Global-Local Feature Learning Via Dynamic Spatial-Temporal Graph Neural Network in Meteorological Prediction
IEEE Transactions on Knowledge and Data Engineering(2024)
摘要
The meteorological environment has a profound impact on global health (e.g., air quality), science and technology (e.g., rocket launches), and economic development (e.g., poverty reduction) etc. Meteorological prediction presents numerous challenges to both academia and industry due to its multifaceted nature which encompasses real-time observations and complex modeling. Recent research adopt graph convolutional recurrent network and establish coordinate information to obtain local spatial-temporal pattern. However, the model only utilizes the local spatial-temporal information and fail to fully consider the dynamic meteorological situation. To address the above limitations, we propose a Dynamic Spatial-Temporal Graph Neural Network (DSTGNN) to learn global-local meteorological features. Specifically, we divide the global spatial-temporal information along the timeline to obtain local spatial-temporal information. For the global aspect, we design a random throwedge module during the neighborhood propagation process in graph neural network (GNN) to extract the features and adapt to the dynamic situation. We also establish convolution operation module to learn the features. Next, we perform information fusion on the two modules to capture sufficient features. In addition, we employ graph ordinary differential equation (ODE) network and utilize the coordinate information to obtain the long-term features and coordinate relationships. In the local aspect, we first construct a GNN to conduct graph embedding. Then, we integrate another GNN into a gated recurrent unit (GRU) and also use the coordinate information to explore the features and coordinate relationships. Finally, we combine the global and local features via a global-local features learning layer for meteorological prediction. Experimental results on the four real-world meteorological datasets show that DSTGNN outperforms the baseline models.
更多查看译文
关键词
Dynamic spatial-temporal graph neural network,global-local meteorological features,graph ODE network,meteorological prediction,random throw edge
AI 理解论文
溯源树
样例
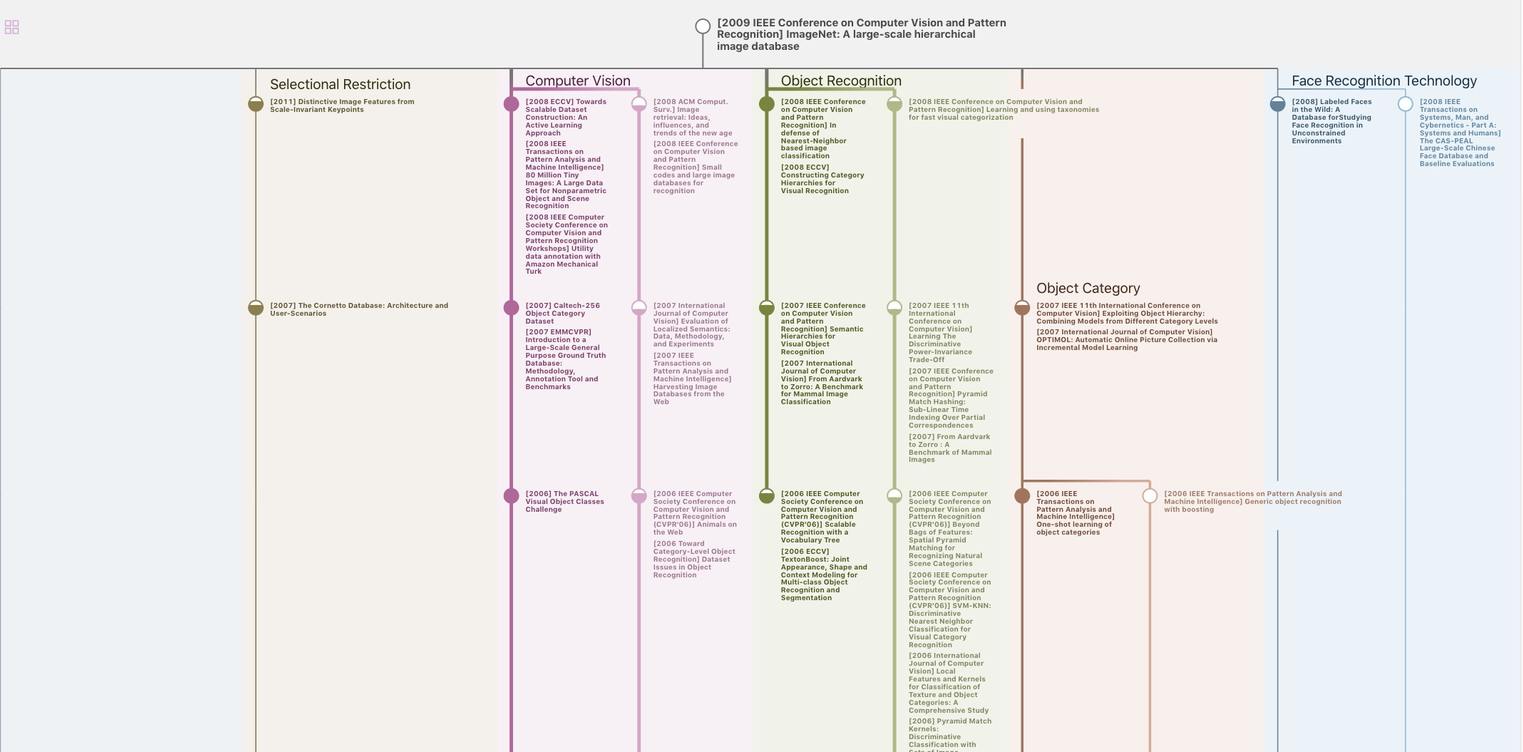
生成溯源树,研究论文发展脉络
Chat Paper
正在生成论文摘要