A Mimic-Filling Algorithm for Pairwise Model Discrimination of Censoring Lifetime Data
IEEE Transactions on Reliability(2024)
摘要
In the realm of pairwise lifetime model discrimination, it is a customary practice to frame it as a hypothesis test. In literature, generalized pivotal quantity (GPQ) emerges as an effective tool with complete observations, primarily owing to its advantages in addressing challenges posed by intricate parameter functions and limited sample size. In practical lifetime tests, the occurrence of censoring observations is not uncommon. Under this circumstance, the GPQ-based discrimination is infrequently employed primarily due to the inherent challenge of directly constructing the requisite GPQ. To tackle it, the present study first introduces an algorithm directly integrating data filling with GPQ. Then to mitigate the impact of data filling to GPQ, the generated samples from fiducial distribution also emulate the censoring and filling processes. This novel algorithm is thus designated as the “Mimic filling Algorithm.” For application purposes, this algorithm is applied to Type I censoring data, with the simulation study centered around widely encountered discrimination scenarios for Lognormal, Gamma, and Weibull distributions. In terms of two types errors, simulation results unequivocally demonstrate its superior performance compared to the direct integration of data filling with bootstrap, asymptotic normal approximation, and GPQ. Finally, this study applies the mimic-filling algorithm to discriminate two lithium-ion battery lifetime models with close-fitting results.
更多查看译文
关键词
Censoring data,generalized pivotal quantity (GPQ),hypothesis test,mimic-filling algorithm,pairwise model discrimination
AI 理解论文
溯源树
样例
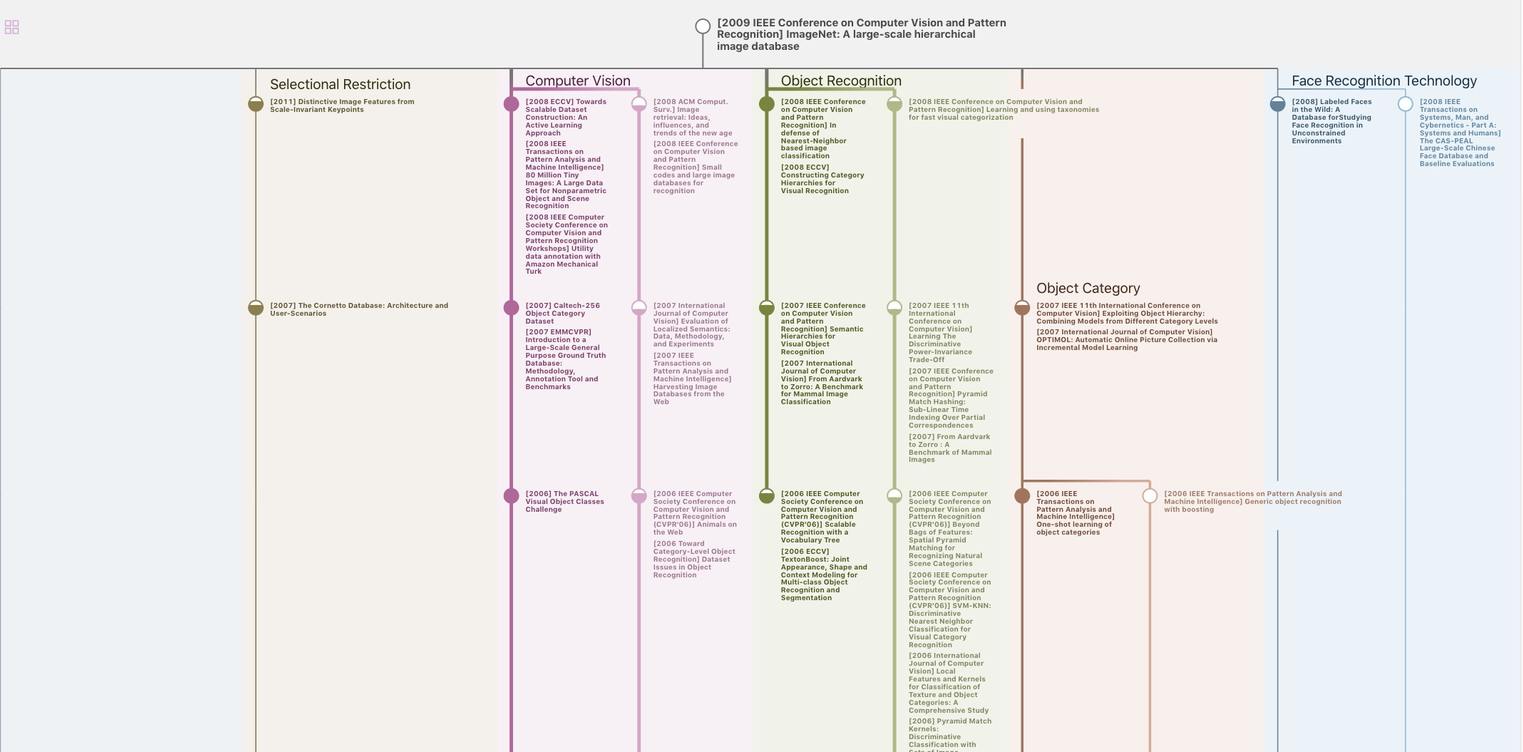
生成溯源树,研究论文发展脉络
Chat Paper
正在生成论文摘要