Estimation Aboveground Biomass in Subtropical Bamboo Forests Based on an Interpretable Machine Learning Framework
Environmental modelling & software(2024)
摘要
Forest biomass is an essential indicator of forest ecosystem carbon cycle and global climate change research, and traditional machine learning cannot explain the mechanism of feature variable impact on forest aboveground biomass (AGB). Therefore, we proposed an interpretable bamboo forest AGB prediction method based on Shaply Additive exPlanation (SHAP) and XGBoost model to explain the impact mechanism of feature variables on AGB. The bamboo forest AGB is estimated using the monthly and annual scale leaf area index (LAI), enhanced vegetation index (EVI), ratio vegetation index (RVI), precipitation (Pre), maximum temperature (Tmax), minimum temperature (Tmin) and solar radiation (Rad) data. The results showed that the method could be effectively predict AGB, and precipitation more important than temperature. The framework revealed the threshold effect, exceeded the threshold value, the impacts of LAI_Ann, EVI_Ann, and Pre_11 on AGB were stable. The SHAP interaction value between LAI_Ann and EVI_Ann decreased with increasing EVI_Ann and LAI_Ann. By contrast, when Pre_11 increased, the SHAP interaction value between LAI_Ann and Pre_11 increased with increasing LAI_Ann. The framework could also be easily implemented, providing an interpretable machine learning model of forest AGB.
更多查看译文
关键词
Bamboo forest,AGB estimation,Interpretable machine learning,SHAP value
AI 理解论文
溯源树
样例
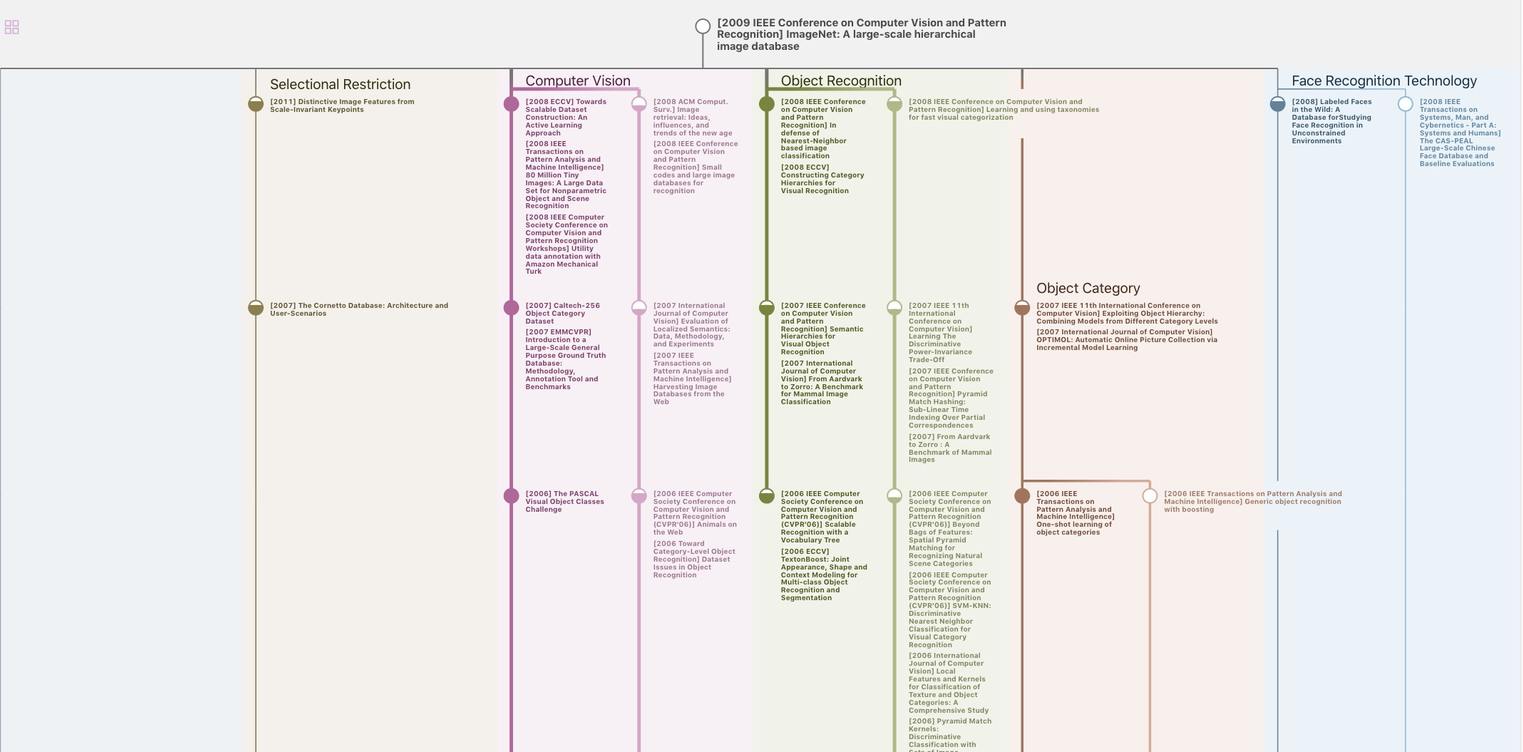
生成溯源树,研究论文发展脉络
Chat Paper
正在生成论文摘要