HELP! Providing Proactive Support in the Presence of Knowledge Asymmetry.
International Conference on Autonomous Agents and Multiagent Systems(2024)
Abstract
While the development of proactive personal assistants has been a popular topic within AI research, most research in this direction tends to focus on a small subset of possible interaction settings. An important setting that is often overlooked is one where the users may have an incomplete or incorrect understanding of the task. This could lead to the user following incorrect plans with potentially disastrous consequences. Supporting such settings requires agents that are able to detect when the user's actions might be leading them to a possibly undesirable state and, if they are, intervene so the user can correct their course of actions. For the former problem, we introduce a novel planning compilation that transforms the task of estimating the likelihood of task failures into a probabilistic goal recognition problem. This allows us to leverage the existing goal recognition techniques to detect the likelihood of failure. For the intervention problem, we use model search algorithms to detect the set of minimal model updates that could help users identify valid plans. These identified model updates become the basis for agent intervention. We further extend the proposed approach by developing methods for pre-emptive interventions, to prevent the users from performing actions that might result in eventual plan failure. We show how we can identify such intervention points by using an efficient approximation of the true intervention problems, which are best represented as a Partially Observable Markov Decision-Process (POMDP). To substantiate our claims and demonstrate the applicability of our methodology, we have conducted exhaustive evaluations across a diverse range of planning benchmarks. These tests have consistently shown the robustness and adaptability of our approach, further solidifying its potential utility in real-world applications.
MoreTranslated text
求助PDF
上传PDF
View via Publisher
AI Read Science
AI Summary
AI Summary is the key point extracted automatically understanding the full text of the paper, including the background, methods, results, conclusions, icons and other key content, so that you can get the outline of the paper at a glance.
Example
Background
Key content
Introduction
Methods
Results
Related work
Fund
Key content
- Pretraining has recently greatly promoted the development of natural language processing (NLP)
- We show that M6 outperforms the baselines in multimodal downstream tasks, and the large M6 with 10 parameters can reach a better performance
- We propose a method called M6 that is able to process information of multiple modalities and perform both single-modal and cross-modal understanding and generation
- The model is scaled to large model with 10 billion parameters with sophisticated deployment, and the 10 -parameter M6-large is the largest pretrained model in Chinese
- Experimental results show that our proposed M6 outperforms the baseline in a number of downstream tasks concerning both single modality and multiple modalities We will continue the pretraining of extremely large models by increasing data to explore the limit of its performance
Upload PDF to Generate Summary
Must-Reading Tree
Example
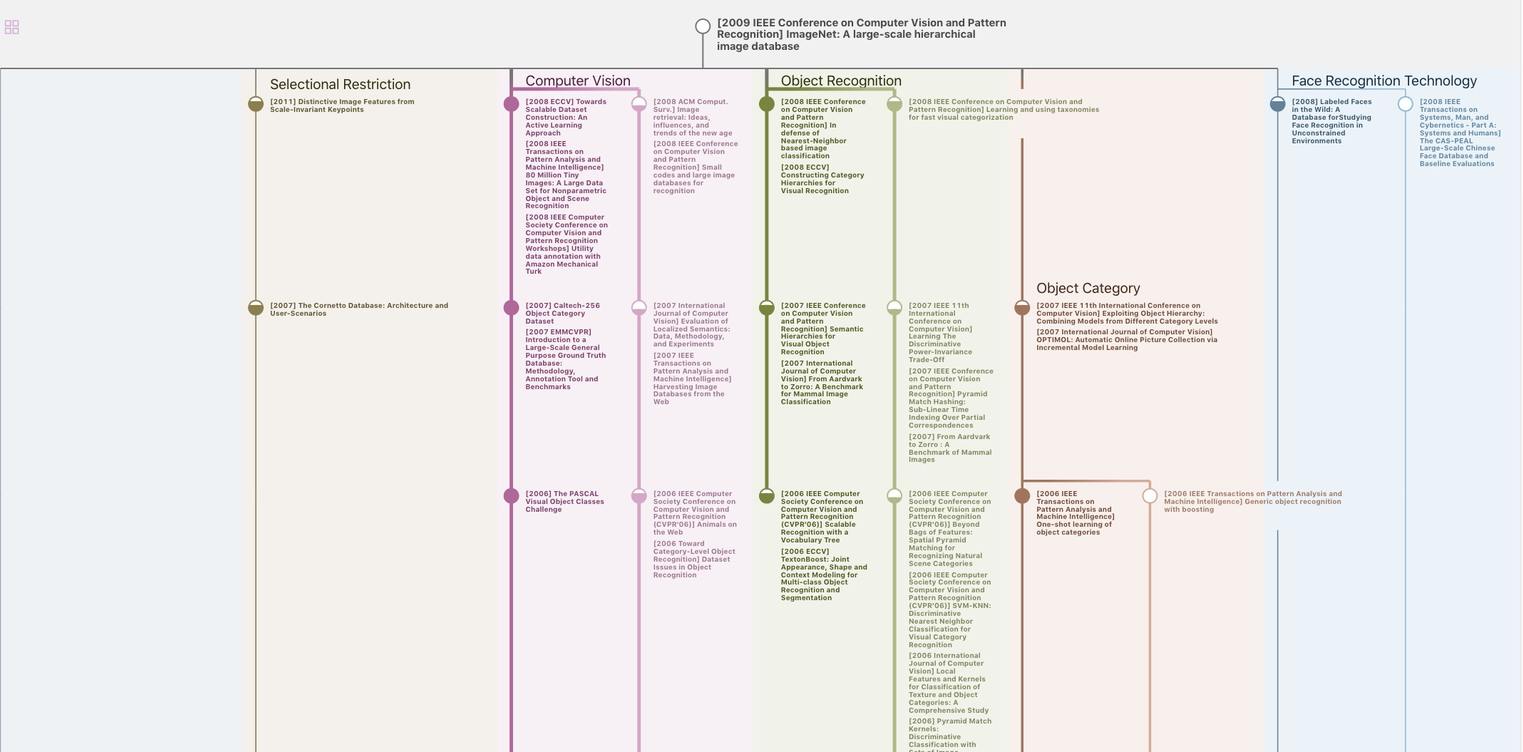
Generate MRT to find the research sequence of this paper
Related Papers
Data Disclaimer
The page data are from open Internet sources, cooperative publishers and automatic analysis results through AI technology. We do not make any commitments and guarantees for the validity, accuracy, correctness, reliability, completeness and timeliness of the page data. If you have any questions, please contact us by email: report@aminer.cn
Chat Paper
GPU is busy, summary generation fails
Rerequest