Patient-Representing Population Perceptions of GPT-Generated vs. Standard Emergency Department Discharge Instructions: Randomized Blind Survey Assessment (Preprint)
Journal of Medical Internet Research(2024)
摘要
BACKGROUND Discharge instructions are a key form of documentation and patient communication in the time of transition from the Emergency Department (ED) to home. Discharge instructions are time-consuming and often under-prioritized, especially in the ED, leading to discharge delays and patient instructions that are either impersonal. Generative artificial intelligence and large language models (LLMs) offer promising methods of creating high-quality and personalized discharge instructions, however there exists a gap in understanding patient perspectives of LLM-generated discharge instructions. OBJECTIVE We aimed to assess the use of LLMs such as ChatGPT in synthesizing accurate and patient-accessible discharge instructions from the ED. METHODS We synthesized 5 unique, fictional ED encounters meant to emulate real ED encounters that included a diverse set of clinician H&P notes and nursing notes. These were passed to GPT-4 in Azure OpenAI service to generate corresponding LLM-generated discharge instructions. Standard discharge instructions were also generated for each of the 5 unique ED encounters. All GPT-generated and standard discharge instructions were then formatted into standardized after-visit summary documents. These after-visit summaries containing either GPT-generated discharge instructions or standard discharge instructions were given to Amazon MTurk respondents subjects representing patient populations through Amazon MTurk Survey Distribution. Discharge instructions were assessed based upon metrics of interpretability of significance, understandability, and satisfaction. RESULTS Our findings revealed 155 survey respondents assigned favorable ratings more frequently to GPT-generated discharge instructions along the metrics of interpretability of significance in discharge instruction subsections regarding diagnosis, procedures, treatment, post-ED medications or any changes to medications, and return precautions (GPT/Standard respectively: 89.2%/79.5%, 86.7%/65.8%, 74.7%/61.6%, 63.9%/49.3%, 86.7%/68.5%). Survey Respondents found GPT-generated instructions more understandable when rating procedures, treatment, post-ED medications or medication changes, post-ED follow-up, and return precautions (80.7%/61.6%, 85.5%/68.5%, 68.7%/57.5%, 86.7%/76.7%, 85.5%/76.7%). Satisfaction with GPT-generated discharge instruction subsections were most favorable in procedures, treatment, post-ED medications or medication changes, and return precautions (75.9%/54.8%, 85.5%/68.5%, 62.7%/53.4%, 83.1%/71.2%). Kruskal-Wallis analysis of Likert-responses between GPT-generated and standard discharge instructions did not conclude significant differences within any specific metric and discharge instruction subsection. CONCLUSIONS This study demonstrates the potential for LLMs such as ChatGPT to act as a method of augmenting current documentation workflows in the ED to reduce documentation burden of physicians. The ability for LLMs to provide tailored instructions for patients by improving readability and making instructions more applicable to patients could possibly improve upon the methods of communication that currently exist.
更多查看译文
AI 理解论文
溯源树
样例
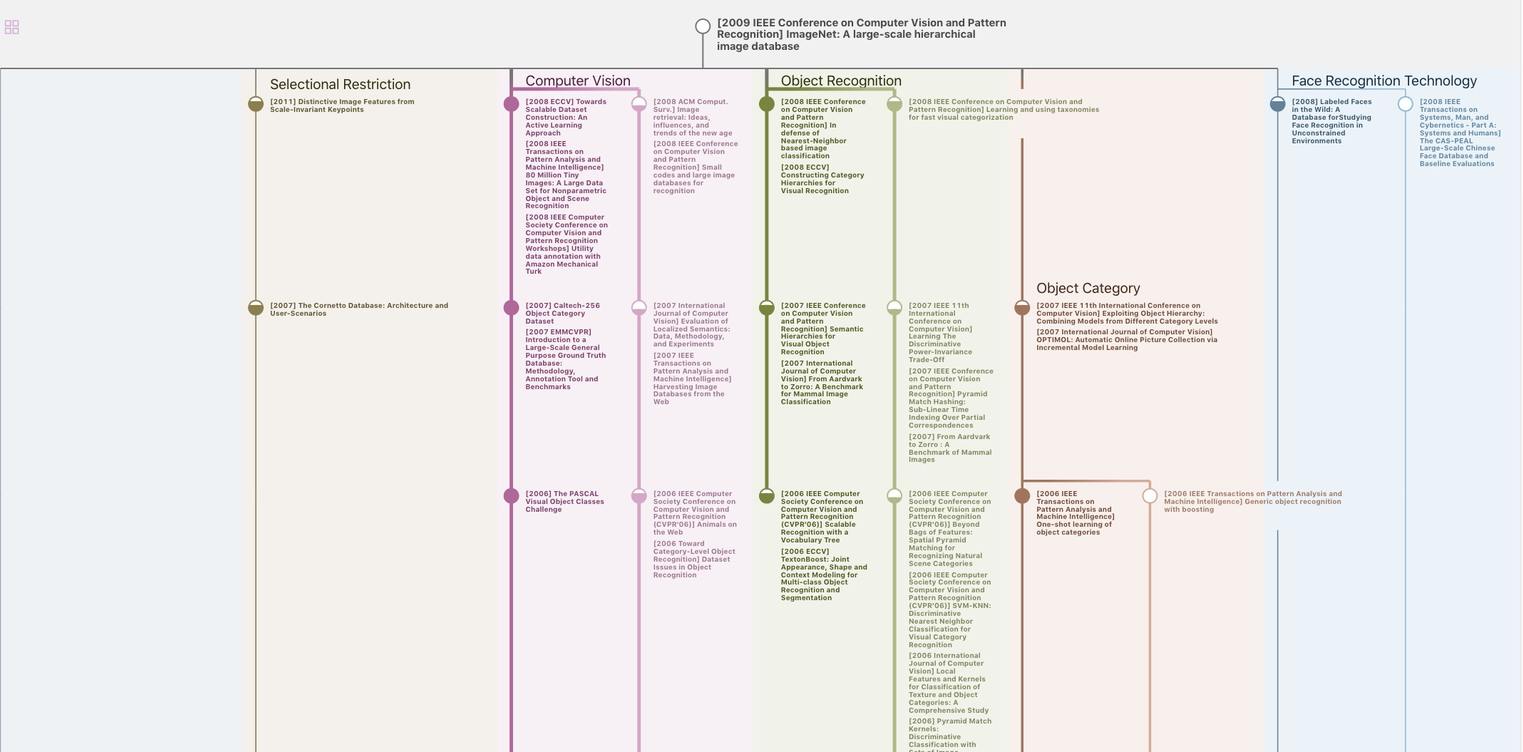
生成溯源树,研究论文发展脉络
Chat Paper
正在生成论文摘要