Machine Learning Quantification of Pulmonary Regurgitation Fraction from Echocardiography
Pediatric Cardiology(2024)
摘要
Assessment of pulmonary regurgitation (PR) guides treatment for patients with congenital heart disease. Quantitative assessment of PR fraction (PRF) by echocardiography is limited. Cardiac MRI (cMRI) is the reference-standard for PRF quantification. We created an algorithm to predict cMRI-quantified PRF from echocardiography using machine learning (ML). We retrospectively performed echocardiographic measurements paired to cMRI within 3 months in patients with ≥ mild PR from 2009 to 2022. Model inputs were vena contracta ratio, PR index, PR pressure half-time, main and branch pulmonary artery diastolic flow reversal (BPAFR), and transannular patch repair. A gradient boosted trees ML algorithm was trained using k-fold cross-validation to predict cMRI PRF by phase contrast imaging as a continuous number and at > mild (PRF ≥ 20
更多查看译文
关键词
Machine learning,XGBoost,Pulmonary regurgitation,Echocardiography,Cardiac magnetic resonance imaging,Model validation,Congenital heart disease,Tetralogy of Fallot
AI 理解论文
溯源树
样例
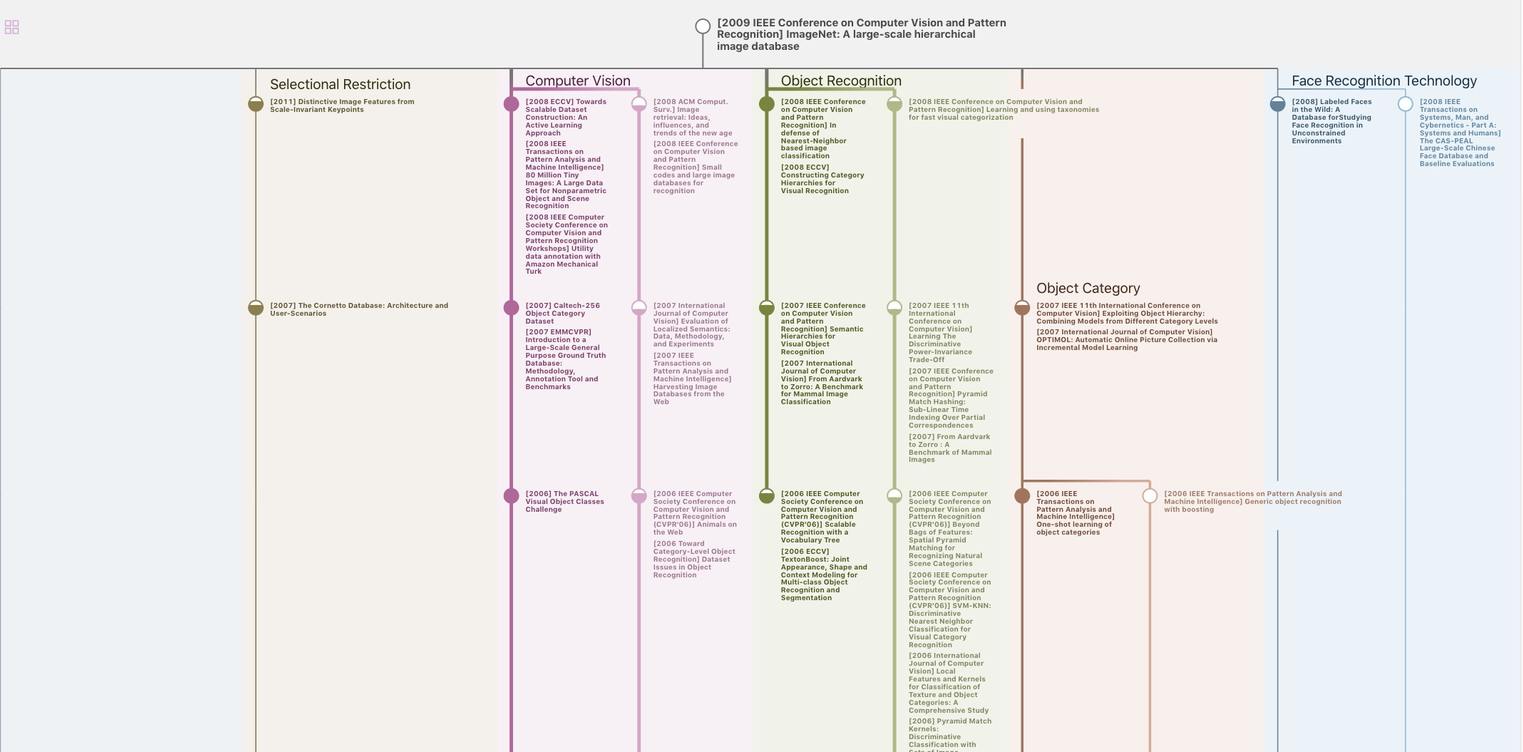
生成溯源树,研究论文发展脉络
Chat Paper
正在生成论文摘要