Learning an Augmented RGB Representation for Dynamic Hand Gesture Authentication
IEEE Transactions on Circuits and Systems for Video Technology(2024)
摘要
Dynamic hand gesture authentication aims to recognize users’ identity through the characteristics of their hand gestures. How to extract favorable features for verification is the key to success. Cross-modal knowledge distillation is an intuitive approach that can introduce additional modality information in the training phase to enhance the target modality representation, improving model performance without incurring additional computation in the inference phase. However, most previous cross-modal knowledge distillation methods directly transfer information from one modality to another one without considering the modality gap. In this paper, we propose a novel translation mechanism in cross-modal knowledge distillation that can effectively mitigate the modality gap and utilize the information from the additional modality to enhance the target modality representation. In order to better transfer modality information, we propose a novel modality fusion-enhanced non-local (MFENL) module, which can fuse the multi-modal information from the teacher network and enhance the fused features based on the modality input into the student network. We use cascaded MFENL modules as the translator based on the proposed cross-modal knowledge distillation method to learn an enhanced RGB representation for dynamic hand gesture authentication. Extensive experiments on the SCUT-DHGA dataset demonstrate that our method has compelling advantages over the state-of-the-art methods. The code is available at https://github.com/SCUT-BIP-Lab/TranslationCKD.
更多查看译文
关键词
Hand gesture authentication,Feature fusion,Augmented representation,Multi-modal information,Cross-modal knowledge distillation
AI 理解论文
溯源树
样例
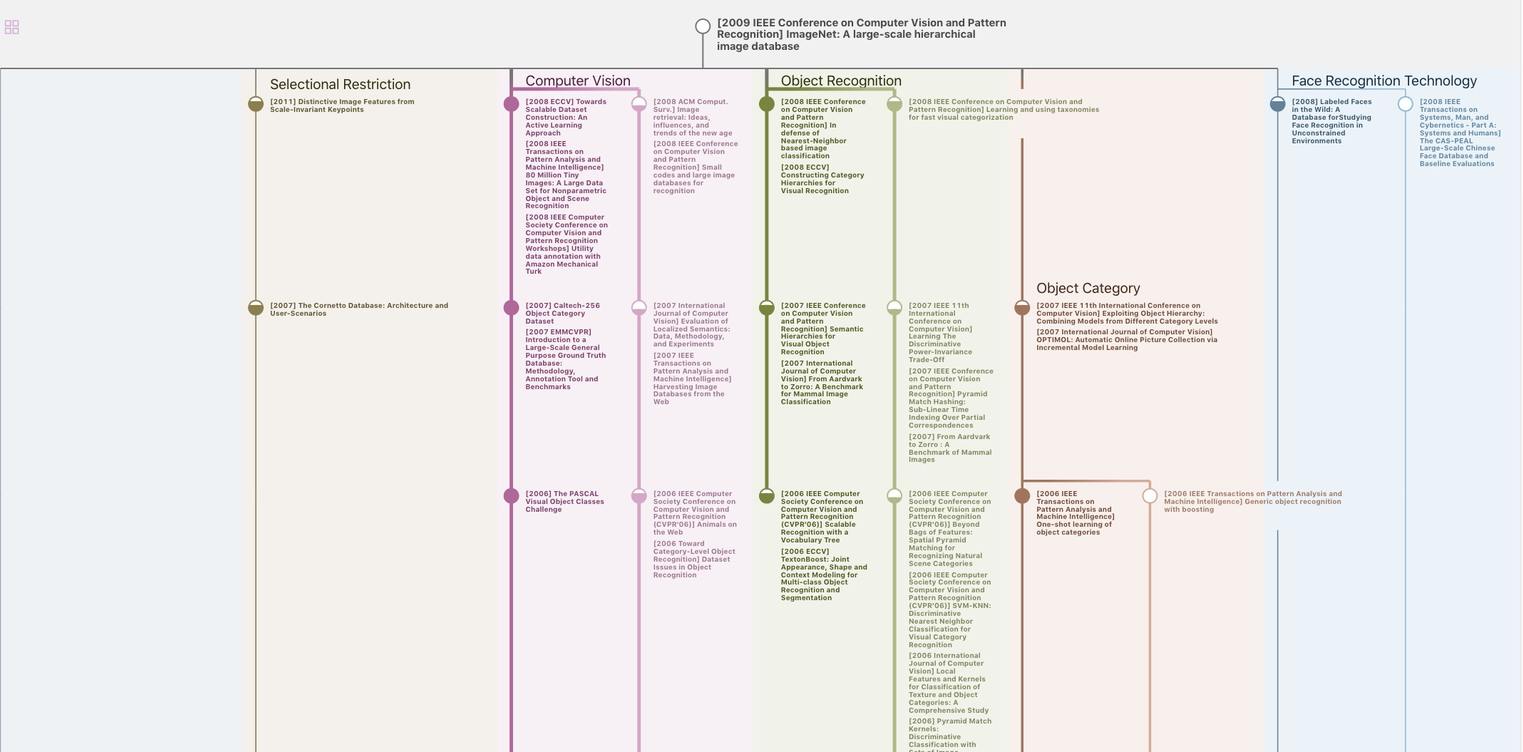
生成溯源树,研究论文发展脉络
Chat Paper
正在生成论文摘要