MA-BLTSI Model for Land Surface Temperature Prediction Based on Multi-Dimensional Data
Theoretical and applied climatology(2024)
摘要
Temperature prediction is a challenging problem due to various complex factors, including geographical location, terrain, seasonal changes, climate and weather conditions, as well as human activities. To increase the precision of temperature prediction, this paper proposes a novel combined attention model (MA-BLTSI) utilizing time series decomposition theory to predict daily average temperature in Chinese cities over the next 30 days. Firstly, features related to temperature are selected from a larger set of potential features by calculating the Pearson correlation coefficient. Then, the temperature feature and the selected features together are decomposed using a moving average (MA) to obtain the trend, seasonality and residual components. Next, the features of each component are combined with the bidirectional long short-term memory (Bi-LSTM), trend decomposition transformer (TDformer) Seasonal, and Informer models to obtain the prediction results for each component. Finally, the prediction of the temperature for the next 30 days is obtained by aggregating the predictions of each component using a specific method. The proposed method is validated using datasets from six Chinese cities: Beijing, Shenyang, Shanghai, Guangzhou, Chengdu, and Xi’an. The results obtained demonstrate lower average mean squared error (MSE) and average absolute error (MAE) values compared to those of the Bi-LSTM, TDformer, and Informer models. The achieved results for Beijing are (0.070 and 0.210), Shenyang (0.092 and 0.240), Shanghai (0.113 and 0.264), Guangzhou (0.245 and 0.386), Chengdu (0.153 and 0.315), and Xi’an (0.113 and 0.269). The results indicate that the combined model outperforms the individual models in terms of temperature prediction.
更多查看译文
AI 理解论文
溯源树
样例
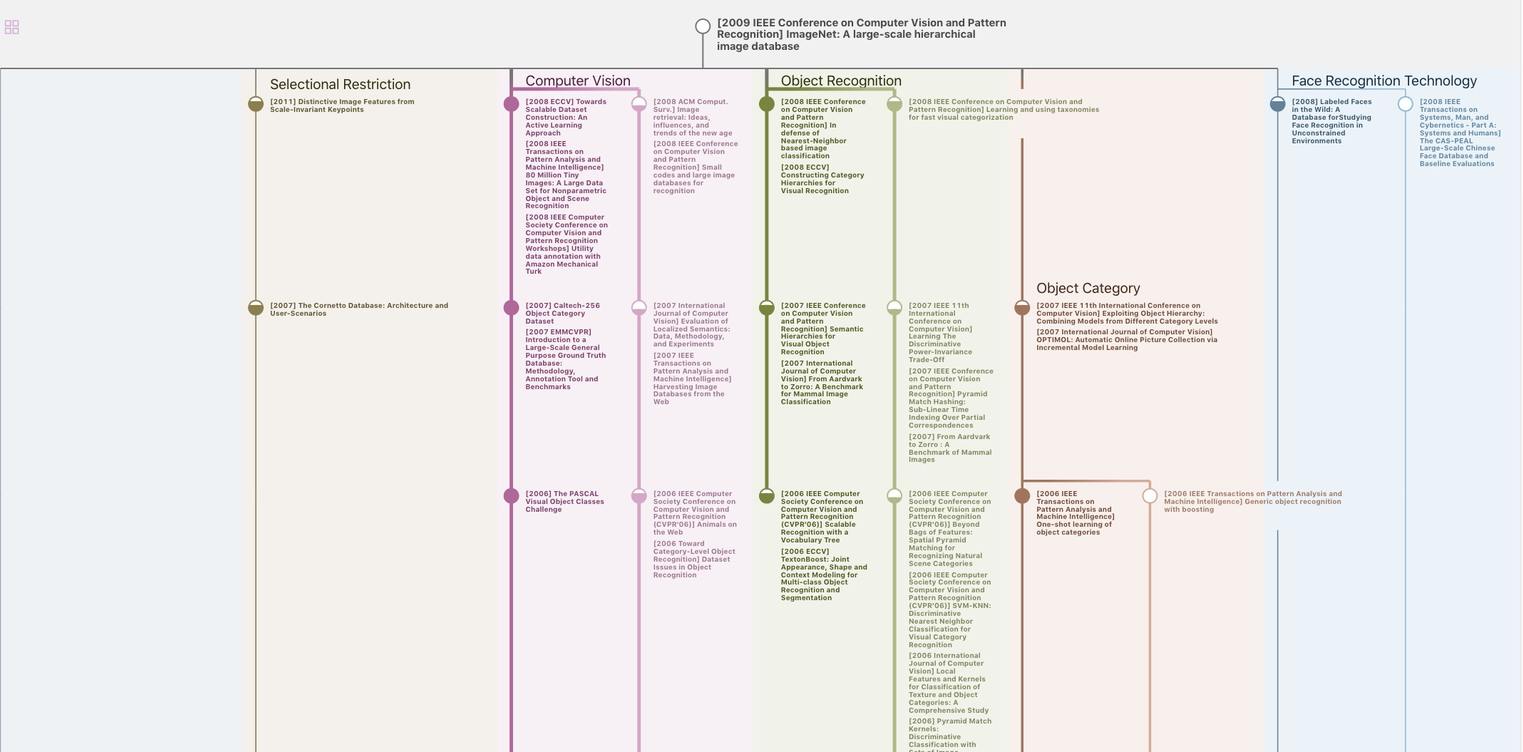
生成溯源树,研究论文发展脉络
Chat Paper
正在生成论文摘要