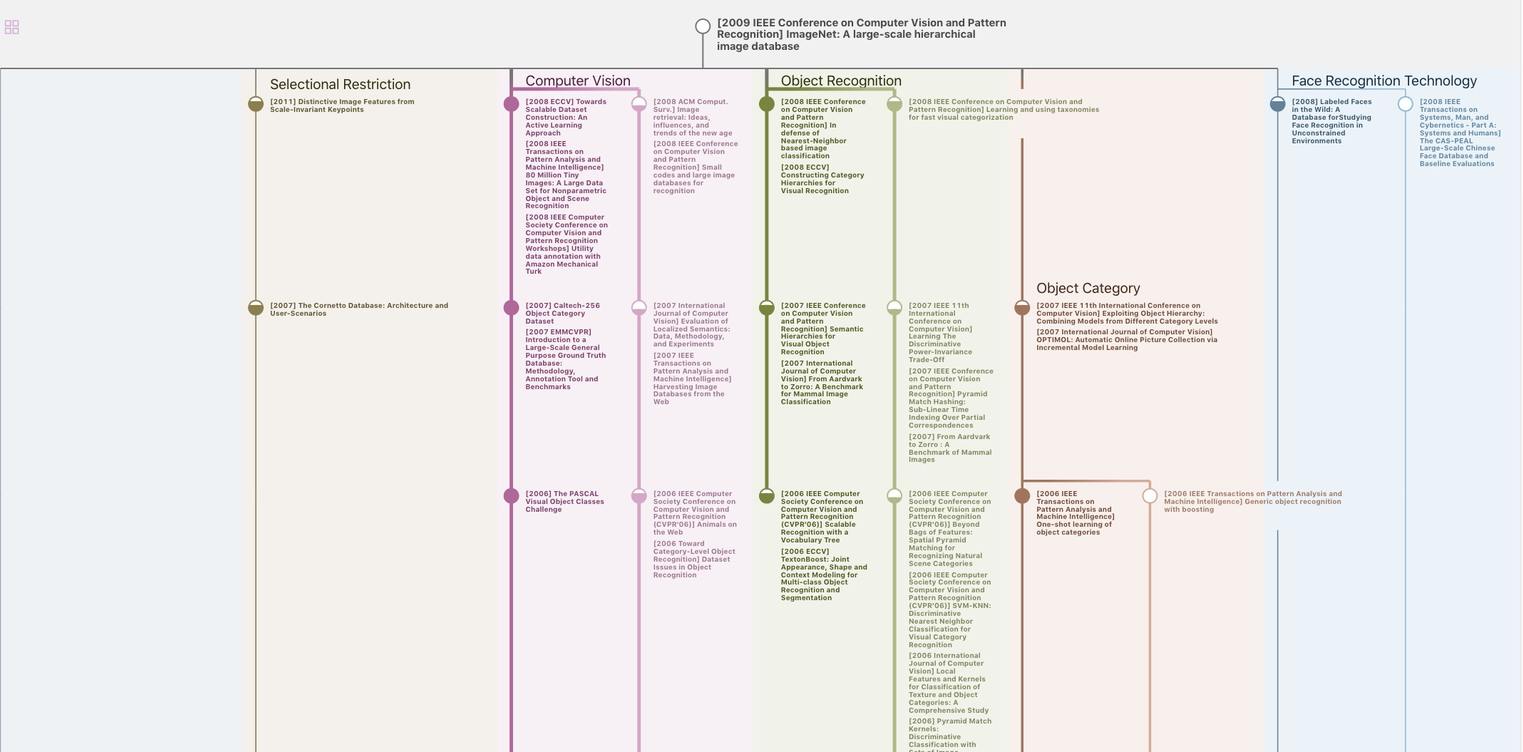
Generate MRT to find the research sequence of this paper
Chat Paper
Summary is being generated by the instructions you defined