SimBU: Self-Similarity-Based Hybrid Binary-Unary Computing for Nonlinear Functions
IEEE TRANSACTIONS ON COMPUTERS(2024)
摘要
Unary computing is a relatively new method for implementing arbitrary nonlinear functions that uses unpacked, thermometer number encoding, enabling much lower hardware costs. In its original form, unary computing provides no trade-off between accuracy and hardware cost. In this work, we propose a novel self-similarity-based method to optimize the previous hybrid binary-unary work and provide it with the trade-off between accuracy and hardware cost by introducing controlled levels of approximation. Looking for self-similarity between different parts of a function allows us to implement a very small subset of core unique subfunctions and derive the rest of the subfunctions from this core using simple linear transformations. We compare our method to previous works such as FloPoCo-LUT (lookup table), HBU (hybrid binary-unary) and FloPoCo-PPA (piecewise polynomial approximation) on several 8–12-bit nonlinear functions including Log, Exp, Sigmoid, GELU, Sin, and Sqr, which are frequently used in neural networks and image processing applications. The area × delay hardware cost of our method is on average 32%–60% better than previous methods in both exact and approximate implementations. We also extend our method to multivariate nonlinear functions and show on average 78%–92% improvement over previous work.
更多查看译文
关键词
Hardware,Costs,Logic gates,Wires,Polynomials,Delays,Image coding,Hardware acceleration,approximate computing,unary computing,stochastic computing,table-based method,piecewise polynomial approximation,nonlinear function,activation function
AI 理解论文
溯源树
样例
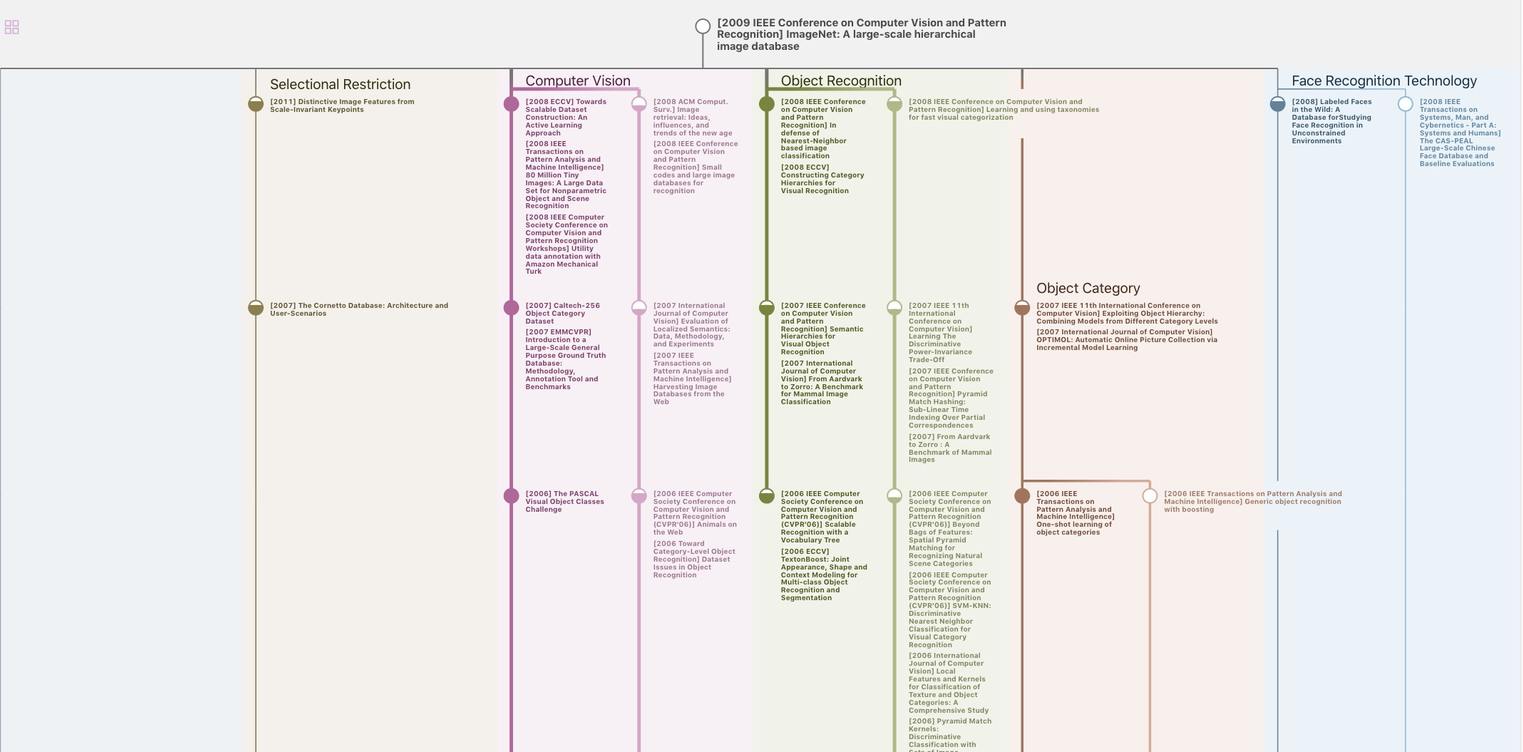
生成溯源树,研究论文发展脉络
Chat Paper
正在生成论文摘要