An Evaluation of Statistical and Deep Learning-Based Correction of Monthly Precipitation over the Yangtze River Basin in China Based on CMIP6 GCMs
Environment, development and sustainability(2024)
摘要
Precipitation, as one of the most indispensable and significant material resources for human survival and society development. Compared with observed data, numerical simulation precipitation products, exemplified by Coupled Model Intercomparison Project Phase 6 (CMIP6), provide a better way to understand past, present, and future climate changes, and have been widely used in hydrological, ecological, and environmental fields. However, the precipitation data of CMIP6 often contains a high degree of uncertainty and bias. This study corrected the historical monthly precipitation data over the Yangtze River Basin (YRB) based on four Global climate models (GCMs) from the CMIP6, by using one statistic method, principal component analysis (PCA), and two deep learning methods, deep neural network (DNN) method, and the long short-term memory (LSTM) based method. The revised monthly precipitation was analyzed temporally and spatially, and the results showed that the LSTM-based method is the most appropriate and gained better performance of correlation, root mean square error (RMSE) and relative bias(RBIAS). Then the precipitation of future scenes was also corrected by the LSTM-based method, the shortcoming of original CMIP6 data in overestimating rainfall has been significantly improved by reducing the peak of extreme rainfall. This result helps us to get a better understanding of the status and trends of rainfall data. Besides, this study not only takes advantage of the fact that LSTM is inherently suitable for long time-series data, but also takes into account spatial heterogeneity by dividing the study area into grids and the data in each grid were revised individually. Therefore, the method is flexible and can be widely used in other regions.
更多查看译文
关键词
CMIP6,YRB,LSTM,Precipitation,Correction method
AI 理解论文
溯源树
样例
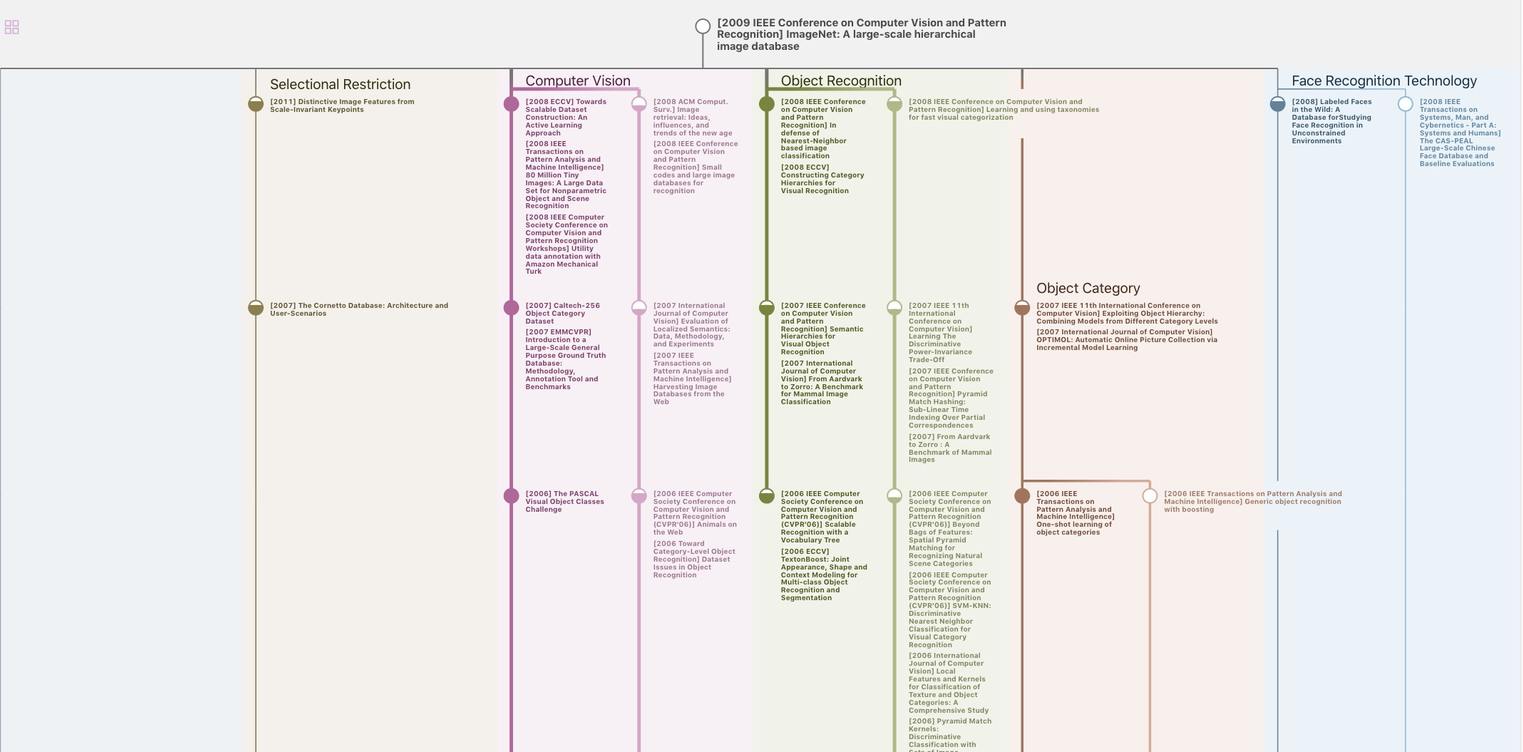
生成溯源树,研究论文发展脉络
Chat Paper
正在生成论文摘要