UVMO: Deep unsupervised visual reconstruction-based multimodal-assisted odometry
Pattern Recognition(2024)
摘要
In recent years, unsupervised visual odometry (VO) based on visual reconstruction has attracted lots of attention due to its end-to-end pose estimation approach and the advantage of not requiring real labels for training. Unsupervised VO inputs monocular video frames into a pose estimation network to output the predicted poses, and optimizes the pose prediction by minimizing visual reconstruction loss with epipolar geometry constraint. However, lack of depth information and complex environments such as rapid turns and uneven lighting in monocular video frames can result in insufficient visual information for pose estimation. Additionally, dynamic objects and discontinuous occlusions in monocular video frames can introduce inappropriate errors in visual reconstruction. In this paper, an Unsupervised V isual reconstruction-based Multimodal-assisted Odometry (UVMO) is proposed. UVMO leverages inertial and lidar information to complement visual information to acquire more accurate pose estimation. Specifically, a triple-modal fusion strategy called SMPF is proposed to conduct a more comprehensive and stable fusion of the three modalities’ data. Additionally, an image-based mask is introduced to filter out the dynamic occlusion regions in video frames, improving the accuracy of visual reconstruction. To the best of our knowledge, this paper is the first to propose a pure deep learning-based visual-inertial-lidar odometry. Experiments show that UVMO achieves state-of-the-art performance among pure deep learning-based unsupervised odometry.
更多查看译文
关键词
Visual odometry,Pose estimation,Visual reconstruction,Multimodal assisted,Triple-modal fusion,Image-based mask
AI 理解论文
溯源树
样例
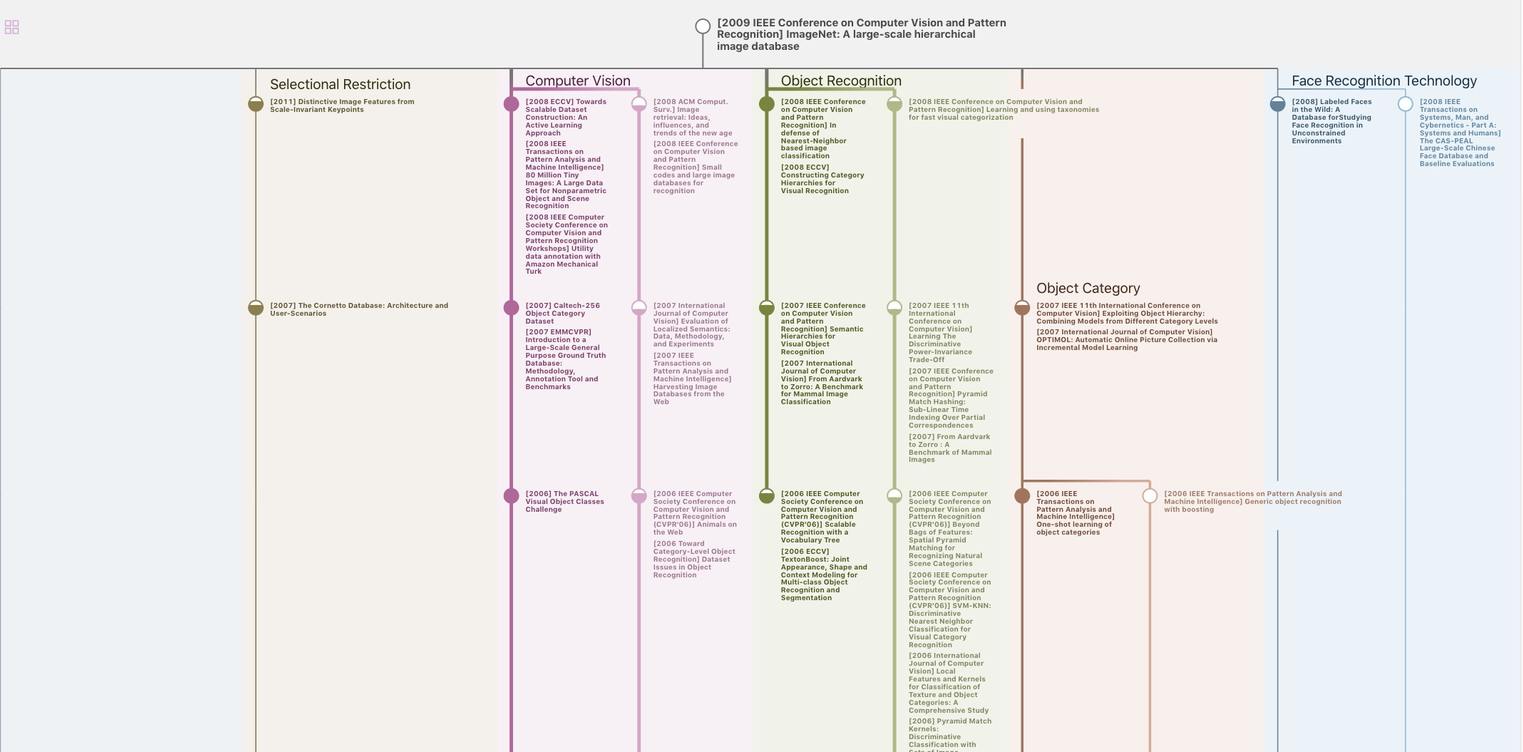
生成溯源树,研究论文发展脉络
Chat Paper
正在生成论文摘要