ANOTO: Improving Automated Negotiation Via Offline-to-Online Reinforcement Learning.
International Conference on Autonomous Agents and Multiagent Systems(2024)
Abstract
Automated negotiation is a crucial component for establishing cooperation and collaboration within multi-agent systems.While reinforcement learning (RL)-based negotiating agents have achieved remarkable success in various scenarios, they still face limitations due to certain assumptions on which they are based.In this work, we proposes a novel approach called ANOTO to improve the negotiating agents' ability via offline-to-online RL.ANOTO enables a negotiating agent (1) to communicate with opponents using an end-to-end strategy that covers all negotiation actions, (2) to learn negotiation strategies from historical offline data without requiring active interactions, and (3) to enhance the optimization process during the online phase, facilitating rapid and stable performance improvements for the learned offline strategies.Experimental results, based on a number of negotiation scenarios and recent winning agents from the Automated Negotiating Agents Competitions (ANAC), are provided.
MoreTranslated text
求助PDF
上传PDF
View via Publisher
AI Read Science
AI Summary
AI Summary is the key point extracted automatically understanding the full text of the paper, including the background, methods, results, conclusions, icons and other key content, so that you can get the outline of the paper at a glance.
Example
Background
Key content
Introduction
Methods
Results
Related work
Fund
Key content
- Pretraining has recently greatly promoted the development of natural language processing (NLP)
- We show that M6 outperforms the baselines in multimodal downstream tasks, and the large M6 with 10 parameters can reach a better performance
- We propose a method called M6 that is able to process information of multiple modalities and perform both single-modal and cross-modal understanding and generation
- The model is scaled to large model with 10 billion parameters with sophisticated deployment, and the 10 -parameter M6-large is the largest pretrained model in Chinese
- Experimental results show that our proposed M6 outperforms the baseline in a number of downstream tasks concerning both single modality and multiple modalities We will continue the pretraining of extremely large models by increasing data to explore the limit of its performance
Upload PDF to Generate Summary
Must-Reading Tree
Example
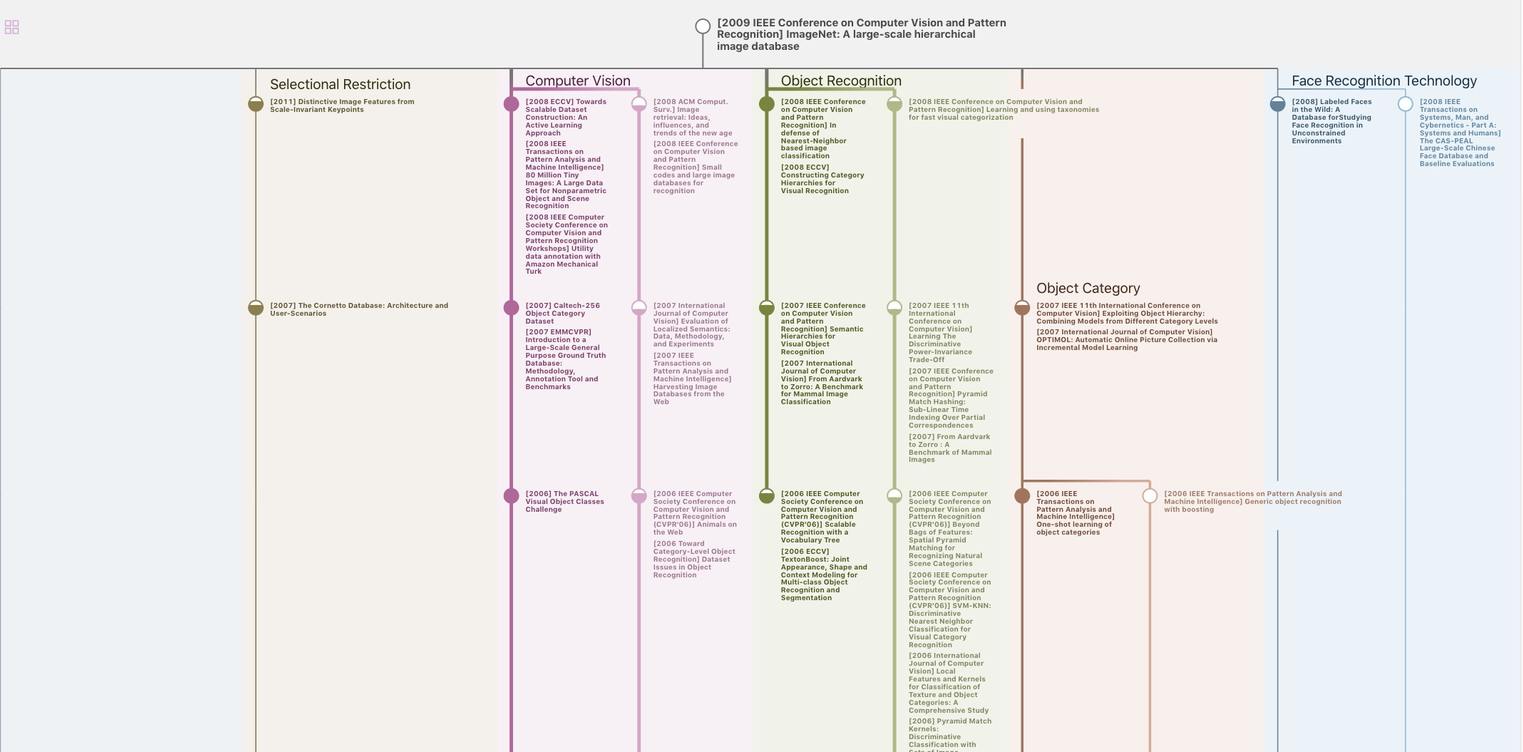
Generate MRT to find the research sequence of this paper
Data Disclaimer
The page data are from open Internet sources, cooperative publishers and automatic analysis results through AI technology. We do not make any commitments and guarantees for the validity, accuracy, correctness, reliability, completeness and timeliness of the page data. If you have any questions, please contact us by email: report@aminer.cn
Chat Paper