Time-Aware Preference Recommendation Based on Behavior Sequence.
International Joint Conference on Web and Big Data(2023)
摘要
Sequential recommendation (SR) has become an important schema to assist people in rapidly finding their interest in the progressively growing data. Especially, long and short-term based methods capture user preferences and provide more precise recommendations. However, they rarely consider the effect of time intervals and limit the short-term preferences’ weight in predicting the next items. In this paper, we propose a novel model called TPR-BS ( T ime-aware P reference R ecommendation based on B ehavior S equence) to address these issues. We model the user’s long and short-term behavioral sequence separately and fuse sequence features to obtain the user’s comprehensive preferences’ representation. Specifically, we first use the sparse attention layer to filter the effect of irrelevant information on long-term preferences. Then we modify the Gated Recurrent Unit (GRU) based on time intervals and encode the user’s short-term behavior sequence into the hidden states for the corresponding moment. Besides, we construct a target attention network layer to highlight the last-moment interaction behavior. TPR-BS aims to dynamically capture user preferences’ changes which can reflect the user’s general preferences and the latest intentions. The experimental results indicate that our model outperforms state-of-the-art methods on three public datasets.
更多查看译文
AI 理解论文
溯源树
样例
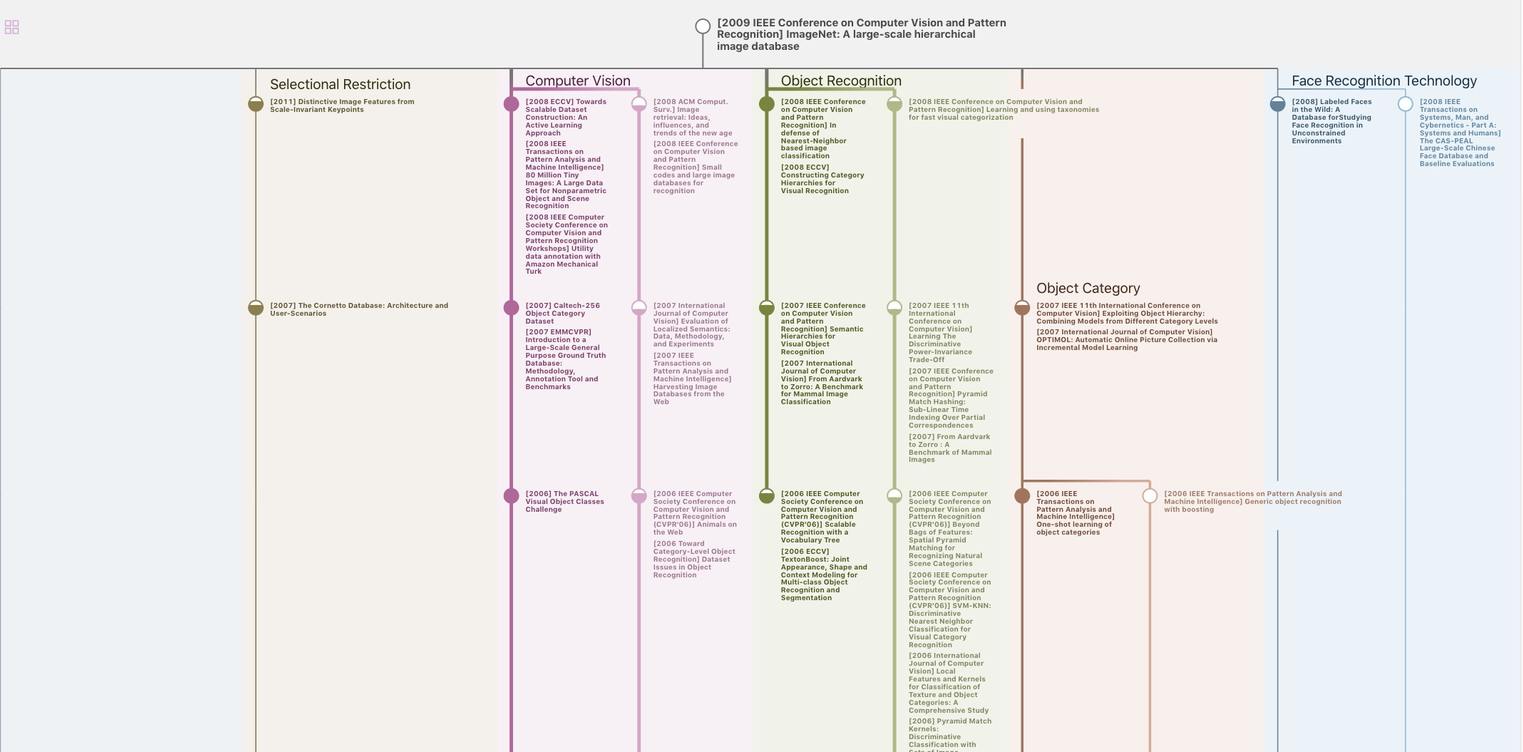
生成溯源树,研究论文发展脉络
Chat Paper
正在生成论文摘要