SFL-TUM: Energy Efficient SFRL method for Large Scale AI Model's Task Offloading in UAV-Assisted MEC Networks
Vehicular Communications(2024)
摘要
The convergence of mobile edge computing (MEC) network with unmanned aerial vehicles (UAVs) presents an auspicious opportunity to revolutionize wireless communication and facilitate high-speed internet access in remote regions for mobile devices (MDs) as well as large scale artificial intelligence (AI) models. However, the substantial amount of data produced by the UAVs-assisted MEC network necessitates the integration of efficient distributed learning techniques in AI models. In recent times, distributed learning algorithms, including federated reinforcement learning (FRL) and split learning (SL), have been explored for the purpose of learning machine learning (ML) models that are distributed by sharing model parameters, as opposed to large raw data-sets as seen in traditional centralized learning algorithms. To implement the hybrid method, the model is first trained locally on each UAV-assisted MEC network using SL. Subsequently, the model parameters that have been encrypted are sent to a central server for federated averaging. Finally, after the model has been updated, it is distributed to each UAV-assisted MEC network for local fine-tuning. Our simulations indicate that the proposed split and federated reinforcement learning (SFRL) framework yields comparable high-test accuracy performance while consuming less energy compared to extant distributed learning algorithms. Furthermore, the SFRL algorithm efficiently realizes energy-efficient selection between the SL and FRL methods under different distributions. Numerical results shows that the proposed scheme improves the accuracy by 29.31% and reduced the energy consumption by around 67.34% and time delay by about 7.37%. as compared to the existing baseline schemes.
更多查看译文
关键词
Mobile Edge Computing,Unmanned Aerial Vehicle,Task Offloading,Federated Reinforcement Learning,Split Learning
AI 理解论文
溯源树
样例
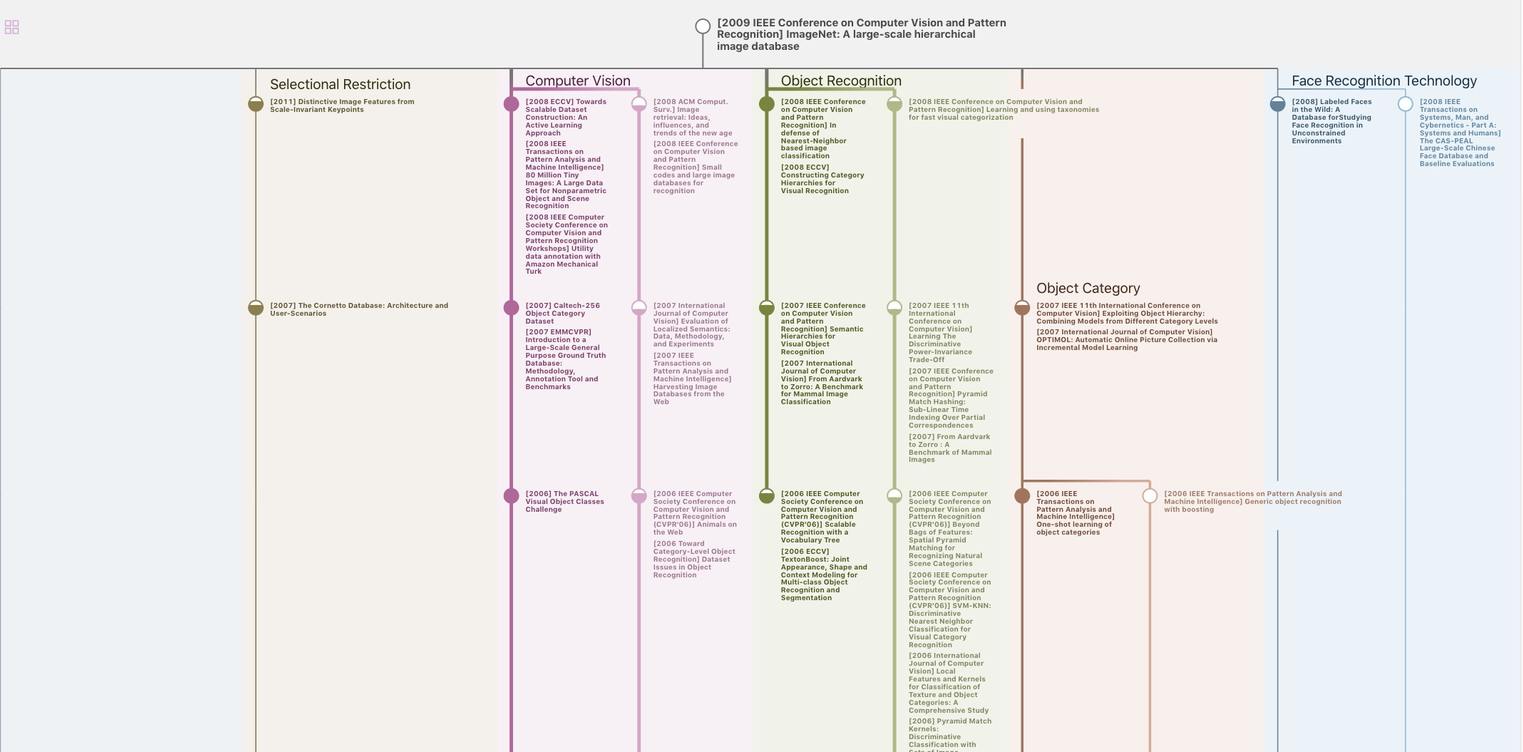
生成溯源树,研究论文发展脉络
Chat Paper
正在生成论文摘要