BathNet: A network to classification of fundus and contrast images using label transfer and multi-branch transformer
Biomedical Signal Processing and Control(2024)
摘要
Diabetic retinopathy (DRP) is commonly caused by the complications of diabetes and, if ignored and untreated, may lead to irreversible blindness in adults. Medical experts mainly diagnose DRP by its disease features, such as microangioma (MA), exudate (EX), and hemorrhage (HE). However, there are limitations in the diagnostic effect due to the small disease features lesions and inconspicuous feature areas of DRP. In this paper, we propose a self-calibrated convolutional network, BathNet, for fine-grained classification, to assist in the diagnosis of three disease features, MA, EX, and HE. In this network we replace the basic convolutional blocks with self-calibrated convolutional blocks so that the internal communication can be extended the field of view of each convolutional layer to extract feature information efficiently. Then, in the multi-branch structure, we obtain local features by using the CNN structure with self-calibrated convolution blocks, and global features by using the progressive transformer structure to obtain the remote dependencies of the feature mapping. Finally, the relatively important feature channels are filtered out by the branch fusion module. In addition, many DRP-related disease features (e.g., EX) must be observed by the fluorescein angiography fundus image (FAF) but not by the colorful fundus image (CFP), so we propose a method for label migration across modalities by corresponding feature labels on FAF images to CFP images as network inputs. The experiment showed that the prediction results of CFP images after label migration were similar to FAF images. Comparing with baseline, our study BathNet achieves the best accuracy for MA, EX, and HE disease features, from 57.01%, 58.88%, and 64.49% to 78.5%, 71.96%, and 77.57%, respectively. In the future, patients can diagnose the disease features on FAF images with only one CFP image, which largely reduces the cost of disease detection. The code is available at https://github.com/hedgehogW/BathNet/.
更多查看译文
关键词
Diabetic retinopathy,Self-calibrated convolutional,Label transfer,Multi-branch structure,Colorful fundus image,Fluorescein angiography fundus image
AI 理解论文
溯源树
样例
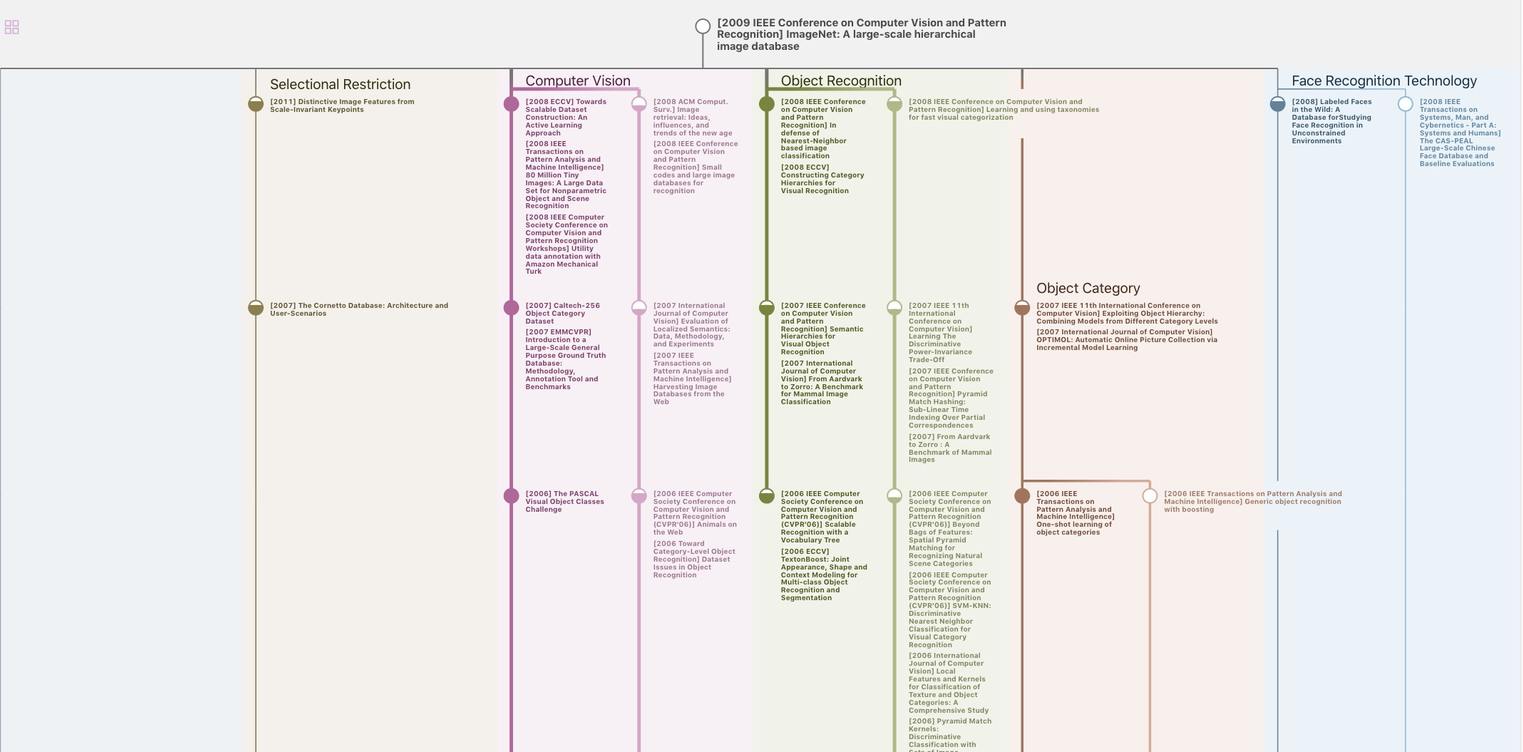
生成溯源树,研究论文发展脉络
Chat Paper
正在生成论文摘要