Cross-Care: Assessing the Healthcare Implications of Pre-training Data on Language Model Bias
arxiv(2024)
摘要
Large language models (LLMs) are increasingly essential in processing natural
languages, yet their application is frequently compromised by biases and
inaccuracies originating in their training data. In this study, we introduce
Cross-Care, the first benchmark framework dedicated to assessing biases and
real world knowledge in LLMs, specifically focusing on the representation of
disease prevalence across diverse demographic groups. We systematically
evaluate how demographic biases embedded in pre-training corpora like ThePile
influence the outputs of LLMs. We expose and quantify discrepancies by
juxtaposing these biases against actual disease prevalences in various U.S.
demographic groups. Our results highlight substantial misalignment between LLM
representation of disease prevalence and real disease prevalence rates across
demographic subgroups, indicating a pronounced risk of bias propagation and a
lack of real-world grounding for medical applications of LLMs. Furthermore, we
observe that various alignment methods minimally resolve inconsistencies in the
models' representation of disease prevalence across different languages. For
further exploration and analysis, we make all data and a data visualization
tool available at: www.crosscare.net.
更多查看译文
AI 理解论文
溯源树
样例
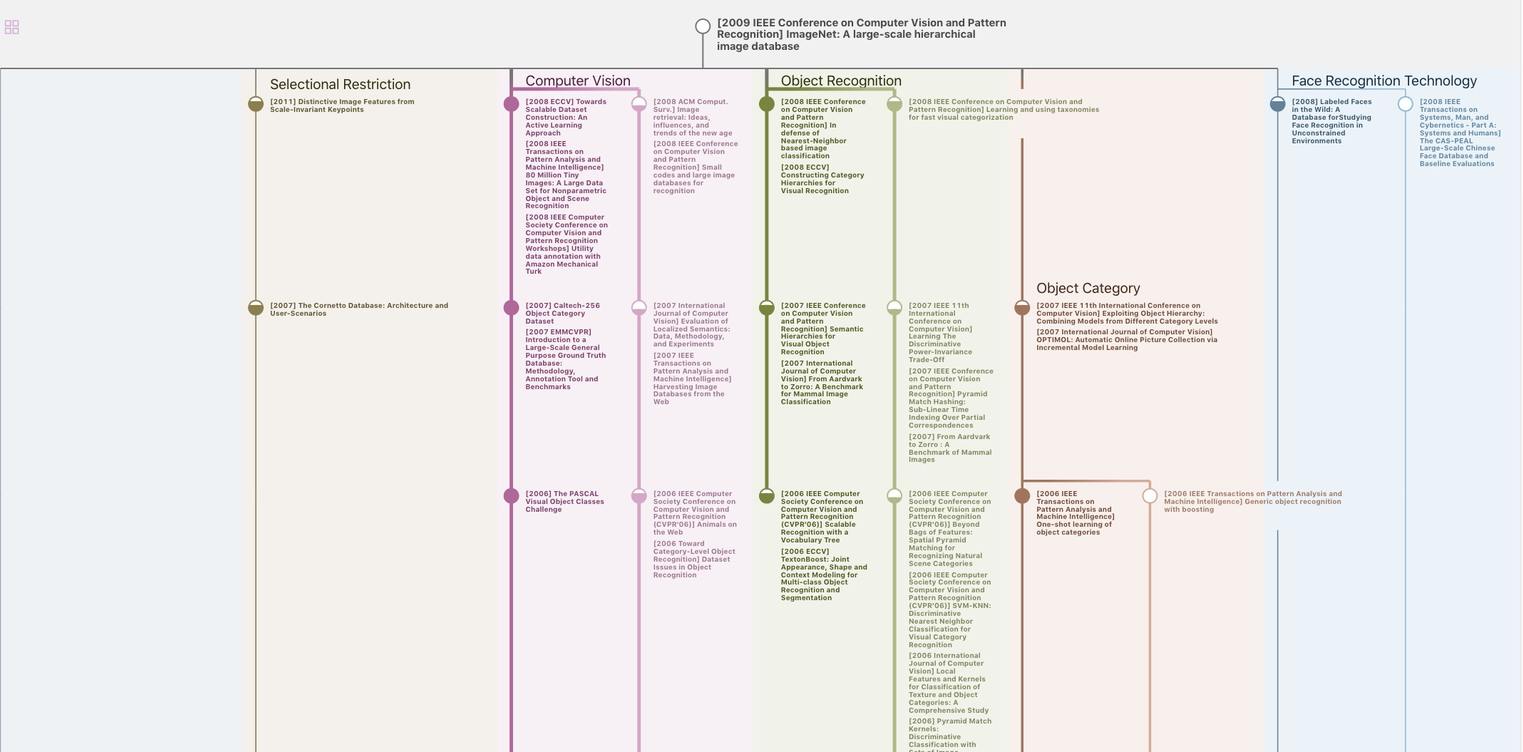
生成溯源树,研究论文发展脉络
Chat Paper
正在生成论文摘要