Recruitment strategies for reaching adults aged 50 years and older with low socioeconomic status for participation in online physical activity interventions.
Frontiers in digital health(2024)
摘要
Background:Generally, the health condition of those with higher socioeconomic status (SES) is better compared to those with lower SES. The application of appropriate strategies to reach low SES populations with electronic health (eHealth) interventions is thus of major importance to reduce health inequalities. eHealth-studies providing detailed information on recruitment strategies are scarce, despite the fact that this information is crucial for comparable research and implementation.
Objective:To provide insight into the reach, sample characteristics and costs of three pre-planned strategies for recruiting adults aged 50 years and older with low SES for participation in an online physical activity intervention, as part of a field study.
Methods:Recruitment took place via (1) invitation letters via a municipality, (2) gyms and (3) social media advertisements, aiming to include 400 participants. Additional procedures were followed to reach specifically the low SES group. Response rates, sociodemographic characteristics and costs per strategy were assessed.
Results:The highest response was shown for the municipality approach (N = 281), followed by social media (N = 71) and gyms (N = 45). Ten participants were recruited via family/friends. The most low-educated participants were reached via the municipality (N = 128) followed by social media (N = 9), gyms (N = 8) and family/friends (N = 5). Recruitment costs were with €2,142.37 the highest for the municipality compared to €96.81 for social media and no costs for gyms.
Conclusions:Recruitment via invitation letters through a municipality has the highest potential for reaching low SES participants of the three applied strategies, although the higher recruitment costs need to be taken into account.
更多查看译文
关键词
older adults,low education,vulnerable populations,eHealth,mHealth
AI 理解论文
溯源树
样例
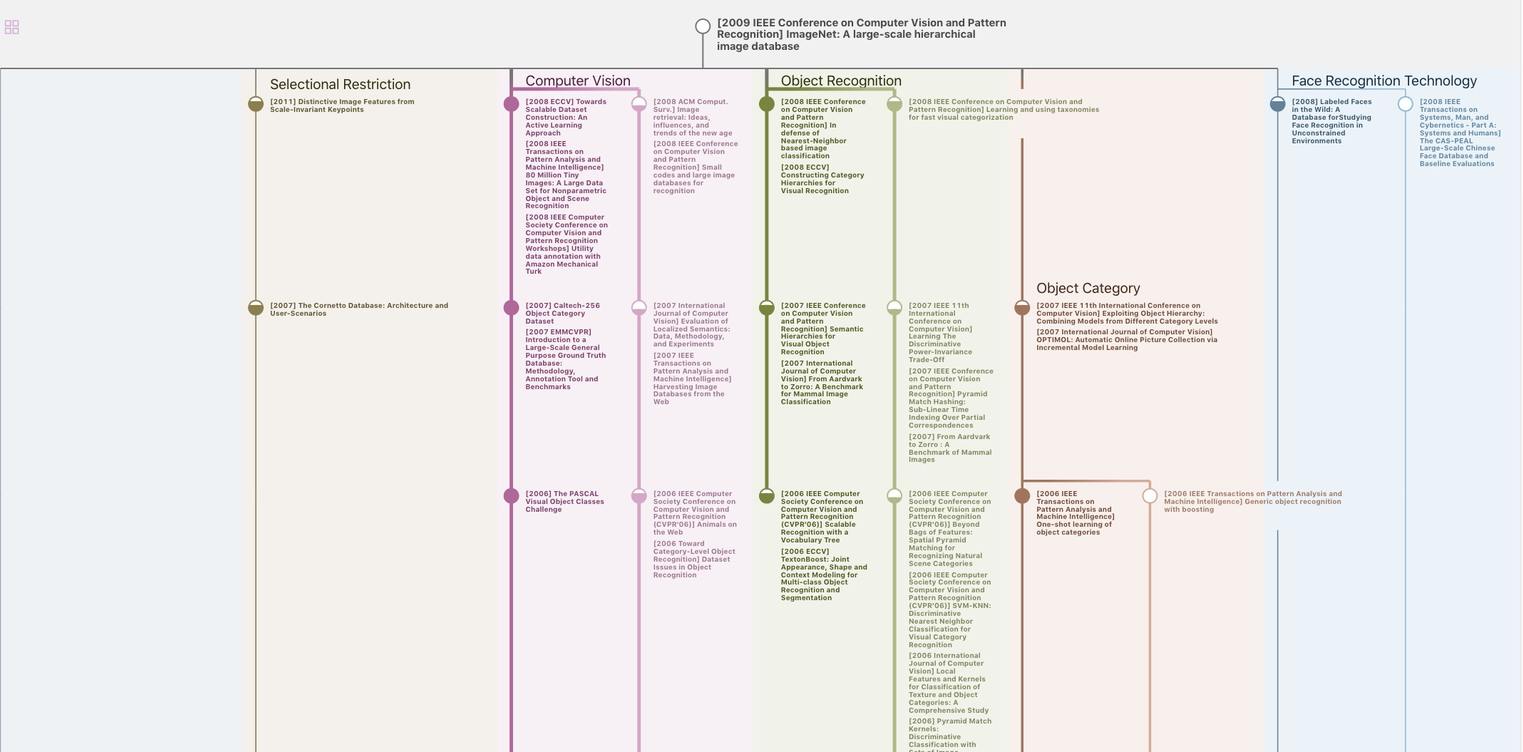
生成溯源树,研究论文发展脉络
Chat Paper
正在生成论文摘要