A novel stochastic configuration network with enhanced feature extraction for industrial process modeling
Neurocomputing(2024)
摘要
Stochastic configuration networks (SCNs), possessing sound generalization performance and low computational burden, have been extensively investigated in data analysis field. But in practice, it performs poor. This is mainly caused by two aspects: (1) the increment of random hidden nodes with incompact constraints may bring the emergence of redundant nodes, thereby resulting in poor modeling performance, and (2) SCNs have limited feature extraction capabilities. To address these issues, a novel SCN inserting feature layer, termed as FSCN, is presented in this paper. First, the embedded feature layer establishes a connection between the input and hidden layers, thus mapping the input data into a suitable feature space. Second, an inequality constraint based on Greville iterative method is established. It can not only facilitate the construction of high-quality hidden nodes but also guarantee convergence of the built model. Then, the Greville iterative method is also employed for output weight updating. Finally, comparative experiments on a real-valued nonlinear function, four real-world data sets and the modeling of two real industrial processes are conducted and the comparison results with other modeling methods show that FSCN has superior performance in terms of both model accuracy and compactness.
更多查看译文
关键词
Stochastic configuration networks,Feature extraction,Greville iterative method,Inequality constraint
AI 理解论文
溯源树
样例
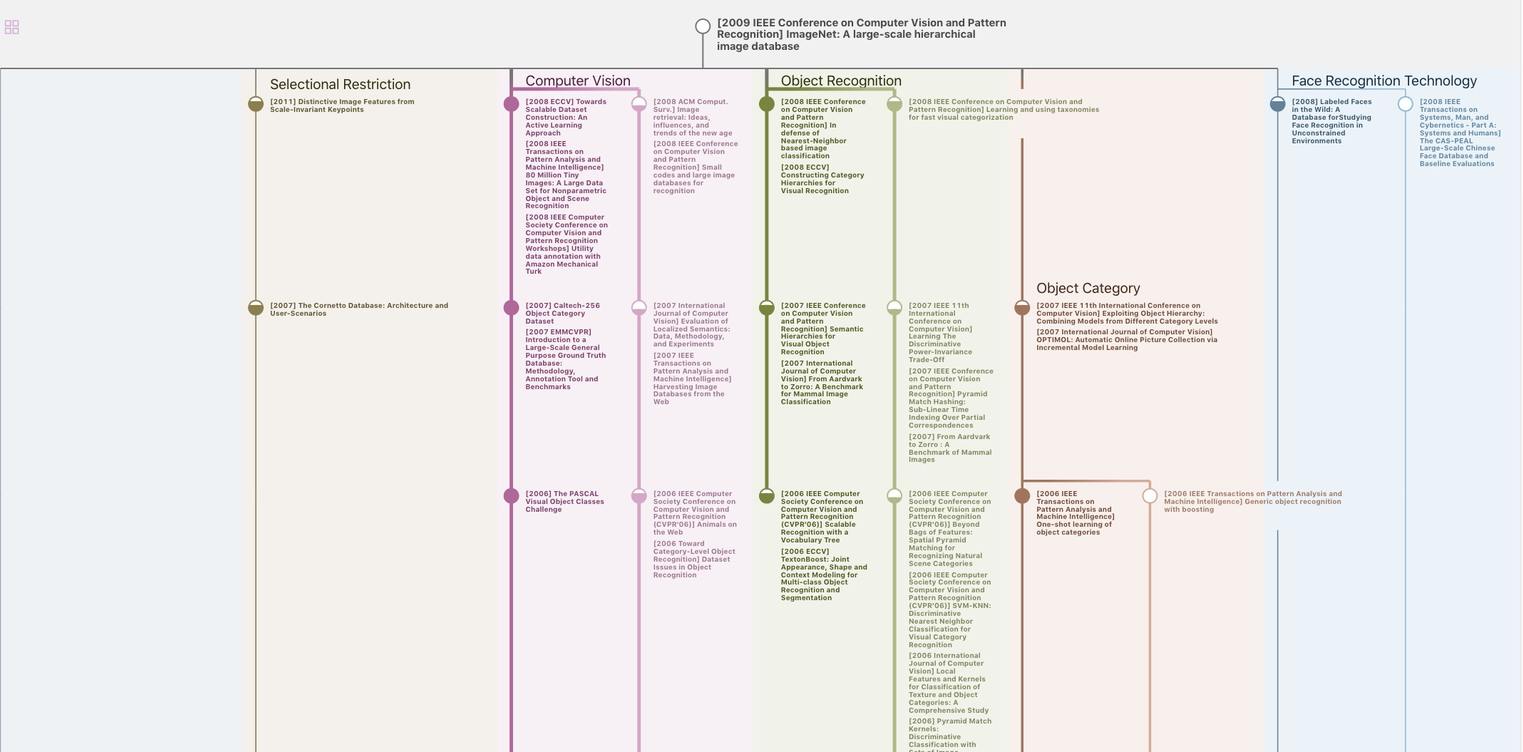
生成溯源树,研究论文发展脉络
Chat Paper
正在生成论文摘要