Evaluating the performance of metaheuristic-tuned weight agnostic neural networks for crop yield prediction
Neural Computing and Applications(2024)
摘要
This study explores crop yield forecasting through weight agnostic neural networks (WANN) optimized by a modified metaheuristic. WANNs offer the potential for lighter networks with shared weights, utilizing a two-layer cooperative framework to optimize network architecture and shared weights. The proposed metaheuristic is tested on real-world crop datasets and benchmarked against state-of-the-art algorithms using standard regression metrics. While not claiming WANN as the definitive solution, the model demonstrates significant potential in crop forecasting with lightweight architectures. The optimized WANN models achieve a mean absolute error (MAE) of 0.017698 and an R-squared ( R^2 ) score of 0.886555, indicating promising forecasting performance. Statistical analysis and Simulator for Autonomy and Generality Evaluation (SAGE) validate the improvement significance and feature importance of the proposed approach.
更多查看译文
关键词
Crop yield prediction,Reptile search algorithm,Weight agnostic neural networks,Metaheuristics
AI 理解论文
溯源树
样例
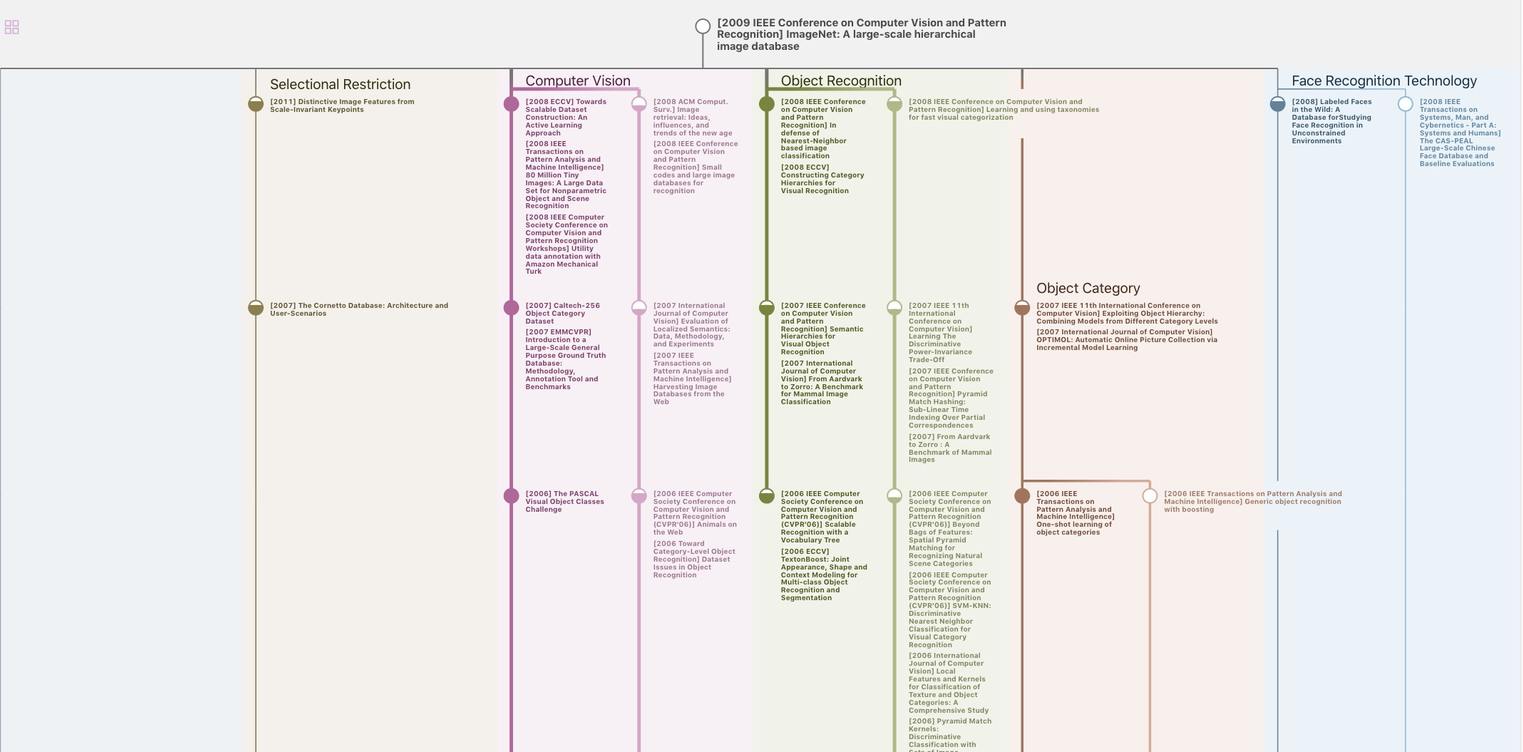
生成溯源树,研究论文发展脉络
Chat Paper
正在生成论文摘要