A Distributed Aggregation Approach for Vehicular Federated Learning
IEEE Access(2024)
摘要
Federated Learning (FL) has rapidly become a crucial paradigm for training Machine Learning (ML) models when datasets are spread across several devices without compromising the privacy of the data owners. In vehicular networks, FL can be used to train driving models and object detection and classification over sensitive datasets to continuously improve user experience and driving safety. However, the majority of FL implementations cannot efficiently filter malicious vehicular users and low-quality contributions. This article proposes Distributed OT-based Federated Learning (DOTFL), an aggregation mechanism based on the clustering of the received trained Neural Networks Neural Network (NN) at the vehicular devices and on outlier detection. The proposed mechanism can detect malicious contributions by comparing them to previously received contributions and following a clustering approach. Furthermore, the convergence time of the FL process is improved by distributing trained NN weights directly through vehicle-to-vehicle links. Experimental analysis shows an improvement of up to 22% in terms of accuracy compared to state-of-the-art FL approaches. This is achieved by using clustering models and removing outliers, enabling a significantly lower presence of malicious contributions in aggregated models.
更多查看译文
关键词
Federated Vehicular Networks,Robust Model Aggregation,Privacy Preservation,Vehicular Ad hoc Networks
AI 理解论文
溯源树
样例
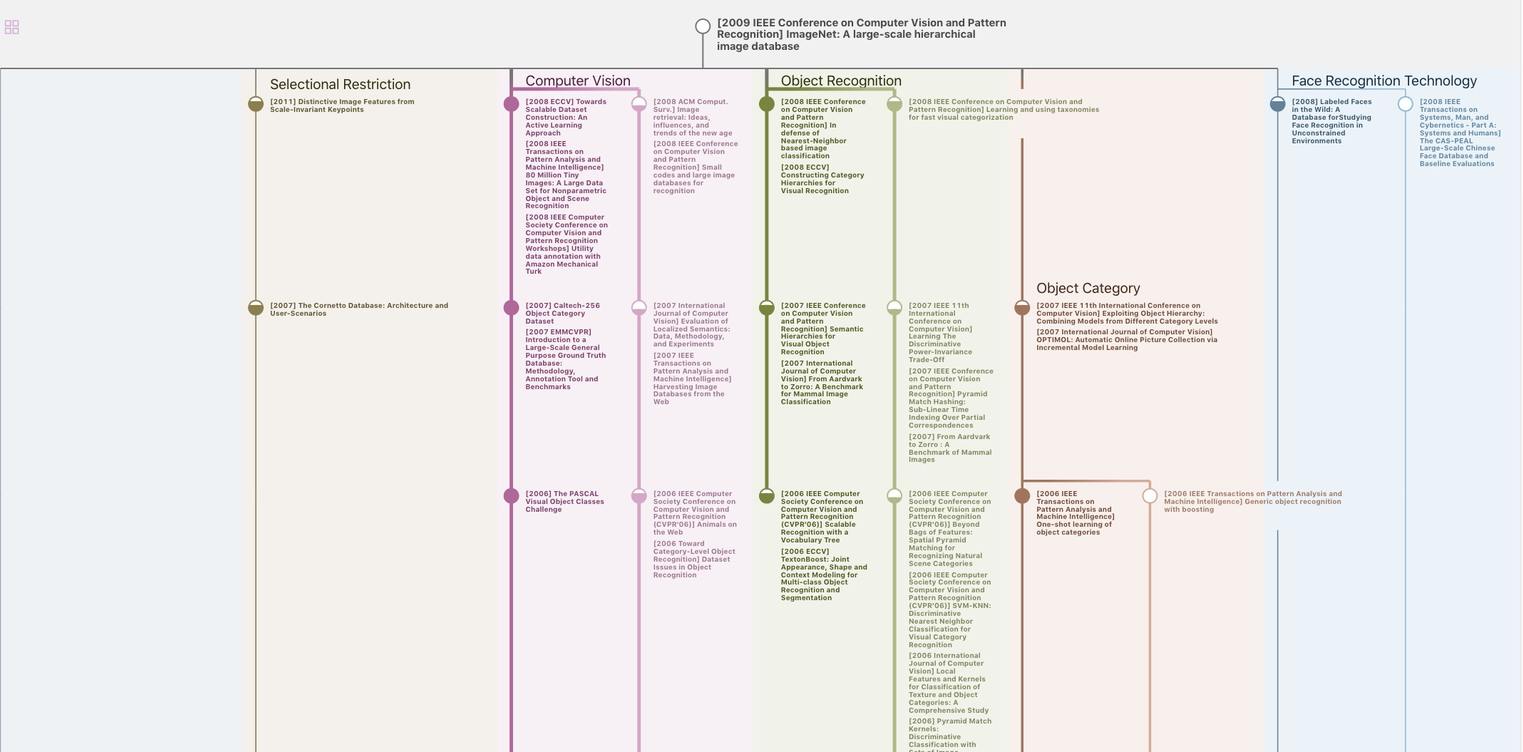
生成溯源树,研究论文发展脉络
Chat Paper
正在生成论文摘要