Fuzzy Clustered Federated Learning under Mixed Data Distributions.
AAMAS '24 Proceedings of the 23rd International Conference on Autonomous Agents and Multiagent Systems(2024)
摘要
Federated learning (FL) is deeply troubled by non-independent and identically distributed (non-IID) data, leading to suboptimal training results. Clustered FL partitions clients' unique data into different clusters to reduce the heterogeneity among clients. Current approaches are unable to eliminate the impact of data heterogeneity and provide personalized models to client devices. By assuming the clients' data can be divided into different data distributions, we propose a novel fuzzy clustered FL method. We partition the client's data and generate a personalized model for each client. The experiments demonstrated that our method achieved excellent results. In the case of N clusters, our method achieved a communication cost reduction of 1/N compared to the SOTA methods, while improving performance by 10.4% on CIFAR-10.
更多查看译文
AI 理解论文
溯源树
样例
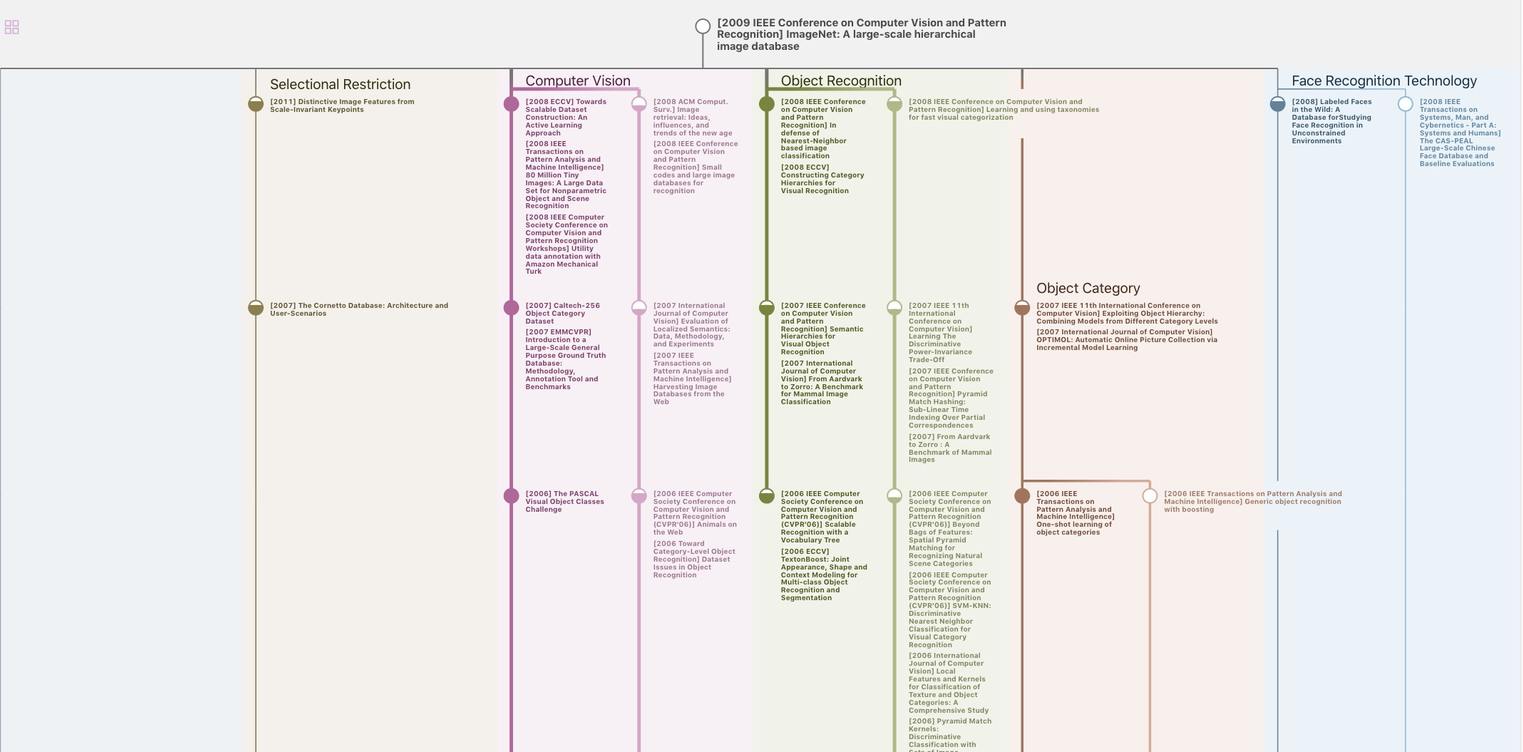
生成溯源树,研究论文发展脉络
Chat Paper
正在生成论文摘要