MRISegmentator-Abdomen: A Fully Automated Multi-Organ and Structure Segmentation Tool for T1-weighted Abdominal MRI
arxiv(2024)
摘要
Background: Segmentation of organs and structures in abdominal MRI is useful
for many clinical applications, such as disease diagnosis and radiotherapy.
Current approaches have focused on delineating a limited set of abdominal
structures (13 types). To date, there is no publicly available abdominal MRI
dataset with voxel-level annotations of multiple organs and structures.
Consequently, a segmentation tool for multi-structure segmentation is also
unavailable. Methods: We curated a T1-weighted abdominal MRI dataset consisting
of 195 patients who underwent imaging at National Institutes of Health (NIH)
Clinical Center. The dataset comprises of axial pre-contrast T1, arterial,
venous, and delayed phases for each patient, thereby amounting to a total of
780 series (69,248 2D slices). Each series contains voxel-level annotations of
62 abdominal organs and structures. A 3D nnUNet model, dubbed as
MRISegmentator-Abdomen (MRISegmentator in short), was trained on this dataset,
and evaluation was conducted on an internal test set and two large external
datasets: AMOS22 and Duke Liver. The predicted segmentations were compared
against the ground-truth using the Dice Similarity Coefficient (DSC) and
Normalized Surface Distance (NSD). Findings: MRISegmentator achieved an average
DSC of 0.861±0.170 and a NSD of 0.924±0.163 in the internal test set.
On the AMOS22 dataset, MRISegmentator attained an average DSC of
0.829±0.133 and a NSD of 0.908±0.067. For the Duke Liver dataset, an
average DSC of 0.933±0.015 and a NSD of 0.929±0.021 was obtained.
Interpretation: The proposed MRISegmentator provides automatic, accurate, and
robust segmentations of 62 organs and structures in T1-weighted abdominal MRI
sequences. The tool has the potential to accelerate research on various
clinical topics, such as abnormality detection, radiotherapy, disease
classification among others.
更多查看译文
AI 理解论文
溯源树
样例
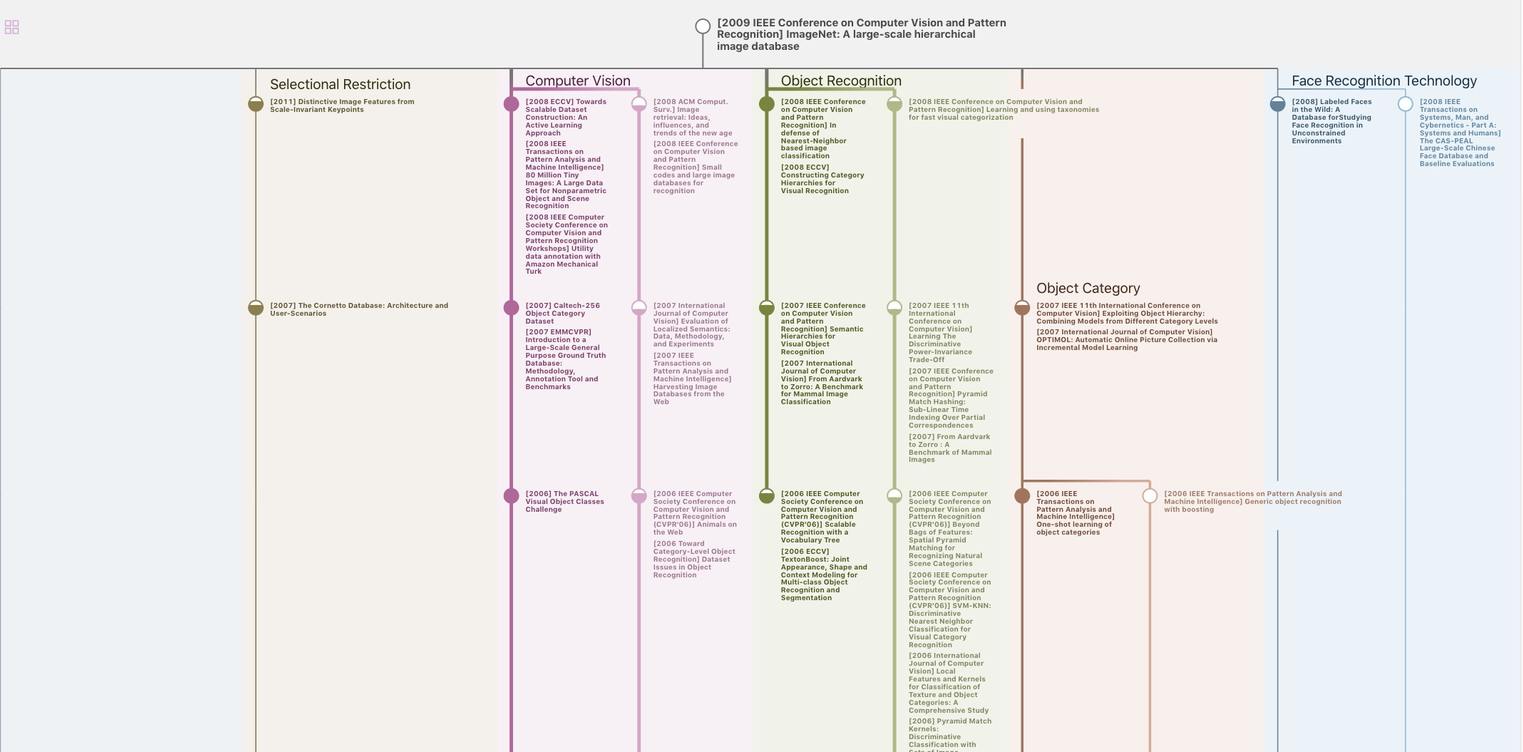
生成溯源树,研究论文发展脉络
Chat Paper
正在生成论文摘要